Lookup table with 'wildcards' in PandasAdd one row to pandas DataFrameSelecting multiple columns in a pandas dataframeRenaming columns in pandasAdding new column to existing DataFrame in Python pandasDelete column from pandas DataFrame by column name“Large data” work flows using pandasHow to iterate over rows in a DataFrame in Pandas?Select rows from a DataFrame based on values in a column in pandasGet list from pandas DataFrame column headersHow to convert timezone to country code in Python?
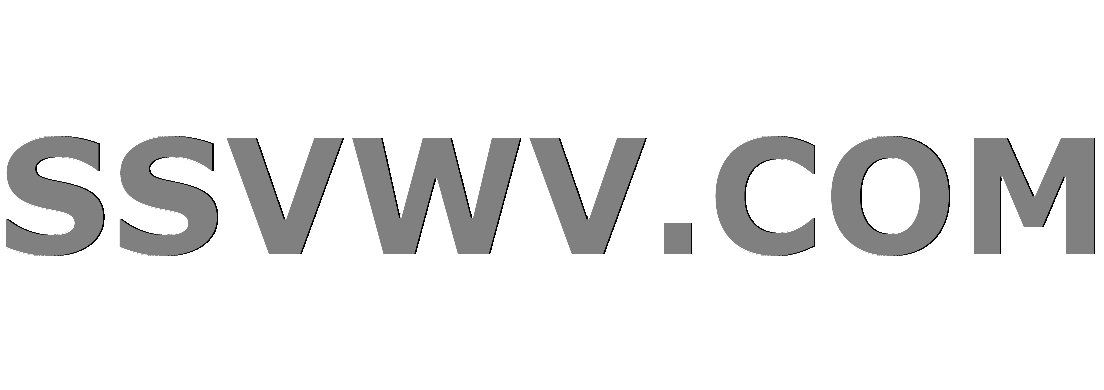
Multi tool use
Cursor Replacement for Newbies
How much of data wrangling is a data scientist's job?
Can my sorcerer use a spellbook only to collect spells and scribe scrolls, not cast?
Why do bosons tend to occupy the same state?
How dangerous is XSS?
Is it acceptable for a professor to tell male students to not think that they are smarter than female students?
I would say: "You are another teacher", but she is a woman and I am a man
How would I stat a creature to be immune to everything but the Magic Missile spell? (just for fun)
Watching something be piped to a file live with tail
Is the myth that if you can play one instrument, you can learn another instrument with ease true?
Why is this clock signal connected to a capacitor to gnd?
Am I breaking OOP practice with this architecture?
Can I run a new neutral wire to repair a broken circuit?
How do I deal with an unproductive colleague in a small company?
How can I determine if the org that I'm currently connected to is a scratch org?
Can a virus destroy the BIOS of a modern computer?
What is the most common color to indicate the input-field is disabled?
What reasons are there for a Capitalist to oppose a 100% inheritance tax?
What are some good books on Machine Learning and AI like Krugman, Wells and Graddy's "Essentials of Economics"
How can saying a song's name be a copyright violation?
Do scales need to be in alphabetical order?
CAST throwing error when run in stored procedure but not when run as raw query
Short story with a alien planet, government officials must wear exploding medallions
Mathematica command that allows it to read my intentions
Lookup table with 'wildcards' in Pandas
Add one row to pandas DataFrameSelecting multiple columns in a pandas dataframeRenaming columns in pandasAdding new column to existing DataFrame in Python pandasDelete column from pandas DataFrame by column name“Large data” work flows using pandasHow to iterate over rows in a DataFrame in Pandas?Select rows from a DataFrame based on values in a column in pandasGet list from pandas DataFrame column headersHow to convert timezone to country code in Python?
I've been looking for an answer to this problem for a few days, but can't find anything similar in other threads.
