Understanding generators in Python2019 Community Moderator ElectionGenerator function not working pythonFor loop not executing two timesGenerators - Printing generated valuesWhy can a python generator only be used once?What exactly do generators do?What does the “yield” keyword do?What does “list comprehension” mean? How does it work and how can I use it?sklearn Kfold acces single fold instead of for loopIs a generator the callable? Which is the generator?Apply Border To Range Of Cells Using OpenpyxlCalling an external command in PythonWhat are metaclasses in Python?What is the difference between @staticmethod and @classmethod?Finding the index of an item given a list containing it in PythonDifference between append vs. extend list methods in PythonHow can I safely create a nested directory in Python?Does Python have a ternary conditional operator?Understanding slice notationUnderstanding Python super() with __init__() methodsDoes Python have a string 'contains' substring method?
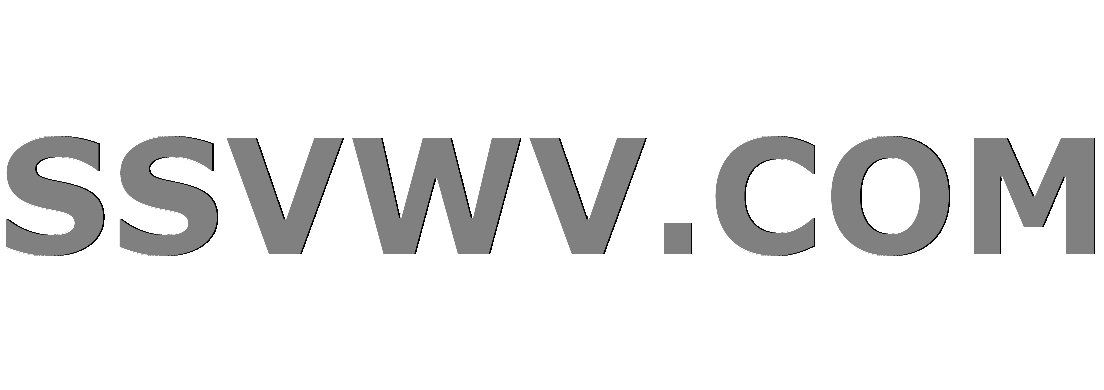
Multi tool use
What is it called when someone votes for an option that's not their first choice?
Have the tides ever turned twice on any open problem?
Is xar preinstalled on macOS?
"Marked down as someone wanting to sell shares." What does that mean?
Does fire aspect on a sword, destroy mob drops?
Is VPN a layer 3 concept?
Exposing a company lying about themselves in a tightly knit industry: Is my career at risk on the long run?
Symbolism of 18 Journeyers
How can a new country break out from a developed country without war?
What is the difference between something being completely legal and being completely decriminalized?
Does convergence of polynomials imply that of its coefficients?
Friend wants my recommendation but I don't want to give it to him
Why I don't get the wanted width of tcbox?
Single word to change groups
Would this string work as string?
Unfrosted light bulb
Air travel with refrigerated insulin
What is the tangent at a sharp point on a curve?
When did hardware antialiasing start being available?
When should a starting writer get his own webpage?
Asserting that Atheism and Theism are both faith based positions
How to balance a monster modification (zombie)?
Could any one tell what PN is this Chip? Thanks~
Writing in a Christian voice
Understanding generators in Python
2019 Community Moderator ElectionGenerator function not working pythonFor loop not executing two timesGenerators - Printing generated valuesWhy can a python generator only be used once?What exactly do generators do?What does the “yield” keyword do?What does “list comprehension” mean? How does it work and how can I use it?sklearn Kfold acces single fold instead of for loopIs a generator the callable? Which is the generator?Apply Border To Range Of Cells Using OpenpyxlCalling an external command in PythonWhat are metaclasses in Python?What is the difference between @staticmethod and @classmethod?Finding the index of an item given a list containing it in PythonDifference between append vs. extend list methods in PythonHow can I safely create a nested directory in Python?Does Python have a ternary conditional operator?Understanding slice notationUnderstanding Python super() with __init__() methodsDoes Python have a string 'contains' substring method?
I am reading the Python cookbook at the moment and am currently looking at generators. I'm finding it hard to get my head round.
As I come from a Java background, is there a Java equivalent? The book was speaking about 'Producer / Consumer', however when I hear that I think of threading.
What is a generator and why would you use it? Without quoting any books, obviously (unless you can find a decent, simplistic answer direct from a book). Perhaps with examples, if you're feeling generous!
python generator
add a comment |
I am reading the Python cookbook at the moment and am currently looking at generators. I'm finding it hard to get my head round.
As I come from a Java background, is there a Java equivalent? The book was speaking about 'Producer / Consumer', however when I hear that I think of threading.
What is a generator and why would you use it? Without quoting any books, obviously (unless you can find a decent, simplistic answer direct from a book). Perhaps with examples, if you're feeling generous!
python generator
add a comment |
I am reading the Python cookbook at the moment and am currently looking at generators. I'm finding it hard to get my head round.
As I come from a Java background, is there a Java equivalent? The book was speaking about 'Producer / Consumer', however when I hear that I think of threading.
What is a generator and why would you use it? Without quoting any books, obviously (unless you can find a decent, simplistic answer direct from a book). Perhaps with examples, if you're feeling generous!
python generator
I am reading the Python cookbook at the moment and am currently looking at generators. I'm finding it hard to get my head round.
As I come from a Java background, is there a Java equivalent? The book was speaking about 'Producer / Consumer', however when I hear that I think of threading.
What is a generator and why would you use it? Without quoting any books, obviously (unless you can find a decent, simplistic answer direct from a book). Perhaps with examples, if you're feeling generous!
python generator
python generator
edited May 20 '18 at 8:44


Peter Mortensen
13.8k1987113
13.8k1987113
asked Nov 18 '09 at 13:46


FedererFederer
12.8k3584117
12.8k3584117
add a comment |
add a comment |
11 Answers
11
active
oldest
votes
Note: this post assumes Python 3.x syntax.†
A generator is simply a function which returns an object on which you can call next
, such that for every call it returns some value, until it raises a StopIteration
exception, signaling that all values have been generated. Such an object is called an iterator.
Normal functions return a single value using return
, just like in Java. In Python, however, there is an alternative, called yield
. Using yield
anywhere in a function makes it a generator. Observe this code:
>>> def myGen(n):
... yield n
... yield n + 1
...
>>> g = myGen(6)
>>> next(g)
6
>>> next(g)
7
>>> next(g)
Traceback (most recent call last):
File "<stdin>", line 1, in <module>
StopIteration
As you can see, myGen(n)
is a function which yields n
and n + 1
. Every call to next
yields a single value, until all values have been yielded. for
loops call next
in the background, thus:
>>> for n in myGen(6):
... print(n)
...
6
7
Likewise there are generator expressions, which provide a means to succinctly describe certain common types of generators:
>>> g = (n for n in range(3, 5))
>>> next(g)
3
>>> next(g)
4
>>> next(g)
Traceback (most recent call last):
File "<stdin>", line 1, in <module>
StopIteration
Note that generator expressions are much like list comprehensions:
>>> lc = [n for n in range(3, 5)]
>>> lc
[3, 4]
Observe that a generator object is generated once, but its code is not run all at once. Only calls to next
actually execute (part of) the code. Execution of the code in a generator stops once a yield
statement has been reached, upon which it returns a value. The next call to next
then causes execution to continue in the state in which the generator was left after the last yield
. This is a fundamental difference with regular functions: those always start execution at the "top" and discard their state upon returning a value.
There are more things to be said about this subject. It is e.g. possible to send
data back into a generator (reference). But that is something I suggest you do not look into until you understand the basic concept of a generator.
Now you may ask: why use generators? There are a couple of good reasons:
- Certain concepts can be described much more succinctly using generators.
- Instead of creating a function which returns a list of values, one can write a generator which generates the values on the fly. This means that no list needs to be constructed, meaning that the resulting code is more memory efficient. In this way one can even describe data streams which would simply be too large to fit in memory.
Generators allow for a natural way to describe infinite streams. Consider for example the Fibonacci numbers:
>>> def fib():
... a, b = 0, 1
... while True:
... yield a
... a, b = b, a + b
...
>>> import itertools
>>> list(itertools.islice(fib(), 10))
[0, 1, 1, 2, 3, 5, 8, 13, 21, 34]This code uses
itertools.islice
to take a finite number of elements from an infinite stream. You are advised to have a good look at the functions in theitertools
module, as they are essential tools for writing advanced generators with great ease.
†About Python <=2.6: in the above examples next
is a function which calls the method __next__
on the given object. In Python <=2.6 one uses a slightly different technique, namely o.next()
instead of next(o)
. Python 2.7 has next()
call .next
so you need not use the following in 2.7:
>>> g = (n for n in range(3, 5))
>>> g.next()
3
7
You mention it is possible tosend
data to a generator. Once you do that you have a 'coroutine'. It's very simple to implement patterns like the mentioned Consumer/Producer with coroutines because they have no need forLock
s and therefore can't deadlock. It's hard to describe coroutines without bashing threads, so I'll just say coroutines are a very elegant alternative to threading.
– Jochen Ritzel
Nov 18 '09 at 14:47
Are Python generators basically Turing machines in terms of how they function?
– Fiery Phoenix
Sep 23 '16 at 23:34
Thank you for the references and mentioning itertools for usage of generators.
– pyeR_biz
Aug 3 '18 at 1:13
add a comment |
A generator is effectively a function that returns (data) before it is finished, but it pauses at that point, and you can resume the function at that point.
>>> def myGenerator():
... yield 'These'
... yield 'words'
... yield 'come'
... yield 'one'
... yield 'at'
... yield 'a'
... yield 'time'
>>> myGeneratorInstance = myGenerator()
>>> next(myGeneratorInstance)
These
>>> next(myGeneratorInstance)
words
and so on. The (or one) benefit of generators is that because they deal with data one piece at a time, you can deal with large amounts of data; with lists, excessive memory requirements could become a problem. Generators, just like lists, are iterable, so they can be used in the same ways:
>>> for word in myGeneratorInstance:
... print word
These
words
come
one
at
a
time
Note that generators provide another way to deal with infinity, for example
>>> from time import gmtime, strftime
>>> def myGen():
... while True:
... yield strftime("%a, %d %b %Y %H:%M:%S +0000", gmtime())
>>> myGeneratorInstance = myGen()
>>> next(myGeneratorInstance)
Thu, 28 Jun 2001 14:17:15 +0000
>>> next(myGeneratorInstance)
Thu, 28 Jun 2001 14:18:02 +0000
The generator encapsulates an infinite loop, but this isn't a problem because you only get each answer every time you ask for it.
add a comment |
First of all, the term generator originally was somewhat ill-defined in Python, leading to lots of confusion. You probably mean iterators and iterables (see here). Then in Python there are also generator functions (which return a generator object), generator objects (which are iterators) and generator expressions (which are evaluated to a generator object).
According to the glossary entry for generator it seems that the official terminology is now that generator is short for "generator function". In the past the documentation defined the terms inconsistently, but fortunately this has been fixed.
It might still be a good idea to be precise and avoid the term "generator" without further specification.