I have a lookup table to define classification for some input data. The classification depends on continent, country and city. However, some classes may depend on a subset of these variables, e.g. only continent and country (no city). An example of such lookup table is below. In my example, I'm using one and two stars as wildcards:
- One Star: I want all cities in France to be classified as France, and
- Two Stars: All cities in US, excepting New York and San Francisco as USA - Other.
lookup_df = pd.DataFrame('Continent': ['Europe', 'Europe', 'Asia', 'America', 'America', 'America', 'America', 'Africa'],
'Country': ['France', 'Italy', 'Japan', 'USA', 'USA', 'USA', 'Argentina', '*'],
'City': ['*', '*', '*', 'New York', 'San Francisco', '**', '*', '*'],
'Classification': ['France', 'Italy', 'Japan', 'USA - NY', 'USA - SF', 'USA - Other', 'Argentina', 'Africa'])
If my dataframe is
df = pd.DataFrame('Continent': ['Europe', 'Europe', 'Asia', 'America ', 'America', 'America', 'Africa'],
'Country': ['France', 'Italy', 'Japan', 'USA', 'USA', 'USA', 'Egypt'],
'City': ['Paris', 'Rome', 'Tokyo', 'San Francisco', 'Houston', 'DC', 'Cairo'])
I am trying to get the following result:
Continent Country City Classification
0 Europe France Paris France
1 Europe Italy Rome Italy
2 Asia Japan Tokyo Japan
3 America USA San Francisco USA - SF
4 America USA Houston USA - Other
5 America USA DC USA - Other
6 Africa Egypt Cairo Africa
I need to start from a lookup table or similar because it's easier to maintain, easier to explain and it's also used by other processes. I can't create a full table, because I would have to consider all possible cities in the world.
Is there any pythonic way of doing this? I thought I could use pd.merge, but I haven't found any examples of this online.
python pandas
add a comment |
I've been looking for an answer to this problem for a few days, but can't find anything similar in other threads.
I have a lookup table to define classification for some input data. The classification depends on continent, country and city. However, some classes may depend on a subset of these variables, e.g. only continent and country (no city). An example of such lookup table is below. In my example, I'm using one and two stars as wildcards:
- One Star: I want all cities in France to be classified as France, and
- Two Stars: All cities in US, excepting New York and San Francisco as USA - Other.
lookup_df = pd.DataFrame('Continent': ['Europe', 'Europe', 'Asia', 'America', 'America', 'America', 'America', 'Africa'],
'Country': ['France', 'Italy', 'Japan', 'USA', 'USA', 'USA', 'Argentina', '*'],
'City': ['*', '*', '*', 'New York', 'San Francisco', '**', '*', '*'],
'Classification': ['France', 'Italy', 'Japan', 'USA - NY', 'USA - SF', 'USA - Other', 'Argentina', 'Africa'])
If my dataframe is
df = pd.DataFrame('Continent': ['Europe', 'Europe', 'Asia', 'America ', 'America', 'America', 'Africa'],
'Country': ['France', 'Italy', 'Japan', 'USA', 'USA', 'USA', 'Egypt'],
'City': ['Paris', 'Rome', 'Tokyo', 'San Francisco', 'Houston', 'DC', 'Cairo'])
I am trying to get the following result:
Continent Country City Classification
0 Europe France Paris France
1 Europe Italy Rome Italy
2 Asia Japan Tokyo Japan
3 America USA San Francisco USA - SF
4 America USA Houston USA - Other
5 America USA DC USA - Other
6 Africa Egypt Cairo Africa
I need to start from a lookup table or similar because it's easier to maintain, easier to explain and it's also used by other processes. I can't create a full table, because I would have to consider all possible cities in the world.
Is there any pythonic way of doing this? I thought I could use pd.merge, but I haven't found any examples of this online.
python pandas
add a comment |
I've been looking for an answer to this problem for a few days, but can't find anything similar in other threads.