2
Hmm I think you're right, at least according to a test of a few lines in Python 2.6. A generator expression returns an iterator (aka 'generator object'), not a generator.
– Craig McQueen
Dec 4 '09 at 1:34
add a comment |
Generators could be thought of as shorthand for creating an iterator. They behave like a Java Iterator. Example:
>>> g = (x for x in range(10))
>>> g
<generator object <genexpr> at 0x7fac1c1e6aa0>
>>> g.next()
0
>>> g.next()
1
>>> g.next()
2
>>> list(g) # force iterating the rest
[3, 4, 5, 6, 7, 8, 9]
>>> g.next() # iterator is at the end; calling next again will throw
Traceback (most recent call last):
File "<stdin>", line 1, in <module>
StopIteration
Hope this helps/is what you are looking for.
Update:
As many other answers are showing, there are different ways to create a generator. You can use the parentheses syntax as in my example above, or you can use yield. Another interesting feature is that generators can be "infinite" -- iterators that don't stop:
>>> def infinite_gen():
... n = 0
... while True:
... yield n
... n = n + 1
...
>>> g = infinite_gen()
>>> g.next()
0
>>> g.next()
1
>>> g.next()
2
>>> g.next()
3
...
Now, Java hasStream
s, which are far more similar to generators, except that you apparently can't just get the next element without a surprising amount of hassle.
– Nic Hartley
Apr 29 '16 at 20:36
add a comment |
There is no Java equivalent.
Here is a bit of a contrived example:
#! /usr/bin/python
def mygen(n):
x = 0
while x < n:
x = x + 1
if x % 3 == 0:
yield x
for a in mygen(100):
print a
There is a loop in the generator that runs from 0 to n, and if the loop variable is a multiple of 3, it yields the variable.
During each iteration of the for
loop the generator is executed. If it is the first time the generator executes, it starts at the beginning, otherwise it continues from the previous time it yielded.
2
The last paragraph is very important: The state of the generator function is 'frozen' everytime it yields sth, and continues in exactly the same state when it is invoked the next time.
– Johannes Charra
Nov 18 '09 at 14:12
There's no syntactic equivalent in Java to a "generator expression", but generators -- once you've got one -- are essentially just an iterator (same basic characteristics as a Java iterator).
– overthink
Nov 18 '09 at 14:21
@overthink: Well, generators can have other side effects that Java iterators can't have. If I were to putprint "hello"
after thex=x+1
in my example, "hello" would be printed 100 times, while the body of the for loop would still only be executed 33 times.
– Wernsey
Nov 18 '09 at 15:02
@iWerner: Pretty sure the same effect could be had in Java. The implementation of next() in the equivalent Java iterator would still have to search from 0 to 99 (using your mygen(100) example), so you could System.out.println() each time if you wanted. You'd only return 33 times from next() though. What Java lacks is the very handy yield syntax which is significantly easier to read (and write).
– overthink
Nov 18 '09 at 15:54
add a comment |
I like to describe generators, to those with a decent background in programming languages and computing, in terms of stack frames.
In many languages, there is a stack on top of which is the current stack "frame". The stack frame includes space allocated for variables local to the function including the arguments passed in to that function.
When you call a function, the current point of execution (the "program counter" or equivalent) is pushed onto the stack, and a new stack frame is created. Execution then transfers to the beginning of the function being called.
With regular functions, at some point the function returns a value, and the stack is "popped". The function's stack frame is discarded and execution resumes at the previous location.
When a function is a generator, it can return a value without the stack frame being discarded, using the yield statement. The values of local variables and the program counter within the function are preserved. This allows the generator to be resumed at a later time, with execution continuing from the yield statement, and it can execute more code and return another value.
Before Python 2.5 this was all generators did. Python 2.5 added the ability to pass values back in to the generator as well. In doing so, the passed-in value is available as an expression resulting from the yield statement which had temporarily returned control (and a value) from the generator.
The key advantage to generators is that the "state" of the function is preserved, unlike with regular functions where each time the stack frame is discarded, you lose all that "state". A secondary advantage is that some of the function call overhead (creating and deleting stack frames) is avoided, though this is a usually a minor advantage.
add a comment |
The only thing I can add to Stephan202's answer is a recommendation that you take a look at David Beazley's PyCon '08 presentation "Generator Tricks for Systems Programmers," which is the best single explanation of the how and why of generators that I've seen anywhere. This is the thing that took me from "Python looks kind of fun" to "This is what I've been looking for." It's at http://www.dabeaz.com/generators/.
add a comment |
It helps to make a clear distinction between the function foo, and the generator foo(n):
def foo(n):
yield n
yield n+1
foo is a function.
foo(6) is a generator object.
The typical way to use a generator object is in a loop:
for n in foo(6):
print(n)
The loop prints
# 6
# 7
Think of a generator as a resumable function.
yield
behaves like return
in the sense that values that are yielded get "returned" by the generator. Unlike return, however, the next time the generator gets asked for a value, the generator's function, foo, resumes where it left off -- after the last yield statement -- and continues to run until it hits another yield statement.
Behind the scenes, when you call bar=foo(6)
the generator object bar is defined for you to have a next
attribute.
You can call it yourself to retrieve values yielded from foo:
next(bar) # Works in Python 2.6 or Python 3.x
bar.next() # Works in Python 2.5+, but is deprecated. Use next() if possible.
When foo ends (and there are no more yielded values), calling next(bar)
throws a StopInteration error.
add a comment |
This post will use Fibonacci numbers as a tool to build up to explaining the usefulness of Python generators.
This post will feature both C++ and Python code.
Fibonacci numbers are defined as the sequence: 0, 1, 1, 2, 3, 5, 8, 13, 21, 34, ....
Or in general:
F0 = 0
F1 = 1
Fn = Fn-1 + Fn-2
This can be transferred into a C++ function extremely easily:
size_t Fib(size_t n)
//Fib(0) = 0
if(n == 0)
return 0;
//Fib(1) = 1
if(n == 1)
return 1;
//Fib(N) = Fib(N-2) + Fib(N-1)
return Fib(n-2) + Fib(n-1);
But if you want to print the first six Fibonacci numbers, you will be recalculating a lot of the values with the above function.
For example: Fib(3) = Fib(2) + Fib(1)
, but Fib(2)
also recalculates Fib(1)
. The higher the value you want to calculate, the worse off you will be.
So one may be tempted to rewrite the above by keeping track of the state in main
.
// Not supported for the first two elements of Fib
size_t GetNextFib(size_t &pp, size_t &p)
int result = pp + p;
pp = p;
p = result;
return result;
int main(int argc, char *argv[])
size_t pp = 0;
size_t p = 1;
std::cout << "0 " << "1 ";
for(size_t i = 0; i <= 4; ++i)
size_t fibI = GetNextFib(pp, p);
std::cout << fibI << " ";
return 0;
But this is very ugly, and it complicates our logic in main
. It would be better to not have to worry about state in our main
function.
We could return a vector
of values and use an iterator
to iterate over that set of values, but this requires a lot of memory all at once for a large number of return values.
So back to our old approach, what happens if we wanted to do something else besides print the numbers? We'd have to copy and paste the whole block of code in main
and change the output statements to whatever else we wanted to do.
And if you copy and paste code, then you should be shot. You don't want to get shot, do you?
To solve these problems, and to avoid getting shot, we may rewrite this block of code using a callback function. Every time a new Fibonacci number is encountered, we would call the callback function.
void GetFibNumbers(size_t max, void(*FoundNewFibCallback)(size_t))
if(max-- == 0) return;
FoundNewFibCallback(0);
if(max-- == 0) return;
FoundNewFibCallback(1);
size_t pp = 0;
size_t p = 1;
for(;;)
if(max-- == 0) return;
int result = pp + p;
pp = p;
p = result;
FoundNewFibCallback(result);
void foundNewFib(size_t fibI)
std::cout << fibI << " ";
int main(int argc, char *argv[])
GetFibNumbers(6, foundNewFib);
return 0;
This is clearly an improvement, your logic in main
is not as cluttered, and you can do anything you want with the Fibonacci numbers, simply define new callbacks.
But this is still not perfect. What if you wanted to only get the first two Fibonacci numbers, and then do something, then get some more, then do something else?
Well, we could go on like we have been, and we could start adding state again into main
, allowing GetFibNumbers to start from an arbitrary point.
But this will further bloat our code, and it already looks too big for a simple task like printing Fibonacci numbers.
We could implement a producer and consumer model via a couple of threads. But this complicates the code even more.
Instead let's talk about generators.
Python has a very nice language feature that solves problems like these called generators.
A generator allows you to execute a function, stop at an arbitrary point, and then continue again where you left off.
Each time returning a value.
Consider the following code that uses a generator:
def fib():
pp, p = 0, 1
while 1:
yield pp
pp, p = p, pp+p
g = fib()
for i in range(6):
g.next()
Which gives us the results:
0
1
1
2
3
5
The yield
statement is used in conjuction with Python generators. It saves the state of the function and returns the yeilded value. The next time you call the next() function on the generator, it will continue where the yield left off.
This is by far more clean than the callback function code. We have cleaner code, smaller code, and not to mention much more functional code (Python allows arbitrarily large integers).
Source
add a comment |
I believe the first appearance of iterators and generators were in the Icon programming language, about 20 years ago.
You may enjoy the Icon overview, which lets you wrap your head around them without concentrating on the syntax (since Icon is a language you probably don't know, and Griswold was explaining the benefits of his language to people coming from other languages).
After reading just a few paragraphs there, the utility of generators and iterators might become more apparent.
add a comment |
Experience with list comprehensions has shown their widespread utility throughout Python. However, many of the use cases do not need to have a full list created in memory. Instead, they only need to iterate over the elements one at a time.
For instance, the following summation code will build a full list of squares in memory, iterate over those values, and, when the reference is no longer needed, delete the list:
sum([x*x for x in range(10)])
Memory is conserved by using a generator expression instead:
sum(x*x for x in range(10))
Similar benefits are conferred on constructors for container objects:
s = Set(word for line in page for word in line.split())
d = dict( (k, func(k)) for k in keylist)
Generator expressions are especially useful with functions like sum(), min(), and max() that reduce an iterable input to a single value:
max(len(line) for line in file if line.strip())
more
add a comment |
protected by Marcin May 15 '13 at 21:56
Thank you for your interest in this question.
Because it has attracted low-quality or spam answers that had to be removed, posting an answer now requires 10 reputation on this site (the association bonus does not count).
Would you like to answer one of these unanswered questions instead?
11 Answers
11
active
oldest
votes
11 Answers
11
active
oldest
votes
active
oldest
votes
active
oldest
votes
Note: this post assumes Python 3.x syntax.†
A generator is simply a function which returns an object on which you can call next
, such that for every call it returns some value, until it raises a StopIteration
exception, signaling that all values have been generated. Such an object is called an iterator.
Normal functions return a single value using return
, just like in Java. In Python, however, there is an alternative, called yield
. Using yield
anywhere in a function makes it a generator. Observe this code:
>>> def myGen(n):
... yield n
... yield n + 1
...
>>> g = myGen(6)
>>> next(g)
6
>>> next(g)
7
>>> next(g)
Traceback (most recent call last):
File "<stdin>", line 1, in <module>
StopIteration
As you can see, myGen(n)
is a function which yields n
and n + 1
. Every call to next
yields a single value, until all values have been yielded. for
loops call next
in the background, thus:
>>> for n in myGen(6):
... print(n)
...
6
7
Likewise there are generator expressions, which provide a means to succinctly describe certain common types of generators:
>>> g = (n for n in range(3, 5))
>>> next(g)
3
>>> next(g)
4
>>> next(g)
Traceback (most recent call last):
File "<stdin>", line 1, in <module>
StopIteration
Note that generator expressions are much like list comprehensions:
>>> lc = [n for n in range(3, 5)]
>>> lc
[3, 4]
Observe that a generator object is generated once, but its code is not run all at once. Only calls to next
actually execute (part of) the code. Execution of the code in a generator stops once a yield
statement has been reached, upon which it returns a value. The next call to next
then causes execution to continue in the state in which the generator was left after the last yield
. This is a fundamental difference with regular functions: those always start execution at the "top" and discard their state upon returning a value.
There are more things to be said about this subject. It is e.g. possible to send
data back into a generator (reference). But that is something I suggest you do not look into until you understand the basic concept of a generator.
Now you may ask: why use generators? There are a couple of good reasons:
- Certain concepts can be described much more succinctly using generators.
- Instead of creating a function which returns a list of values, one can write a generator which generates the values on the fly. This means that no list needs to be constructed, meaning that the resulting code is more memory efficient. In this way one can even describe data streams which would simply be too large to fit in memory.
Generators allow for a natural way to describe infinite streams. Consider for example the Fibonacci numbers:
>>> def fib():
... a, b = 0, 1
... while True:
... yield a
... a, b = b, a + b
...
>>> import itertools
>>> list(itertools.islice(fib(), 10))
[0, 1, 1, 2, 3, 5, 8, 13, 21, 34]This code uses
itertools.islice
to take a finite number of elements from an infinite stream. You are advised to have a good look at the functions in theitertools
module, as they are essential tools for writing advanced generators with great ease.
†About Python <=2.6: in the above examples next
is a function which calls the method __next__
on the given object. In Python <=2.6 one uses a slightly different technique, namely o.next()
instead of next(o)
. Python 2.7 has next()
call .next
so you need not use the following in 2.7:
>>> g = (n for n in range(3, 5))
>>> g.next()
3
7
You mention it is possible tosend
data to a generator. Once you do that you have a 'coroutine'. It's very simple to implement patterns like the mentioned Consumer/Producer with coroutines because they have no need forLock
s and therefore can't deadlock. It's hard to describe coroutines without bashing threads, so I'll just say coroutines are a very elegant alternative to threading.
– Jochen Ritzel
Nov 18 '09 at 14:47
Are Python generators basically Turing machines in terms of how they function?
– Fiery Phoenix
Sep 23 '16 at 23:34
Thank you for the references and mentioning itertools for usage of generators.
– pyeR_biz
Aug 3 '18 at 1:13
add a comment |
Note: this post assumes Python 3.x syntax.†
A generator is simply a function which returns an object on which you can call next
, such that for every call it returns some value, until it raises a StopIteration
exception, signaling that all values have been generated. Such an object is called an iterator.
Normal functions return a single value using return
, just like in Java. In Python, however, there is an alternative, called yield
. Using yield
anywhere in a function makes it a generator. Observe this code:
>>> def myGen(n):
... yield n
... yield n + 1
...
>>> g = myGen(6)
>>> next(g)
6
>>> next(g)
7
>>> next(g)
Traceback (most recent call last):
File "<stdin>", line 1, in <module>
StopIteration
As you can see, myGen(n)
is a function which yields n
and n + 1
. Every call to next
yields a single value, until all values have been yielded. for
loops call next
in the background, thus:
>>> for n in myGen(6):
... print(n)
...
6
7
Likewise there are generator expressions, which provide a means to succinctly describe certain common types of generators:
>>> g = (n for n in range(3, 5))
>>> next(g)
3
>>> next(g)
4
>>> next(g)
Traceback (most recent call last):
File "<stdin>", line 1, in <module>
StopIteration
Note that generator expressions are much like list comprehensions:
>>> lc = [n for n in range(3, 5)]
>>> lc
[3, 4]
Observe that a generator object is generated once, but its code is not run all at once. Only calls to next
actually execute (part of) the code. Execution of the code in a generator stops once a yield
statement has been reached, upon which it returns a value. The next call to next
then causes execution to continue in the state in which the generator was left after the last yield
. This is a fundamental difference with regular functions: those always start execution at the "top" and discard their state upon returning a value.
There are more things to be said about this subject. It is e.g. possible to send
data back into a generator (reference). But that is something I suggest you do not look into until you understand the basic concept of a generator.
Now you may ask: why use generators? There are a couple of good reasons:
- Certain concepts can be described much more succinctly using generators.
- Instead of creating a function which returns a list of values, one can write a generator which generates the values on the fly. This means that no list needs to be constructed, meaning that the resulting code is more memory efficient. In this way one can even describe data streams which would simply be too large to fit in memory.
Generators allow for a natural way to describe infinite streams. Consider for example the Fibonacci numbers:
>>> def fib():
... a, b = 0, 1
... while True:
... yield a
... a, b = b, a + b
...
>>> import itertools
>>> list(itertools.islice(fib(), 10))
[0, 1, 1, 2, 3, 5, 8, 13, 21, 34]This code uses
itertools.islice
to take a finite number of elements from an infinite stream. You are advised to have a good look at the functions in theitertools
module, as they are essential tools for writing advanced generators with great ease.
†About Python <=2.6: in the above examples next
is a function which calls the method __next__
on the given object. In Python <=2.6 one uses a slightly different technique, namely o.next()
instead of next(o)
. Python 2.7 has next()
call .next
so you need not use the following in 2.7:
>>> g = (n for n in range(3, 5))
>>> g.next()
3
7
You mention it is possible tosend
data to a generator. Once you do that you have a 'coroutine'. It's very simple to implement patterns like the mentioned Consumer/Producer with coroutines because they have no need forLock
s and therefore can't deadlock. It's hard to describe coroutines without bashing threads, so I'll just say coroutines are a very elegant alternative to threading.
– Jochen Ritzel
Nov 18 '09 at 14:47
Are Python generators basically Turing machines in terms of how they function?
– Fiery Phoenix
Sep 23 '16 at 23:34
Thank you for the references and mentioning itertools for usage of generators.
– pyeR_biz
Aug 3 '18 at 1:13
add a comment |
Note: this post assumes Python 3.x syntax.†
A generator is simply a function which returns an object on which you can call next
, such that for every call it returns some value, until it raises a StopIteration
exception, signaling that all values have been generated. Such an object is called an iterator.
Normal functions return a single value using return
, just like in Java. In Python, however, there is an alternative, called yield
. Using yield
anywhere in a function makes it a generator. Observe this code:
>>> def myGen(n):
... yield n
... yield n + 1
...
>>> g = myGen(6)
>>> next(g)
6
>>> next(g)
7
>>> next(g)
Traceback (most recent call last):
File "<stdin>", line 1, in <module>
StopIteration
As you can see, myGen(n)
is a function which yields n
and n + 1
. Every call to next
yields a single value, until all values have been yielded. for
loops call next
in the background, thus:
>>> for n in myGen(6):
... print(n)
...
6
7
Likewise there are generator expressions, which provide a means to succinctly describe certain common types of generators:
>>> g = (n for n in range(3, 5))
>>> next(g)
3
>>> next(g)
4
>>> next(g)
Traceback (most recent call last):
File "<stdin>", line 1, in <module>
StopIteration
Note that generator expressions are much like list comprehensions:
>>> lc = [n for n in range(3, 5)]
>>> lc
[3, 4]
Observe that a generator object is generated once, but its code is not run all at once. Only calls to next
actually execute (part of) the code. Execution of the code in a generator stops once a yield
statement has been reached, upon which it returns a value. The next call to next
then causes execution to continue in the state in which the generator was left after the last yield
. This is a fundamental difference with regular functions: those always start execution at the "top" and discard their state upon returning a value.
There are more things to be said about this subject. It is e.g. possible to send
data back into a generator (reference). But that is something I suggest you do not look into until you understand the basic concept of a generator.
Now you may ask: why use generators? There are a couple of good reasons:
- Certain concepts can be described much more succinctly using generators.
- Instead of creating a function which returns a list of values, one can write a generator which generates the values on the fly. This means that no list needs to be constructed, meaning that the resulting code is more memory efficient. In this way one can even describe data streams which would simply be too large to fit in memory.
Generators allow for a natural way to describe infinite streams. Consider for example the Fibonacci numbers:
>>> def fib():
... a, b = 0, 1
... while True:
... yield a
... a, b = b, a + b
...
>>> import itertools
>>> list(itertools.islice(fib(), 10))
[0, 1, 1, 2, 3, 5, 8, 13, 21, 34]This code uses
itertools.islice
to take a finite number of elements from an infinite stream. You are advised to have a good look at the functions in theitertools
module, as they are essential tools for writing advanced generators with great ease.
†About Python <=2.6: in the above examples next
is a function which calls the method __next__
on the given object. In Python <=2.6 one uses a slightly different technique, namely o.next()
instead of next(o)
. Python 2.7 has next()
call .next
so you need not use the following in 2.7:
>>> g = (n for n in range(3, 5))
>>> g.next()
3
Note: this post assumes Python 3.x syntax.†
A generator is simply a function which returns an object on which you can call next
, such that for every call it returns some value, until it raises a StopIteration
exception, signaling that all values have been generated. Such an object is called an iterator.
Normal functions return a single value using return
, just like in Java. In Python, however, there is an alternative, called yield
. Using yield
anywhere in a function makes it a generator. Observe this code:
>>> def myGen(n):
... yield n
... yield n + 1
...
>>> g = myGen(6)
>>> next(g)
6
>>> next(g)
7
>>> next(g)
Traceback (most recent call last):
File "<stdin>", line 1, in <module>
StopIteration
As you can see, myGen(n)
is a function which yields n
and n + 1
. Every call to next
yields a single value, until all values have been yielded. for
loops call next
in the background, thus:
>>> for n in myGen(6):
... print(n)
...
6
7
Likewise there are generator expressions, which provide a means to succinctly describe certain common types of generators:
>>> g = (n for n in range(3, 5))
>>> next(g)
3
>>> next(g)
4
>>> next(g)
Traceback (most recent call last):
File "<stdin>", line 1, in <module>
StopIteration
Note that generator expressions are much like list comprehensions:
>>> lc = [n for n in range(3, 5)]
>>> lc
[3, 4]
Observe that a generator object is generated once, but its code is not run all at once. Only calls to next
actually execute (part of) the code. Execution of the code in a generator stops once a yield
statement has been reached, upon which it returns a value. The next call to next
then causes execution to continue in the state in which the generator was left after the last yield
. This is a fundamental difference with regular functions: those always start execution at the "top" and discard their state upon returning a value.
There are more things to be said about this subject. It is e.g. possible to send
data back into a generator (reference). But that is something I suggest you do not look into until you understand the basic concept of a generator.
Now you may ask: why use generators? There are a couple of good reasons:
- Certain concepts can be described much more succinctly using generators.