I have a lookup table to define classification for some input data. The classification depends on continent, country and city. However, some classes may depend on a subset of these variables, e.g. only continent and country (no city). An example of such lookup table is below. In my example, I'm using one and two stars as wildcards:
- One Star: I want all cities in France to be classified as France, and
- Two Stars: All cities in US, excepting New York and San Francisco as USA - Other.
lookup_df = pd.DataFrame('Continent': ['Europe', 'Europe', 'Asia', 'America', 'America', 'America', 'America', 'Africa'],
'Country': ['France', 'Italy', 'Japan', 'USA', 'USA', 'USA', 'Argentina', '*'],
'City': ['*', '*', '*', 'New York', 'San Francisco', '**', '*', '*'],
'Classification': ['France', 'Italy', 'Japan', 'USA - NY', 'USA - SF', 'USA - Other', 'Argentina', 'Africa'])
If my dataframe is
df = pd.DataFrame('Continent': ['Europe', 'Europe', 'Asia', 'America ', 'America', 'America', 'Africa'],
'Country': ['France', 'Italy', 'Japan', 'USA', 'USA', 'USA', 'Egypt'],
'City': ['Paris', 'Rome', 'Tokyo', 'San Francisco', 'Houston', 'DC', 'Cairo'])
I am trying to get the following result:
Continent Country City Classification
0 Europe France Paris France
1 Europe Italy Rome Italy
2 Asia Japan Tokyo Japan
3 America USA San Francisco USA - SF
4 America USA Houston USA - Other
5 America USA DC USA - Other
6 Africa Egypt Cairo Africa
I need to start from a lookup table or similar because it's easier to maintain, easier to explain and it's also used by other processes. I can't create a full table, because I would have to consider all possible cities in the world.
Is there any pythonic way of doing this? I thought I could use pd.merge, but I haven't found any examples of this online.
python pandas
I've been looking for an answer to this problem for a few days, but can't find anything similar in other threads.
I have a lookup table to define classification for some input data. The classification depends on continent, country and city. However, some classes may depend on a subset of these variables, e.g. only continent and country (no city). An example of such lookup table is below. In my example, I'm using one and two stars as wildcards:
- One Star: I want all cities in France to be classified as France, and
- Two Stars: All cities in US, excepting New York and San Francisco as USA - Other.
lookup_df = pd.DataFrame('Continent': ['Europe', 'Europe', 'Asia', 'America', 'America', 'America', 'America', 'Africa'],
'Country': ['France', 'Italy', 'Japan', 'USA', 'USA', 'USA', 'Argentina', '*'],
'City': ['*', '*', '*', 'New York', 'San Francisco', '**', '*', '*'],
'Classification': ['France', 'Italy', 'Japan', 'USA - NY', 'USA - SF', 'USA - Other', 'Argentina', 'Africa'])
If my dataframe is
df = pd.DataFrame('Continent': ['Europe', 'Europe', 'Asia', 'America ', 'America', 'America', 'Africa'],
'Country': ['France', 'Italy', 'Japan', 'USA', 'USA', 'USA', 'Egypt'],
'City': ['Paris', 'Rome', 'Tokyo', 'San Francisco', 'Houston', 'DC', 'Cairo'])
I am trying to get the following result:
Continent Country City Classification
0 Europe France Paris France
1 Europe Italy Rome Italy
2 Asia Japan Tokyo Japan
3 America USA San Francisco USA - SF
4 America USA Houston USA - Other
5 America USA DC USA - Other
6 Africa Egypt Cairo Africa
I need to start from a lookup table or similar because it's easier to maintain, easier to explain and it's also used by other processes. I can't create a full table, because I would have to consider all possible cities in the world.
Is there any pythonic way of doing this? I thought I could use pd.merge, but I haven't found any examples of this online.