- Instead of creating a function which returns a list of values, one can write a generator which generates the values on the fly. This means that no list needs to be constructed, meaning that the resulting code is more memory efficient. In this way one can even describe data streams which would simply be too large to fit in memory.
Generators allow for a natural way to describe infinite streams. Consider for example the Fibonacci numbers:
>>> def fib():
... a, b = 0, 1
... while True:
... yield a
... a, b = b, a + b
...
>>> import itertools
>>> list(itertools.islice(fib(), 10))
[0, 1, 1, 2, 3, 5, 8, 13, 21, 34]This code uses
itertools.islice
to take a finite number of elements from an infinite stream. You are advised to have a good look at the functions in theitertools
module, as they are essential tools for writing advanced generators with great ease.
†About Python <=2.6: in the above examples next
is a function which calls the method __next__
on the given object. In Python <=2.6 one uses a slightly different technique, namely o.next()
instead of next(o)
. Python 2.7 has next()
call .next
so you need not use the following in 2.7:
>>> g = (n for n in range(3, 5))
>>> g.next()
3
edited Jun 7 '16 at 2:33
Community♦
11
11
answered Nov 18 '09 at 13:54
Stephan202Stephan202
47.9k8108124
47.9k8108124
7
You mention it is possible tosend
data to a generator. Once you do that you have a 'coroutine'. It's very simple to implement patterns like the mentioned Consumer/Producer with coroutines because they have no need forLock
s and therefore can't deadlock. It's hard to describe coroutines without bashing threads, so I'll just say coroutines are a very elegant alternative to threading.
– Jochen Ritzel
Nov 18 '09 at 14:47
Are Python generators basically Turing machines in terms of how they function?
– Fiery Phoenix
Sep 23 '16 at 23:34
Thank you for the references and mentioning itertools for usage of generators.
– pyeR_biz
Aug 3 '18 at 1:13
add a comment |
7
You mention it is possible tosend
data to a generator. Once you do that you have a 'coroutine'. It's very simple to implement patterns like the mentioned Consumer/Producer with coroutines because they have no need forLock
s and therefore can't deadlock. It's hard to describe coroutines without bashing threads, so I'll just say coroutines are a very elegant alternative to threading.
– Jochen Ritzel
Nov 18 '09 at 14:47
Are Python generators basically Turing machines in terms of how they function?
– Fiery Phoenix
Sep 23 '16 at 23:34
Thank you for the references and mentioning itertools for usage of generators.
– pyeR_biz
Aug 3 '18 at 1:13
7
7
You mention it is possible to
send
data to a generator. Once you do that you have a 'coroutine'. It's very simple to implement patterns like the mentioned Consumer/Producer with coroutines because they have no need for Lock
s and therefore can't deadlock. It's hard to describe coroutines without bashing threads, so I'll just say coroutines are a very elegant alternative to threading.– Jochen Ritzel
Nov 18 '09 at 14:47
You mention it is possible to
send
data to a generator. Once you do that you have a 'coroutine'. It's very simple to implement patterns like the mentioned Consumer/Producer with coroutines because they have no need for Lock
s and therefore can't deadlock. It's hard to describe coroutines without bashing threads, so I'll just say coroutines are a very elegant alternative to threading.– Jochen Ritzel
Nov 18 '09 at 14:47
Are Python generators basically Turing machines in terms of how they function?
– Fiery Phoenix
Sep 23 '16 at 23:34
Are Python generators basically Turing machines in terms of how they function?
– Fiery Phoenix
Sep 23 '16 at 23:34
Thank you for the references and mentioning itertools for usage of generators.
– pyeR_biz
Aug 3 '18 at 1:13
Thank you for the references and mentioning itertools for usage of generators.
– pyeR_biz
Aug 3 '18 at 1:13
add a comment |
A generator is effectively a function that returns (data) before it is finished, but it pauses at that point, and you can resume the function at that point.
>>> def myGenerator():
... yield 'These'
... yield 'words'
... yield 'come'
... yield 'one'
... yield 'at'
... yield 'a'
... yield 'time'
>>> myGeneratorInstance = myGenerator()
>>> next(myGeneratorInstance)
These
>>> next(myGeneratorInstance)
words
and so on. The (or one) benefit of generators is that because they deal with data one piece at a time, you can deal with large amounts of data; with lists, excessive memory requirements could become a problem. Generators, just like lists, are iterable, so they can be used in the same ways:
>>> for word in myGeneratorInstance:
... print word
These
words
come
one
at
a
time
Note that generators provide another way to deal with infinity, for example
>>> from time import gmtime, strftime
>>> def myGen():
... while True:
... yield strftime("%a, %d %b %Y %H:%M:%S +0000", gmtime())
>>> myGeneratorInstance = myGen()
>>> next(myGeneratorInstance)
Thu, 28 Jun 2001 14:17:15 +0000
>>> next(myGeneratorInstance)
Thu, 28 Jun 2001 14:18:02 +0000
The generator encapsulates an infinite loop, but this isn't a problem because you only get each answer every time you ask for it.
add a comment |
A generator is effectively a function that returns (data) before it is finished, but it pauses at that point, and you can resume the function at that point.
>>> def myGenerator():
... yield 'These'
... yield 'words'
... yield 'come'
... yield 'one'
... yield 'at'
... yield 'a'
... yield 'time'
>>> myGeneratorInstance = myGenerator()
>>> next(myGeneratorInstance)
These
>>> next(myGeneratorInstance)
words
and so on. The (or one) benefit of generators is that because they deal with data one piece at a time, you can deal with large amounts of data; with lists, excessive memory requirements could become a problem. Generators, just like lists, are iterable, so they can be used in the same ways:
>>> for word in myGeneratorInstance:
... print word
These
words
come
one
at
a
time
Note that generators provide another way to deal with infinity, for example
>>> from time import gmtime, strftime
>>> def myGen():
... while True:
... yield strftime("%a, %d %b %Y %H:%M:%S +0000", gmtime())
>>> myGeneratorInstance = myGen()
>>> next(myGeneratorInstance)
Thu, 28 Jun 2001 14:17:15 +0000
>>> next(myGeneratorInstance)
Thu, 28 Jun 2001 14:18:02 +0000
The generator encapsulates an infinite loop, but this isn't a problem because you only get each answer every time you ask for it.
add a comment |
A generator is effectively a function that returns (data) before it is finished, but it pauses at that point, and you can resume the function at that point.
>>> def myGenerator():
... yield 'These'
... yield 'words'
... yield 'come'
... yield 'one'
... yield 'at'
... yield 'a'
... yield 'time'
>>> myGeneratorInstance = myGenerator()
>>> next(myGeneratorInstance)
These
>>> next(myGeneratorInstance)
words
and so on. The (or one) benefit of generators is that because they deal with data one piece at a time, you can deal with large amounts of data; with lists, excessive memory requirements could become a problem. Generators, just like lists, are iterable, so they can be used in the same ways:
>>> for word in myGeneratorInstance:
... print word
These
words
come
one
at
a
time
Note that generators provide another way to deal with infinity, for example
>>> from time import gmtime, strftime
>>> def myGen():
... while True:
... yield strftime("%a, %d %b %Y %H:%M:%S +0000", gmtime())
>>> myGeneratorInstance = myGen()
>>> next(myGeneratorInstance)
Thu, 28 Jun 2001 14:17:15 +0000
>>> next(myGeneratorInstance)
Thu, 28 Jun 2001 14:18:02 +0000
The generator encapsulates an infinite loop, but this isn't a problem because you only get each answer every time you ask for it.
A generator is effectively a function that returns (data) before it is finished, but it pauses at that point, and you can resume the function at that point.
>>> def myGenerator():
... yield 'These'
... yield 'words'
... yield 'come'
... yield 'one'
... yield 'at'
... yield 'a'
... yield 'time'
>>> myGeneratorInstance = myGenerator()
>>> next(myGeneratorInstance)
These
>>> next(myGeneratorInstance)
words
and so on. The (or one) benefit of generators is that because they deal with data one piece at a time, you can deal with large amounts of data; with lists, excessive memory requirements could become a problem. Generators, just like lists, are iterable, so they can be used in the same ways:
>>> for word in myGeneratorInstance:
... print word
These
words
come
one
at
a
time
Note that generators provide another way to deal with infinity, for example
>>> from time import gmtime, strftime
>>> def myGen():
... while True:
... yield strftime("%a, %d %b %Y %H:%M:%S +0000", gmtime())
>>> myGeneratorInstance = myGen()
>>> next(myGeneratorInstance)
Thu, 28 Jun 2001 14:17:15 +0000
>>> next(myGeneratorInstance)
Thu, 28 Jun 2001 14:18:02 +0000
The generator encapsulates an infinite loop, but this isn't a problem because you only get each answer every time you ask for it.
edited Aug 26 '16 at 14:21
Community♦
11
11
answered Nov 18 '09 at 14:24
Caleb HattinghCaleb Hattingh
7,46312540
7,46312540
add a comment |
add a comment |
First of all, the term generator originally was somewhat ill-defined in Python, leading to lots of confusion. You probably mean iterators and iterables (see here). Then in Python there are also generator functions (which return a generator object), generator objects (which are iterators) and generator expressions (which are evaluated to a generator object).
According to the glossary entry for generator it seems that the official terminology is now that generator is short for "generator function". In the past the documentation defined the terms inconsistently, but fortunately this has been fixed.
It might still be a good idea to be precise and avoid the term "generator" without further specification.
2
Hmm I think you're right, at least according to a test of a few lines in Python 2.6. A generator expression returns an iterator (aka 'generator object'), not a generator.
– Craig McQueen
Dec 4 '09 at 1:34
add a comment |
First of all, the term generator originally was somewhat ill-defined in Python, leading to lots of confusion. You probably mean iterators and iterables (see here). Then in Python there are also generator functions (which return a generator object), generator objects (which are iterators) and generator expressions (which are evaluated to a generator object).
According to the glossary entry for generator it seems that the official terminology is now that generator is short for "generator function". In the past the documentation defined the terms inconsistently, but fortunately this has been fixed.
It might still be a good idea to be precise and avoid the term "generator" without further specification.
2
Hmm I think you're right, at least according to a test of a few lines in Python 2.6. A generator expression returns an iterator (aka 'generator object'), not a generator.
– Craig McQueen
Dec 4 '09 at 1:34
add a comment |
First of all, the term generator originally was somewhat ill-defined in Python, leading to lots of confusion. You probably mean iterators and iterables (see here). Then in Python there are also generator functions (which return a generator object), generator objects (which are iterators) and generator expressions (which are evaluated to a generator object).
According to the glossary entry for generator it seems that the official terminology is now that generator is short for "generator function". In the past the documentation defined the terms inconsistently, but fortunately this has been fixed.
It might still be a good idea to be precise and avoid the term "generator" without further specification.
First of all, the term generator originally was somewhat ill-defined in Python, leading to lots of confusion. You probably mean iterators and iterables (see here). Then in Python there are also generator functions (which return a generator object), generator objects (which are iterators) and generator expressions (which are evaluated to a generator object).
According to the glossary entry for generator it seems that the official terminology is now that generator is short for "generator function". In the past the documentation defined the terms inconsistently, but fortunately this has been fixed.
It might still be a good idea to be precise and avoid the term "generator" without further specification.
edited Jun 15 '18 at 5:04


Peter Mortensen
13.8k1987113
13.8k1987113
answered Nov 18 '09 at 14:35
nikownikow
17k63666
17k63666
2
Hmm I think you're right, at least according to a test of a few lines in Python 2.6. A generator expression returns an iterator (aka 'generator object'), not a generator.
– Craig McQueen
Dec 4 '09 at 1:34
add a comment |
2
Hmm I think you're right, at least according to a test of a few lines in Python 2.6. A generator expression returns an iterator (aka 'generator object'), not a generator.
– Craig McQueen
Dec 4 '09 at 1:34
2
2
Hmm I think you're right, at least according to a test of a few lines in Python 2.6. A generator expression returns an iterator (aka 'generator object'), not a generator.
– Craig McQueen
Dec 4 '09 at 1:34
Hmm I think you're right, at least according to a test of a few lines in Python 2.6. A generator expression returns an iterator (aka 'generator object'), not a generator.
– Craig McQueen
Dec 4 '09 at 1:34
add a comment |
Generators could be thought of as shorthand for creating an iterator. They behave like a Java Iterator. Example:
>>> g = (x for x in range(10))
>>> g
<generator object <genexpr> at 0x7fac1c1e6aa0>
>>> g.next()
0
>>> g.next()
1
>>> g.next()
2
>>> list(g) # force iterating the rest
[3, 4, 5, 6, 7, 8, 9]
>>> g.next() # iterator is at the end; calling next again will throw
Traceback (most recent call last):
File "<stdin>", line 1, in <module>
StopIteration
Hope this helps/is what you are looking for.
Update:
As many other answers are showing, there are different ways to create a generator. You can use the parentheses syntax as in my example above, or you can use yield. Another interesting feature is that generators can be "infinite" -- iterators that don't stop:
>>> def infinite_gen():
... n = 0
... while True:
... yield n
... n = n + 1
...
>>> g = infinite_gen()
>>> g.next()
0
>>> g.next()
1
>>> g.next()
2
>>> g.next()
3
...
Now, Java hasStream
s, which are far more similar to generators, except that you apparently can't just get the next element without a surprising amount of hassle.
– Nic Hartley
Apr 29 '16 at 20:36
add a comment |
Generators could be thought of as shorthand for creating an iterator. They behave like a Java Iterator. Example:
>>> g = (x for x in range(10))
>>> g
<generator object <genexpr> at 0x7fac1c1e6aa0>
>>> g.next()
0
>>> g.next()
1
>>> g.next()
2
>>> list(g) # force iterating the rest
[3, 4, 5, 6, 7, 8, 9]
>>> g.next() # iterator is at the end; calling next again will throw
Traceback (most recent call last):
File "<stdin>", line 1, in <module>
StopIteration
Hope this helps/is what you are looking for.
Update:
As many other answers are showing, there are different ways to create a generator. You can use the parentheses syntax as in my example above, or you can use yield. Another interesting feature is that generators can be "infinite" -- iterators that don't stop:
>>> def infinite_gen():
... n = 0
... while True:
... yield n
... n = n + 1
...
>>> g = infinite_gen()
>>> g.next()
0
>>> g.next()
1
>>> g.next()
2
>>> g.next()
3
...
Now, Java hasStream
s, which are far more similar to generators, except that you apparently can't just get the next element without a surprising amount of hassle.
– Nic Hartley
Apr 29 '16 at 20:36
add a comment |
Generators could be thought of as shorthand for creating an iterator. They behave like a Java Iterator. Example:
>>> g = (x for x in range(10))
>>> g
<generator object <genexpr> at 0x7fac1c1e6aa0>
>>> g.next()
0
>>> g.next()
1
>>> g.next()
2
>>> list(g) # force iterating the rest
[3, 4, 5, 6, 7, 8, 9]
>>> g.next() # iterator is at the end; calling next again will throw
Traceback (most recent call last):
File "<stdin>", line 1, in <module>
StopIteration
Hope this helps/is what you are looking for.
Update:
As many other answers are showing, there are different ways to create a generator. You can use the parentheses syntax as in my example above, or you can use yield. Another interesting feature is that generators can be "infinite" -- iterators that don't stop:
>>> def infinite_gen():
... n = 0
... while True:
... yield n
... n = n + 1
...
>>> g = infinite_gen()
>>> g.next()
0
>>> g.next()
1
>>> g.next()
2
>>> g.next()
3
...
Generators could be thought of as shorthand for creating an iterator. They behave like a Java Iterator. Example:
>>> g = (x for x in range(10))
>>> g
<generator object <genexpr> at 0x7fac1c1e6aa0>
>>> g.next()
0
>>> g.next()
1
>>> g.next()
2
>>> list(g) # force iterating the rest
[3, 4, 5, 6, 7, 8, 9]
>>> g.next() # iterator is at the end; calling next again will throw
Traceback (most recent call last):
File "<stdin>", line 1, in <module>
StopIteration
Hope this helps/is what you are looking for.
Update:
As many other answers are showing, there are different ways to create a generator. You can use the parentheses syntax as in my example above, or you can use yield. Another interesting feature is that generators can be "infinite" -- iterators that don't stop:
>>> def infinite_gen():
... n = 0
... while True:
... yield n
... n = n + 1
...
>>> g = infinite_gen()
>>> g.next()
0
>>> g.next()
1
>>> g.next()
2
>>> g.next()
3
...
edited Nov 18 '09 at 14:15
answered Nov 18 '09 at 13:53
overthinkoverthink
20k35665
20k35665
Now, Java hasStream
s, which are far more similar to generators, except that you apparently can't just get the next element without a surprising amount of hassle.
– Nic Hartley
Apr 29 '16 at 20:36
add a comment |
Now, Java hasStream
s, which are far more similar to generators, except that you apparently can't just get the next element without a surprising amount of hassle.
– Nic Hartley
Apr 29 '16 at 20:36
Now, Java has
Stream
s, which are far more similar to generators, except that you apparently can't just get the next element without a surprising amount of hassle.– Nic Hartley
Apr 29 '16 at 20:36
Now, Java has
Stream
s, which are far more similar to generators, except that you apparently can't just get the next element without a surprising amount of hassle.– Nic Hartley
Apr 29 '16 at 20:36
add a comment |
There is no Java equivalent.
Here is a bit of a contrived example:
#! /usr/bin/python
def mygen(n):
x = 0
while x < n:
x = x + 1
if x % 3 == 0:
yield x
for a in mygen(100):
print a
There is a loop in the generator that runs from 0 to n, and if the loop variable is a multiple of 3, it yields the variable.
During each iteration of the for
loop the generator is executed. If it is the first time the generator executes, it starts at the beginning, otherwise it continues from the previous time it yielded.
2
The last paragraph is very important: The state of the generator function is 'frozen' everytime it yields sth, and continues in exactly the same state when it is invoked the next time.
– Johannes Charra
Nov 18 '09 at 14:12
There's no syntactic equivalent in Java to a "generator expression", but generators -- once you've got one -- are essentially just an iterator (same basic characteristics as a Java iterator).
– overthink
Nov 18 '09 at 14:21
@overthink: Well, generators can have other side effects that Java iterators can't have. If I were to putprint "hello"
after thex=x+1
in my example, "hello" would be printed 100 times, while the body of the for loop would still only be executed 33 times.
– Wernsey
Nov 18 '09 at 15:02
@iWerner: Pretty sure the same effect could be had in Java. The implementation of next() in the equivalent Java iterator would still have to search from 0 to 99 (using your mygen(100) example), so you could System.out.println() each time if you wanted. You'd only return 33 times from next() though. What Java lacks is the very handy yield syntax which is significantly easier to read (and write).
– overthink
Nov 18 '09 at 15:54
add a comment |
There is no Java equivalent.
Here is a bit of a contrived example:
#! /usr/bin/python
def mygen(n):
x = 0
while x < n:
x = x + 1
if x % 3 == 0:
yield x
for a in mygen(100):
print a
There is a loop in the generator that runs from 0 to n, and if the loop variable is a multiple of 3, it yields the variable.
During each iteration of the for
loop the generator is executed. If it is the first time the generator executes, it starts at the beginning, otherwise it continues from the previous time it yielded.
2
The last paragraph is very important: The state of the generator function is 'frozen' everytime it yields sth, and continues in exactly the same state when it is invoked the next time.
– Johannes Charra
Nov 18 '09 at 14:12
There's no syntactic equivalent in Java to a "generator expression", but generators -- once you've got one -- are essentially just an iterator (same basic characteristics as a Java iterator).
– overthink
Nov 18 '09 at 14:21
@overthink: Well, generators can have other side effects that Java iterators can't have. If I were to putprint "hello"
after thex=x+1
in my example, "hello" would be printed 100 times, while the body of the for loop would still only be executed 33 times.
– Wernsey
Nov 18 '09 at 15:02
@iWerner: Pretty sure the same effect could be had in Java. The implementation of next() in the equivalent Java iterator would still have to search from 0 to 99 (using your mygen(100) example), so you could System.out.println() each time if you wanted. You'd only return 33 times from next() though. What Java lacks is the very handy yield syntax which is significantly easier to read (and write).
– overthink
Nov 18 '09 at 15:54
add a comment |
There is no Java equivalent.
Here is a bit of a contrived example:
#! /usr/bin/python
def mygen(n):
x = 0
while x < n:
x = x + 1
if x % 3 == 0:
yield x
for a in mygen(100):
print a
There is a loop in the generator that runs from 0 to n, and if the loop variable is a multiple of 3, it yields the variable.
During each iteration of the for
loop the generator is executed. If it is the first time the generator executes, it starts at the beginning, otherwise it continues from the previous time it yielded.
There is no Java equivalent.
Here is a bit of a contrived example:
#! /usr/bin/python
def mygen(n):
x = 0
while x < n:
x = x + 1
if x % 3 == 0:
yield x
for a in mygen(100):
print a
There is a loop in the generator that runs from 0 to n, and if the loop variable is a multiple of 3, it yields the variable.
During each iteration of the for
loop the generator is executed. If it is the first time the generator executes, it starts at the beginning, otherwise it continues from the previous time it yielded.
edited May 20 '18 at 9:02


Peter Mortensen
13.8k1987113
13.8k1987113
answered Nov 18 '09 at 13:58


WernseyWernsey
4,7791635
4,7791635
2
The last paragraph is very important: The state of the generator function is 'frozen' everytime it yields sth, and continues in exactly the same state when it is invoked the next time.
– Johannes Charra
Nov 18 '09 at 14:12
There's no syntactic equivalent in Java to a "generator expression", but generators -- once you've got one -- are essentially just an iterator (same basic characteristics as a Java iterator).
– overthink
Nov 18 '09 at 14:21
@overthink: Well, generators can have other side effects that Java iterators can't have. If I were to putprint "hello"
after thex=x+1
in my example, "hello" would be printed 100 times, while the body of the for loop would still only be executed 33 times.
– Wernsey
Nov 18 '09 at 15:02
@iWerner: Pretty sure the same effect could be had in Java. The implementation of next() in the equivalent Java iterator would still have to search from 0 to 99 (using your mygen(100) example), so you could System.out.println() each time if you wanted. You'd only return 33 times from next() though. What Java lacks is the very handy yield syntax which is significantly easier to read (and write).