python pandas
python pandas
edited Mar 10 at 13:02
user11209442
asked Mar 8 at 22:45
user11209442user11209442
32
32
add a comment |
add a comment |
1 Answer
1
active
oldest
votes
One easy-to-maintain way to handle your task is to use maps:
df2 = df.copy()
# below will yield a field df2.Classification and save the value when all "Continent", "Country" and "City" match, otherwise np.nan
df2 = df2.merge(lookup_df, how='left', on = ["Continent", "Country", "City"])
# create map1 from lookup_df when City is '*' but Country is not '*'
map1 = lookup_df.loc[lookup_df.City.str.match('^*+$') & ~lookup_df.Country.str.match('^*+$')].set_index(['Continent','Country']).Classification.to_dict()
map1
#('Europe', 'France'): 'France',
# ('Europe', 'Italy'): 'Italy',
# ('Asia', 'Japan'): 'Japan',
# ('America', 'USA'): 'USA - Other',
# ('America', 'Argentina'): 'Argentina'
# create map2 from lookup_df when both City and Country are '*'
map2 = lookup_df.loc[lookup_df.City.str.match('^*+$') & lookup_df.Country.str.match('^*+$')].set_index('Continent').Classification.to_dict()
map2
#'Africa': 'Africa'
# create a function to define your logic:
def set_classification(x):
return x.Classification if x.Classification is not np.nan else
map1[(x.Continent, x.Country)] if (x.Continent, x.Country) in map1 else
map2[x.Continent] if x.Continent in map2 else
np.nan
# apply the above function to each row of the df2
df2["Classification"] = df2.apply(set_classification, axis = 1)
Note: your original df.Continent
on the 4th row contains an extra trailing space 'America '
which will fail the above df2 = df2.merge(...)
line. you will need to fix this data issue though.
Thanks, it's exactly what I needed.
– user11209442
Mar 15 at 15:29
add a comment |
Your Answer
StackExchange.ifUsing("editor", function ()
StackExchange.using("externalEditor", function ()
StackExchange.using("snippets", function ()
StackExchange.snippets.init();
);
);
, "code-snippets");
StackExchange.ready(function()
var channelOptions =
tags: "".split(" "),
id: "1"
;
initTagRenderer("".split(" "), "".split(" "), channelOptions);
StackExchange.using("externalEditor", function()
// Have to fire editor after snippets, if snippets enabled
if (StackExchange.settings.snippets.snippetsEnabled)
StackExchange.using("snippets", function()
createEditor();
);
else
createEditor();
);
function createEditor()
StackExchange.prepareEditor(
heartbeatType: 'answer',
autoActivateHeartbeat: false,
convertImagesToLinks: true,
noModals: true,
showLowRepImageUploadWarning: true,
reputationToPostImages: 10,
bindNavPrevention: true,
postfix: "",
imageUploader:
brandingHtml: "Powered by u003ca class="icon-imgur-white" href="https://imgur.com/"u003eu003c/au003e",
contentPolicyHtml: "User contributions licensed under u003ca href="https://creativecommons.org/licenses/by-sa/3.0/"u003ecc by-sa 3.0 with attribution requiredu003c/au003e u003ca href="https://stackoverflow.com/legal/content-policy"u003e(content policy)u003c/au003e",
allowUrls: true
,
onDemand: true,
discardSelector: ".discard-answer"
,immediatelyShowMarkdownHelp:true
);
);
Sign up or log in
StackExchange.ready(function ()
StackExchange.helpers.onClickDraftSave('#login-link');
);
Sign up using Google
Sign up using Facebook
Sign up using Email and Password
Post as a guest
Required, but never shown
StackExchange.ready(
function ()
StackExchange.openid.initPostLogin('.new-post-login', 'https%3a%2f%2fstackoverflow.com%2fquestions%2f55072038%2flookup-table-with-wildcards-in-pandas%23new-answer', 'question_page');
);
Post as a guest
Required, but never shown
1 Answer
1
active
oldest
votes
1 Answer
1
active
oldest
votes
active
oldest
votes
active
oldest
votes
One easy-to-maintain way to handle your task is to use maps:
df2 = df.copy()
# below will yield a field df2.Classification and save the value when all "Continent", "Country" and "City" match, otherwise np.nan
df2 = df2.merge(lookup_df, how='left', on = ["Continent", "Country", "City"])
# create map1 from lookup_df when City is '*' but Country is not '*'
map1 = lookup_df.loc[lookup_df.City.str.match('^*+$') & ~lookup_df.Country.str.match('^*+$')].set_index(['Continent','Country']).Classification.to_dict()
map1
#('Europe', 'France'): 'France',
# ('Europe', 'Italy'): 'Italy',
# ('Asia', 'Japan'): 'Japan',
# ('America', 'USA'): 'USA - Other',
# ('America', 'Argentina'): 'Argentina'
# create map2 from lookup_df when both City and Country are '*'
map2 = lookup_df.loc[lookup_df.City.str.match('^*+$') & lookup_df.Country.str.match('^*+$')].set_index('Continent').Classification.to_dict()
map2
#'Africa': 'Africa'
# create a function to define your logic:
def set_classification(x):
return x.Classification if x.Classification is not np.nan else
map1[(x.Continent, x.Country)] if (x.Continent, x.Country) in map1 else
map2[x.Continent] if x.Continent in map2 else
np.nan
# apply the above function to each row of the df2
df2["Classification"] = df2.apply(set_classification, axis = 1)
Note: your original df.Continent
on the 4th row contains an extra trailing space 'America '
which will fail the above df2 = df2.merge(...)