– overthink
Nov 18 '09 at 15:54
add a comment |
2
The last paragraph is very important: The state of the generator function is 'frozen' everytime it yields sth, and continues in exactly the same state when it is invoked the next time.
– Johannes Charra
Nov 18 '09 at 14:12
There's no syntactic equivalent in Java to a "generator expression", but generators -- once you've got one -- are essentially just an iterator (same basic characteristics as a Java iterator).
– overthink
Nov 18 '09 at 14:21
@overthink: Well, generators can have other side effects that Java iterators can't have. If I were to putprint "hello"
after thex=x+1
in my example, "hello" would be printed 100 times, while the body of the for loop would still only be executed 33 times.
– Wernsey
Nov 18 '09 at 15:02
@iWerner: Pretty sure the same effect could be had in Java. The implementation of next() in the equivalent Java iterator would still have to search from 0 to 99 (using your mygen(100) example), so you could System.out.println() each time if you wanted. You'd only return 33 times from next() though. What Java lacks is the very handy yield syntax which is significantly easier to read (and write).
– overthink
Nov 18 '09 at 15:54
2
2
The last paragraph is very important: The state of the generator function is 'frozen' everytime it yields sth, and continues in exactly the same state when it is invoked the next time.
– Johannes Charra
Nov 18 '09 at 14:12
The last paragraph is very important: The state of the generator function is 'frozen' everytime it yields sth, and continues in exactly the same state when it is invoked the next time.
– Johannes Charra
Nov 18 '09 at 14:12
There's no syntactic equivalent in Java to a "generator expression", but generators -- once you've got one -- are essentially just an iterator (same basic characteristics as a Java iterator).
– overthink
Nov 18 '09 at 14:21
There's no syntactic equivalent in Java to a "generator expression", but generators -- once you've got one -- are essentially just an iterator (same basic characteristics as a Java iterator).
– overthink
Nov 18 '09 at 14:21
@overthink: Well, generators can have other side effects that Java iterators can't have. If I were to put
print "hello"
after the x=x+1
in my example, "hello" would be printed 100 times, while the body of the for loop would still only be executed 33 times.– Wernsey
Nov 18 '09 at 15:02
@overthink: Well, generators can have other side effects that Java iterators can't have. If I were to put
print "hello"
after the x=x+1
in my example, "hello" would be printed 100 times, while the body of the for loop would still only be executed 33 times.– Wernsey
Nov 18 '09 at 15:02
@iWerner: Pretty sure the same effect could be had in Java. The implementation of next() in the equivalent Java iterator would still have to search from 0 to 99 (using your mygen(100) example), so you could System.out.println() each time if you wanted. You'd only return 33 times from next() though. What Java lacks is the very handy yield syntax which is significantly easier to read (and write).
– overthink
Nov 18 '09 at 15:54
@iWerner: Pretty sure the same effect could be had in Java. The implementation of next() in the equivalent Java iterator would still have to search from 0 to 99 (using your mygen(100) example), so you could System.out.println() each time if you wanted. You'd only return 33 times from next() though. What Java lacks is the very handy yield syntax which is significantly easier to read (and write).
– overthink
Nov 18 '09 at 15:54
add a comment |
I like to describe generators, to those with a decent background in programming languages and computing, in terms of stack frames.
In many languages, there is a stack on top of which is the current stack "frame". The stack frame includes space allocated for variables local to the function including the arguments passed in to that function.
When you call a function, the current point of execution (the "program counter" or equivalent) is pushed onto the stack, and a new stack frame is created. Execution then transfers to the beginning of the function being called.
With regular functions, at some point the function returns a value, and the stack is "popped". The function's stack frame is discarded and execution resumes at the previous location.
When a function is a generator, it can return a value without the stack frame being discarded, using the yield statement. The values of local variables and the program counter within the function are preserved. This allows the generator to be resumed at a later time, with execution continuing from the yield statement, and it can execute more code and return another value.
Before Python 2.5 this was all generators did. Python 2.5 added the ability to pass values back in to the generator as well. In doing so, the passed-in value is available as an expression resulting from the yield statement which had temporarily returned control (and a value) from the generator.
The key advantage to generators is that the "state" of the function is preserved, unlike with regular functions where each time the stack frame is discarded, you lose all that "state". A secondary advantage is that some of the function call overhead (creating and deleting stack frames) is avoided, though this is a usually a minor advantage.
add a comment |
I like to describe generators, to those with a decent background in programming languages and computing, in terms of stack frames.
In many languages, there is a stack on top of which is the current stack "frame". The stack frame includes space allocated for variables local to the function including the arguments passed in to that function.
When you call a function, the current point of execution (the "program counter" or equivalent) is pushed onto the stack, and a new stack frame is created. Execution then transfers to the beginning of the function being called.
With regular functions, at some point the function returns a value, and the stack is "popped". The function's stack frame is discarded and execution resumes at the previous location.
When a function is a generator, it can return a value without the stack frame being discarded, using the yield statement. The values of local variables and the program counter within the function are preserved. This allows the generator to be resumed at a later time, with execution continuing from the yield statement, and it can execute more code and return another value.
Before Python 2.5 this was all generators did. Python 2.5 added the ability to pass values back in to the generator as well. In doing so, the passed-in value is available as an expression resulting from the yield statement which had temporarily returned control (and a value) from the generator.
The key advantage to generators is that the "state" of the function is preserved, unlike with regular functions where each time the stack frame is discarded, you lose all that "state". A secondary advantage is that some of the function call overhead (creating and deleting stack frames) is avoided, though this is a usually a minor advantage.
add a comment |
I like to describe generators, to those with a decent background in programming languages and computing, in terms of stack frames.
In many languages, there is a stack on top of which is the current stack "frame". The stack frame includes space allocated for variables local to the function including the arguments passed in to that function.
When you call a function, the current point of execution (the "program counter" or equivalent) is pushed onto the stack, and a new stack frame is created. Execution then transfers to the beginning of the function being called.
With regular functions, at some point the function returns a value, and the stack is "popped". The function's stack frame is discarded and execution resumes at the previous location.
When a function is a generator, it can return a value without the stack frame being discarded, using the yield statement. The values of local variables and the program counter within the function are preserved. This allows the generator to be resumed at a later time, with execution continuing from the yield statement, and it can execute more code and return another value.
Before Python 2.5 this was all generators did. Python 2.5 added the ability to pass values back in to the generator as well. In doing so, the passed-in value is available as an expression resulting from the yield statement which had temporarily returned control (and a value) from the generator.
The key advantage to generators is that the "state" of the function is preserved, unlike with regular functions where each time the stack frame is discarded, you lose all that "state". A secondary advantage is that some of the function call overhead (creating and deleting stack frames) is avoided, though this is a usually a minor advantage.
I like to describe generators, to those with a decent background in programming languages and computing, in terms of stack frames.
In many languages, there is a stack on top of which is the current stack "frame". The stack frame includes space allocated for variables local to the function including the arguments passed in to that function.
When you call a function, the current point of execution (the "program counter" or equivalent) is pushed onto the stack, and a new stack frame is created. Execution then transfers to the beginning of the function being called.
With regular functions, at some point the function returns a value, and the stack is "popped". The function's stack frame is discarded and execution resumes at the previous location.
When a function is a generator, it can return a value without the stack frame being discarded, using the yield statement. The values of local variables and the program counter within the function are preserved. This allows the generator to be resumed at a later time, with execution continuing from the yield statement, and it can execute more code and return another value.
Before Python 2.5 this was all generators did. Python 2.5 added the ability to pass values back in to the generator as well. In doing so, the passed-in value is available as an expression resulting from the yield statement which had temporarily returned control (and a value) from the generator.
The key advantage to generators is that the "state" of the function is preserved, unlike with regular functions where each time the stack frame is discarded, you lose all that "state". A secondary advantage is that some of the function call overhead (creating and deleting stack frames) is avoided, though this is a usually a minor advantage.
answered Dec 19 '09 at 10:50
Peter HansenPeter Hansen
15.2k24066
15.2k24066
add a comment |
add a comment |
The only thing I can add to Stephan202's answer is a recommendation that you take a look at David Beazley's PyCon '08 presentation "Generator Tricks for Systems Programmers," which is the best single explanation of the how and why of generators that I've seen anywhere. This is the thing that took me from "Python looks kind of fun" to "This is what I've been looking for." It's at http://www.dabeaz.com/generators/.
add a comment |
The only thing I can add to Stephan202's answer is a recommendation that you take a look at David Beazley's PyCon '08 presentation "Generator Tricks for Systems Programmers," which is the best single explanation of the how and why of generators that I've seen anywhere. This is the thing that took me from "Python looks kind of fun" to "This is what I've been looking for." It's at http://www.dabeaz.com/generators/.
add a comment |
The only thing I can add to Stephan202's answer is a recommendation that you take a look at David Beazley's PyCon '08 presentation "Generator Tricks for Systems Programmers," which is the best single explanation of the how and why of generators that I've seen anywhere. This is the thing that took me from "Python looks kind of fun" to "This is what I've been looking for." It's at http://www.dabeaz.com/generators/.
The only thing I can add to Stephan202's answer is a recommendation that you take a look at David Beazley's PyCon '08 presentation "Generator Tricks for Systems Programmers," which is the best single explanation of the how and why of generators that I've seen anywhere. This is the thing that took me from "Python looks kind of fun" to "This is what I've been looking for." It's at http://www.dabeaz.com/generators/.
answered Nov 18 '09 at 17:54
Robert RossneyRobert Rossney
72.8k23121201
72.8k23121201
add a comment |
add a comment |
It helps to make a clear distinction between the function foo, and the generator foo(n):
def foo(n):
yield n
yield n+1
foo is a function.
foo(6) is a generator object.
The typical way to use a generator object is in a loop:
for n in foo(6):
print(n)
The loop prints
# 6
# 7
Think of a generator as a resumable function.
yield
behaves like return
in the sense that values that are yielded get "returned" by the generator. Unlike return, however, the next time the generator gets asked for a value, the generator's function, foo, resumes where it left off -- after the last yield statement -- and continues to run until it hits another yield statement.
Behind the scenes, when you call bar=foo(6)
the generator object bar is defined for you to have a next
attribute.
You can call it yourself to retrieve values yielded from foo:
next(bar) # Works in Python 2.6 or Python 3.x
bar.next() # Works in Python 2.5+, but is deprecated. Use next() if possible.
When foo ends (and there are no more yielded values), calling next(bar)
throws a StopInteration error.
add a comment |
It helps to make a clear distinction between the function foo, and the generator foo(n):
def foo(n):
yield n
yield n+1
foo is a function.
foo(6) is a generator object.
The typical way to use a generator object is in a loop:
for n in foo(6):
print(n)
The loop prints
# 6
# 7
Think of a generator as a resumable function.
yield
behaves like return
in the sense that values that are yielded get "returned" by the generator. Unlike return, however, the next time the generator gets asked for a value, the generator's function, foo, resumes where it left off -- after the last yield statement -- and continues to run until it hits another yield statement.
Behind the scenes, when you call bar=foo(6)
the generator object bar is defined for you to have a next
attribute.
You can call it yourself to retrieve values yielded from foo:
next(bar) # Works in Python 2.6 or Python 3.x
bar.next() # Works in Python 2.5+, but is deprecated. Use next() if possible.