line. you will need to fix this data issue though.
Thanks, it's exactly what I needed.
– user11209442
Mar 15 at 15:29
add a comment |
One easy-to-maintain way to handle your task is to use maps:
df2 = df.copy()
# below will yield a field df2.Classification and save the value when all "Continent", "Country" and "City" match, otherwise np.nan
df2 = df2.merge(lookup_df, how='left', on = ["Continent", "Country", "City"])
# create map1 from lookup_df when City is '*' but Country is not '*'
map1 = lookup_df.loc[lookup_df.City.str.match('^*+$') & ~lookup_df.Country.str.match('^*+$')].set_index(['Continent','Country']).Classification.to_dict()
map1
#('Europe', 'France'): 'France',
# ('Europe', 'Italy'): 'Italy',
# ('Asia', 'Japan'): 'Japan',
# ('America', 'USA'): 'USA - Other',
# ('America', 'Argentina'): 'Argentina'
# create map2 from lookup_df when both City and Country are '*'
map2 = lookup_df.loc[lookup_df.City.str.match('^*+$') & lookup_df.Country.str.match('^*+$')].set_index('Continent').Classification.to_dict()
map2
#'Africa': 'Africa'
# create a function to define your logic:
def set_classification(x):
return x.Classification if x.Classification is not np.nan else
map1[(x.Continent, x.Country)] if (x.Continent, x.Country) in map1 else
map2[x.Continent] if x.Continent in map2 else
np.nan
# apply the above function to each row of the df2
df2["Classification"] = df2.apply(set_classification, axis = 1)
Note: your original df.Continent
on the 4th row contains an extra trailing space 'America '
which will fail the above df2 = df2.merge(...)
line. you will need to fix this data issue though.
Thanks, it's exactly what I needed.
– user11209442
Mar 15 at 15:29
add a comment |
One easy-to-maintain way to handle your task is to use maps:
df2 = df.copy()
# below will yield a field df2.Classification and save the value when all "Continent", "Country" and "City" match, otherwise np.nan
df2 = df2.merge(lookup_df, how='left', on = ["Continent", "Country", "City"])
# create map1 from lookup_df when City is '*' but Country is not '*'
map1 = lookup_df.loc[lookup_df.City.str.match('^*+$') & ~lookup_df.Country.str.match('^*+$')].set_index(['Continent','Country']).Classification.to_dict()
map1
#('Europe', 'France'): 'France',
# ('Europe', 'Italy'): 'Italy',
# ('Asia', 'Japan'): 'Japan',
# ('America', 'USA'): 'USA - Other',
# ('America', 'Argentina'): 'Argentina'
# create map2 from lookup_df when both City and Country are '*'
map2 = lookup_df.loc[lookup_df.City.str.match('^*+$') & lookup_df.Country.str.match('^*+$')].set_index('Continent').Classification.to_dict()
map2
#'Africa': 'Africa'
# create a function to define your logic:
def set_classification(x):
return x.Classification if x.Classification is not np.nan else
map1[(x.Continent, x.Country)] if (x.Continent, x.Country) in map1 else
map2[x.Continent] if x.Continent in map2 else
np.nan
# apply the above function to each row of the df2
df2["Classification"] = df2.apply(set_classification, axis = 1)
Note: your original df.Continent
on the 4th row contains an extra trailing space 'America '
which will fail the above df2 = df2.merge(...)
line. you will need to fix this data issue though.