When foo ends (and there are no more yielded values), calling next(bar)
throws a StopInteration error.
add a comment |
It helps to make a clear distinction between the function foo, and the generator foo(n):
def foo(n):
yield n
yield n+1
foo is a function.
foo(6) is a generator object.
The typical way to use a generator object is in a loop:
for n in foo(6):
print(n)
The loop prints
# 6
# 7
Think of a generator as a resumable function.
yield
behaves like return
in the sense that values that are yielded get "returned" by the generator. Unlike return, however, the next time the generator gets asked for a value, the generator's function, foo, resumes where it left off -- after the last yield statement -- and continues to run until it hits another yield statement.
Behind the scenes, when you call bar=foo(6)
the generator object bar is defined for you to have a next
attribute.
You can call it yourself to retrieve values yielded from foo:
next(bar) # Works in Python 2.6 or Python 3.x
bar.next() # Works in Python 2.5+, but is deprecated. Use next() if possible.
When foo ends (and there are no more yielded values), calling next(bar)
throws a StopInteration error.
It helps to make a clear distinction between the function foo, and the generator foo(n):
def foo(n):
yield n
yield n+1
foo is a function.
foo(6) is a generator object.
The typical way to use a generator object is in a loop:
for n in foo(6):
print(n)
The loop prints
# 6
# 7
Think of a generator as a resumable function.
yield
behaves like return
in the sense that values that are yielded get "returned" by the generator. Unlike return, however, the next time the generator gets asked for a value, the generator's function, foo, resumes where it left off -- after the last yield statement -- and continues to run until it hits another yield statement.
Behind the scenes, when you call bar=foo(6)
the generator object bar is defined for you to have a next
attribute.
You can call it yourself to retrieve values yielded from foo:
next(bar) # Works in Python 2.6 or Python 3.x
bar.next() # Works in Python 2.5+, but is deprecated. Use next() if possible.
When foo ends (and there are no more yielded values), calling next(bar)
throws a StopInteration error.
edited May 20 '18 at 9:04


Peter Mortensen
13.8k1987113
13.8k1987113
answered Nov 18 '09 at 14:15
unutbuunutbu
558k10511991255
558k10511991255
add a comment |
add a comment |
This post will use Fibonacci numbers as a tool to build up to explaining the usefulness of Python generators.
This post will feature both C++ and Python code.
Fibonacci numbers are defined as the sequence: 0, 1, 1, 2, 3, 5, 8, 13, 21, 34, ....
Or in general:
F0 = 0
F1 = 1
Fn = Fn-1 + Fn-2
This can be transferred into a C++ function extremely easily:
size_t Fib(size_t n)
//Fib(0) = 0
if(n == 0)
return 0;
//Fib(1) = 1
if(n == 1)
return 1;
//Fib(N) = Fib(N-2) + Fib(N-1)
return Fib(n-2) + Fib(n-1);
But if you want to print the first six Fibonacci numbers, you will be recalculating a lot of the values with the above function.
For example: Fib(3) = Fib(2) + Fib(1)
, but Fib(2)
also recalculates Fib(1)
. The higher the value you want to calculate, the worse off you will be.
So one may be tempted to rewrite the above by keeping track of the state in main
.
// Not supported for the first two elements of Fib
size_t GetNextFib(size_t &pp, size_t &p)
int result = pp + p;
pp = p;
p = result;
return result;
int main(int argc, char *argv[])
size_t pp = 0;
size_t p = 1;
std::cout << "0 " << "1 ";
for(size_t i = 0; i <= 4; ++i)
size_t fibI = GetNextFib(pp, p);
std::cout << fibI << " ";
return 0;
But this is very ugly, and it complicates our logic in main
. It would be better to not have to worry about state in our main
function.
We could return a vector
of values and use an iterator
to iterate over that set of values, but this requires a lot of memory all at once for a large number of return values.
So back to our old approach, what happens if we wanted to do something else besides print the numbers? We'd have to copy and paste the whole block of code in main
and change the output statements to whatever else we wanted to do.
And if you copy and paste code, then you should be shot. You don't want to get shot, do you?
To solve these problems, and to avoid getting shot, we may rewrite this block of code using a callback function. Every time a new Fibonacci number is encountered, we would call the callback function.
void GetFibNumbers(size_t max, void(*FoundNewFibCallback)(size_t))
if(max-- == 0) return;
FoundNewFibCallback(0);
if(max-- == 0) return;
FoundNewFibCallback(1);
size_t pp = 0;
size_t p = 1;
for(;;)
if(max-- == 0) return;
int result = pp + p;
pp = p;
p = result;
FoundNewFibCallback(result);
void foundNewFib(size_t fibI)
std::cout << fibI << " ";
int main(int argc, char *argv[])
GetFibNumbers(6, foundNewFib);
return 0;
This is clearly an improvement, your logic in main
is not as cluttered, and you can do anything you want with the Fibonacci numbers, simply define new callbacks.
But this is still not perfect. What if you wanted to only get the first two Fibonacci numbers, and then do something, then get some more, then do something else?
Well, we could go on like we have been, and we could start adding state again into main
, allowing GetFibNumbers to start from an arbitrary point.
But this will further bloat our code, and it already looks too big for a simple task like printing Fibonacci numbers.
We could implement a producer and consumer model via a couple of threads. But this complicates the code even more.
Instead let's talk about generators.
Python has a very nice language feature that solves problems like these called generators.
A generator allows you to execute a function, stop at an arbitrary point, and then continue again where you left off.
Each time returning a value.
Consider the following code that uses a generator:
def fib():
pp, p = 0, 1
while 1:
yield pp
pp, p = p, pp+p
g = fib()
for i in range(6):
g.next()
Which gives us the results:
0
1
1
2
3
5
The yield
statement is used in conjuction with Python generators. It saves the state of the function and returns the yeilded value. The next time you call the next() function on the generator, it will continue where the yield left off.
This is by far more clean than the callback function code. We have cleaner code, smaller code, and not to mention much more functional code (Python allows arbitrarily large integers).
Source
add a comment |
This post will use Fibonacci numbers as a tool to build up to explaining the usefulness of Python generators.
This post will feature both C++ and Python code.
Fibonacci numbers are defined as the sequence: 0, 1, 1, 2, 3, 5, 8, 13, 21, 34, ....
Or in general:
F0 = 0
F1 = 1
Fn = Fn-1 + Fn-2
This can be transferred into a C++ function extremely easily:
size_t Fib(size_t n)
//Fib(0) = 0
if(n == 0)
return 0;
//Fib(1) = 1
if(n == 1)
return 1;
//Fib(N) = Fib(N-2) + Fib(N-1)
return Fib(n-2) + Fib(n-1);
But if you want to print the first six Fibonacci numbers, you will be recalculating a lot of the values with the above function.
For example: Fib(3) = Fib(2) + Fib(1)
, but Fib(2)
also recalculates Fib(1)
. The higher the value you want to calculate, the worse off you will be.
So one may be tempted to rewrite the above by keeping track of the state in main
.
// Not supported for the first two elements of Fib
size_t GetNextFib(size_t &pp, size_t &p)
int result = pp + p;
pp = p;
p = result;
return result;
int main(int argc, char *argv[])
size_t pp = 0;
size_t p = 1;
std::cout << "0 " << "1 ";
for(size_t i = 0; i <= 4; ++i)
size_t fibI = GetNextFib(pp, p);
std::cout << fibI << " ";
return 0;
But this is very ugly, and it complicates our logic in main
. It would be better to not have to worry about state in our main
function.
We could return a vector
of values and use an iterator
to iterate over that set of values, but this requires a lot of memory all at once for a large number of return values.
So back to our old approach, what happens if we wanted to do something else besides print the numbers? We'd have to copy and paste the whole block of code in main
and change the output statements to whatever else we wanted to do.
And if you copy and paste code, then you should be shot. You don't want to get shot, do you?
To solve these problems, and to avoid getting shot, we may rewrite this block of code using a callback function. Every time a new Fibonacci number is encountered, we would call the callback function.
void GetFibNumbers(size_t max, void(*FoundNewFibCallback)(size_t))
if(max-- == 0) return;
FoundNewFibCallback(0);
if(max-- == 0) return;
FoundNewFibCallback(1);
size_t pp = 0;
size_t p = 1;
for(;;)
if(max-- == 0) return;
int result = pp + p;
pp = p;
p = result;
FoundNewFibCallback(result);
void foundNewFib(size_t fibI)
std::cout << fibI << " ";
int main(int argc, char *argv[])
GetFibNumbers(6, foundNewFib);
return 0;
This is clearly an improvement, your logic in main
is not as cluttered, and you can do anything you want with the Fibonacci numbers, simply define new callbacks.
But this is still not perfect. What if you wanted to only get the first two Fibonacci numbers, and then do something, then get some more, then do something else?
Well, we could go on like we have been, and we could start adding state again into main
, allowing GetFibNumbers to start from an arbitrary point.
But this will further bloat our code, and it already looks too big for a simple task like printing Fibonacci numbers.
We could implement a producer and consumer model via a couple of threads. But this complicates the code even more.
Instead let's talk about generators.
Python has a very nice language feature that solves problems like these called generators.
A generator allows you to execute a function, stop at an arbitrary point, and then continue again where you left off.
Each time returning a value.
Consider the following code that uses a generator:
def fib():
pp, p = 0, 1
while 1:
yield pp
pp, p = p, pp+p
g = fib()
for i in range(6):
g.next()
Which gives us the results:
0
1
1
2
3
5
The yield
statement is used in conjuction with Python generators. It saves the state of the function and returns the yeilded value. The next time you call the next() function on the generator, it will continue where the yield left off.
This is by far more clean than the callback function code. We have cleaner code, smaller code, and not to mention much more functional code (Python allows arbitrarily large integers).
Source
add a comment |
This post will use Fibonacci numbers as a tool to build up to explaining the usefulness of Python generators.
This post will feature both C++ and Python code.
Fibonacci numbers are defined as the sequence: 0, 1, 1, 2, 3, 5, 8, 13, 21, 34, ....
Or in general:
F0 = 0
F1 = 1
Fn = Fn-1 + Fn-2
This can be transferred into a C++ function extremely easily:
size_t Fib(size_t n)
//Fib(0) = 0
if(n == 0)
return 0;
//Fib(1) = 1
if(n == 1)
return 1;
//Fib(N) = Fib(N-2) + Fib(N-1)
return Fib(n-2) + Fib(n-1);
But if you want to print the first six Fibonacci numbers, you will be recalculating a lot of the values with the above function.
For example: Fib(3) = Fib(2) + Fib(1)
, but Fib(2)
also recalculates Fib(1)
. The higher the value you want to calculate, the worse off you will be.
So one may be tempted to rewrite the above by keeping track of the state in main
.
// Not supported for the first two elements of Fib
size_t GetNextFib(size_t &pp, size_t &p)
int result = pp + p;
pp = p;
p = result;
return result;
int main(int argc, char *argv[])
size_t pp = 0;
size_t p = 1;
std::cout << "0 " << "1 ";
for(size_t i = 0; i <= 4; ++i)
size_t fibI = GetNextFib(pp, p);
std::cout << fibI << " ";
return 0;
But this is very ugly, and it complicates our logic in main
. It would be better to not have to worry about state in our main
function.