One easy-to-maintain way to handle your task is to use maps:
df2 = df.copy()
# below will yield a field df2.Classification and save the value when all "Continent", "Country" and "City" match, otherwise np.nan
df2 = df2.merge(lookup_df, how='left', on = ["Continent", "Country", "City"])
# create map1 from lookup_df when City is '*' but Country is not '*'
map1 = lookup_df.loc[lookup_df.City.str.match('^*+$') & ~lookup_df.Country.str.match('^*+$')].set_index(['Continent','Country']).Classification.to_dict()
map1
#('Europe', 'France'): 'France',
# ('Europe', 'Italy'): 'Italy',
# ('Asia', 'Japan'): 'Japan',
# ('America', 'USA'): 'USA - Other',
# ('America', 'Argentina'): 'Argentina'
# create map2 from lookup_df when both City and Country are '*'
map2 = lookup_df.loc[lookup_df.City.str.match('^*+$') & lookup_df.Country.str.match('^*+$')].set_index('Continent').Classification.to_dict()
map2
#'Africa': 'Africa'
# create a function to define your logic:
def set_classification(x):
return x.Classification if x.Classification is not np.nan else
map1[(x.Continent, x.Country)] if (x.Continent, x.Country) in map1 else
map2[x.Continent] if x.Continent in map2 else
np.nan
# apply the above function to each row of the df2
df2["Classification"] = df2.apply(set_classification, axis = 1)
Note: your original df.Continent
on the 4th row contains an extra trailing space 'America '
which will fail the above df2 = df2.merge(...)
line. you will need to fix this data issue though.
answered Mar 11 at 18:26


jxcjxc
1,098139
1,098139
Thanks, it's exactly what I needed.
– user11209442
Mar 15 at 15:29
add a comment |
Thanks, it's exactly what I needed.
– user11209442
Mar 15 at 15:29
Thanks, it's exactly what I needed.
– user11209442
Mar 15 at 15:29
Thanks, it's exactly what I needed.
– user11209442
Mar 15 at 15:29
add a comment |
Thanks for contributing an answer to Stack Overflow!
- Please be sure to answer the question. Provide details and share your research!
But avoid …
- Asking for help, clarification, or responding to other answers.
- Making statements based on opinion; back them up with references or personal experience.
To learn more, see our tips on writing great answers.
Sign up or log in
StackExchange.ready(function ()
StackExchange.helpers.onClickDraftSave('#login-link');
);
Sign up using Google
Sign up using Facebook
Sign up using Email and Password
Post as a guest
Required, but never shown
StackExchange.ready(
function ()
StackExchange.openid.initPostLogin('.new-post-login', 'https%3a%2f%2fstackoverflow.com%2fquestions%2f55072038%2flookup-table-with-wildcards-in-pandas%23new-answer', 'question_page');
);
Post as a guest
Required, but never shown
Sign up or log in
StackExchange.ready(function ()
StackExchange.helpers.onClickDraftSave('#login-link');
);
Sign up using Google
Sign up using Facebook
Sign up using Email and Password
Post as a guest
Required, but never shown
Sign up or log in
StackExchange.ready(function ()
StackExchange.helpers.onClickDraftSave('#login-link');
);
Sign up using Google
Sign up using Facebook
Sign up using Email and Password
Post as a guest
Required, but never shown
Sign up or log in
StackExchange.ready(function ()
StackExchange.helpers.onClickDraftSave('#login-link');
);
Sign up using Google
Sign up using Facebook
Sign up using Email and Password
Sign up using Google
Sign up using Facebook
Sign up using Email and Password
Post as a guest
Required, but never shown
Required, but never shown
Required, but never shown
Required, but never shown
Required, but never shown
Required, but never shown
Required, but never shown
Required, but never shown
Required, but never shown
K5 maYJI3z j,m HdB53HZMsJbTX1hWhNAJZLF 02DI7 h68fim92FiFMeb3Fw Q,paimaor1NIiDc F79v8kRNImk0oHHGn7gd