We could return a vector
of values and use an iterator
to iterate over that set of values, but this requires a lot of memory all at once for a large number of return values.
So back to our old approach, what happens if we wanted to do something else besides print the numbers? We'd have to copy and paste the whole block of code in main
and change the output statements to whatever else we wanted to do.
And if you copy and paste code, then you should be shot. You don't want to get shot, do you?
To solve these problems, and to avoid getting shot, we may rewrite this block of code using a callback function. Every time a new Fibonacci number is encountered, we would call the callback function.
void GetFibNumbers(size_t max, void(*FoundNewFibCallback)(size_t))
if(max-- == 0) return;
FoundNewFibCallback(0);
if(max-- == 0) return;
FoundNewFibCallback(1);
size_t pp = 0;
size_t p = 1;
for(;;)
if(max-- == 0) return;
int result = pp + p;
pp = p;
p = result;
FoundNewFibCallback(result);
void foundNewFib(size_t fibI)
std::cout << fibI << " ";
int main(int argc, char *argv[])
GetFibNumbers(6, foundNewFib);
return 0;
This is clearly an improvement, your logic in main
is not as cluttered, and you can do anything you want with the Fibonacci numbers, simply define new callbacks.
But this is still not perfect. What if you wanted to only get the first two Fibonacci numbers, and then do something, then get some more, then do something else?
Well, we could go on like we have been, and we could start adding state again into main
, allowing GetFibNumbers to start from an arbitrary point.
But this will further bloat our code, and it already looks too big for a simple task like printing Fibonacci numbers.
We could implement a producer and consumer model via a couple of threads. But this complicates the code even more.
Instead let's talk about generators.
Python has a very nice language feature that solves problems like these called generators.
A generator allows you to execute a function, stop at an arbitrary point, and then continue again where you left off.
Each time returning a value.
Consider the following code that uses a generator:
def fib():
pp, p = 0, 1
while 1:
yield pp
pp, p = p, pp+p
g = fib()
for i in range(6):
g.next()
Which gives us the results:
0
1
1
2
3
5
The yield
statement is used in conjuction with Python generators. It saves the state of the function and returns the yeilded value. The next time you call the next() function on the generator, it will continue where the yield left off.
This is by far more clean than the callback function code. We have cleaner code, smaller code, and not to mention much more functional code (Python allows arbitrarily large integers).
Source
This post will use Fibonacci numbers as a tool to build up to explaining the usefulness of Python generators.
This post will feature both C++ and Python code.
Fibonacci numbers are defined as the sequence: 0, 1, 1, 2, 3, 5, 8, 13, 21, 34, ....
Or in general:
F0 = 0
F1 = 1
Fn = Fn-1 + Fn-2
This can be transferred into a C++ function extremely easily:
size_t Fib(size_t n)
//Fib(0) = 0
if(n == 0)
return 0;
//Fib(1) = 1
if(n == 1)
return 1;
//Fib(N) = Fib(N-2) + Fib(N-1)
return Fib(n-2) + Fib(n-1);
But if you want to print the first six Fibonacci numbers, you will be recalculating a lot of the values with the above function.
For example: Fib(3) = Fib(2) + Fib(1)
, but Fib(2)
also recalculates Fib(1)
. The higher the value you want to calculate, the worse off you will be.
So one may be tempted to rewrite the above by keeping track of the state in main
.
// Not supported for the first two elements of Fib
size_t GetNextFib(size_t &pp, size_t &p)
int result = pp + p;
pp = p;
p = result;
return result;
int main(int argc, char *argv[])
size_t pp = 0;
size_t p = 1;
std::cout << "0 " << "1 ";
for(size_t i = 0; i <= 4; ++i)
size_t fibI = GetNextFib(pp, p);
std::cout << fibI << " ";
return 0;
But this is very ugly, and it complicates our logic in main
. It would be better to not have to worry about state in our main
function.
We could return a vector
of values and use an iterator
to iterate over that set of values, but this requires a lot of memory all at once for a large number of return values.
So back to our old approach, what happens if we wanted to do something else besides print the numbers? We'd have to copy and paste the whole block of code in main
and change the output statements to whatever else we wanted to do.
And if you copy and paste code, then you should be shot. You don't want to get shot, do you?
To solve these problems, and to avoid getting shot, we may rewrite this block of code using a callback function. Every time a new Fibonacci number is encountered, we would call the callback function.
void GetFibNumbers(size_t max, void(*FoundNewFibCallback)(size_t))
if(max-- == 0) return;
FoundNewFibCallback(0);
if(max-- == 0) return;
FoundNewFibCallback(1);
size_t pp = 0;
size_t p = 1;
for(;;)
if(max-- == 0) return;
int result = pp + p;
pp = p;
p = result;
FoundNewFibCallback(result);
void foundNewFib(size_t fibI)
std::cout << fibI << " ";
int main(int argc, char *argv[])
GetFibNumbers(6, foundNewFib);
return 0;
This is clearly an improvement, your logic in main
is not as cluttered, and you can do anything you want with the Fibonacci numbers, simply define new callbacks.
But this is still not perfect. What if you wanted to only get the first two Fibonacci numbers, and then do something, then get some more, then do something else?
Well, we could go on like we have been, and we could start adding state again into main
, allowing GetFibNumbers to start from an arbitrary point.
But this will further bloat our code, and it already looks too big for a simple task like printing Fibonacci numbers.
We could implement a producer and consumer model via a couple of threads. But this complicates the code even more.
Instead let's talk about generators.
Python has a very nice language feature that solves problems like these called generators.
A generator allows you to execute a function, stop at an arbitrary point, and then continue again where you left off.
Each time returning a value.
Consider the following code that uses a generator:
def fib():
pp, p = 0, 1
while 1:
yield pp
pp, p = p, pp+p
g = fib()
for i in range(6):
g.next()
Which gives us the results:
0
1
1
2
3
5
The yield
statement is used in conjuction with Python generators. It saves the state of the function and returns the yeilded value. The next time you call the next() function on the generator, it will continue where the yield left off.
This is by far more clean than the callback function code. We have cleaner code, smaller code, and not to mention much more functional code (Python allows arbitrarily large integers).
Source
edited May 20 '18 at 9:19


Peter Mortensen
13.8k1987113
13.8k1987113
answered Dec 19 '09 at 5:58
Brian R. BondyBrian R. Bondy
255k98544594
255k98544594
add a comment |
add a comment |
I believe the first appearance of iterators and generators were in the Icon programming language, about 20 years ago.
You may enjoy the Icon overview, which lets you wrap your head around them without concentrating on the syntax (since Icon is a language you probably don't know, and Griswold was explaining the benefits of his language to people coming from other languages).
After reading just a few paragraphs there, the utility of generators and iterators might become more apparent.
add a comment |
I believe the first appearance of iterators and generators were in the Icon programming language, about 20 years ago.
You may enjoy the Icon overview, which lets you wrap your head around them without concentrating on the syntax (since Icon is a language you probably don't know, and Griswold was explaining the benefits of his language to people coming from other languages).
After reading just a few paragraphs there, the utility of generators and iterators might become more apparent.
add a comment |
I believe the first appearance of iterators and generators were in the Icon programming language, about 20 years ago.
You may enjoy the Icon overview, which lets you wrap your head around them without concentrating on the syntax (since Icon is a language you probably don't know, and Griswold was explaining the benefits of his language to people coming from other languages).
After reading just a few paragraphs there, the utility of generators and iterators might become more apparent.
I believe the first appearance of iterators and generators were in the Icon programming language, about 20 years ago.
You may enjoy the Icon overview, which lets you wrap your head around them without concentrating on the syntax (since Icon is a language you probably don't know, and Griswold was explaining the benefits of his language to people coming from other languages).
After reading just a few paragraphs there, the utility of generators and iterators might become more apparent.
answered Nov 18 '09 at 14:53
NosrednaNosredna
59.3k1489119
59.3k1489119
add a comment |
add a comment |
Experience with list comprehensions has shown their widespread utility throughout Python. However, many of the use cases do not need to have a full list created in memory. Instead, they only need to iterate over the elements one at a time.
For instance, the following summation code will build a full list of squares in memory, iterate over those values, and, when the reference is no longer needed, delete the list:
sum([x*x for x in range(10)])
Memory is conserved by using a generator expression instead:
sum(x*x for x in range(10))
Similar benefits are conferred on constructors for container objects:
s = Set(word for line in page for word in line.split())
d = dict( (k, func(k)) for k in keylist)
Generator expressions are especially useful with functions like sum(), min(), and max() that reduce an iterable input to a single value:
max(len(line) for line in file if line.strip())
more
add a comment |
Experience with list comprehensions has shown their widespread utility throughout Python. However, many of the use cases do not need to have a full list created in memory. Instead, they only need to iterate over the elements one at a time.
For instance, the following summation code will build a full list of squares in memory, iterate over those values, and, when the reference is no longer needed, delete the list:
sum([x*x for x in range(10)])
Memory is conserved by using a generator expression instead:
sum(x*x for x in range(10))
Similar benefits are conferred on constructors for container objects:
s = Set(word for line in page for word in line.split())
d = dict( (k, func(k)) for k in keylist)
Generator expressions are especially useful with functions like sum(), min(), and max() that reduce an iterable input to a single value:
max(len(line) for line in file if line.strip())
more
add a comment |
Experience with list comprehensions has shown their widespread utility throughout Python. However, many of the use cases do not need to have a full list created in memory. Instead, they only need to iterate over the elements one at a time.
For instance, the following summation code will build a full list of squares in memory, iterate over those values, and, when the reference is no longer needed, delete the list:
sum([x*x for x in range(10)])
Memory is conserved by using a generator expression instead:
sum(x*x for x in range(10))
Similar benefits are conferred on constructors for container objects:
s = Set(word for line in page for word in line.split())
d = dict( (k, func(k)) for k in keylist)
Generator expressions are especially useful with functions like sum(), min(), and max() that reduce an iterable input to a single value:
max(len(line) for line in file if line.strip())
more
Experience with list comprehensions has shown their widespread utility throughout Python. However, many of the use cases do not need to have a full list created in memory. Instead, they only need to iterate over the elements one at a time.
For instance, the following summation code will build a full list of squares in memory, iterate over those values, and, when the reference is no longer needed, delete the list:
sum([x*x for x in range(10)])
Memory is conserved by using a generator expression instead:
sum(x*x for x in range(10))
Similar benefits are conferred on constructors for container objects:
s = Set(word for line in page for word in line.split())
d = dict( (k, func(k)) for k in keylist)
Generator expressions are especially useful with functions like sum(), min(), and max() that reduce an iterable input to a single value:
max(len(line) for line in file if line.strip())
more
edited Nov 24 '17 at 18:38
answered Nov 24 '17 at 18:28


Saqib MujtabaSaqib Mujtaba
346214
346214
add a comment |
add a comment |
protected by Marcin May 15 '13 at 21:56
Thank you for your interest in this question.
Because it has attracted low-quality or spam answers that had to be removed, posting an answer now requires 10 reputation on this site (the association bonus does not count).
Would you like to answer one of these unanswered questions instead?
UTW8i 4knpDb yC zKAlxmYXQbDfwb DP73,F H LgWSWn