How to pivot DataFrame?Does Spark supports melt and dcastHow to transpose/pivot the rows data to column in Spark Scala?Convert row values into columns with its value from another column in spark scalaHow to transpose data in Hive, Impala or Spark?Create Spark Dataframe from existing Dataframe such that new Dataframe's columns based on existing Dataframe rowspyspark dataframe using group to get multiple fields countSpark - Transpose DataFrame ColumnsHow to “dense” a data frame in SparkConverting Values to Columns in Spark Dataset (Convert Key & Value pair of columns to regular columns)PySpark - How to transpose a DataframeHow to sort a dataframe by multiple column(s)?Is the Scala 2.8 collections library a case of “the longest suicide note in history”?How to iterate over rows in a DataFrame in Pandas?Select rows from a DataFrame based on values in a column in pandasReshaping/Pivoting data in Spark RDD and/or Spark DataFramesHow to define partitioning of DataFrame?How to apply a function to a column of a Spark DataFrame?How to pivot a dataframeHow to pivot on arbitrary column?Trouble porting Scala spark code to PySpark
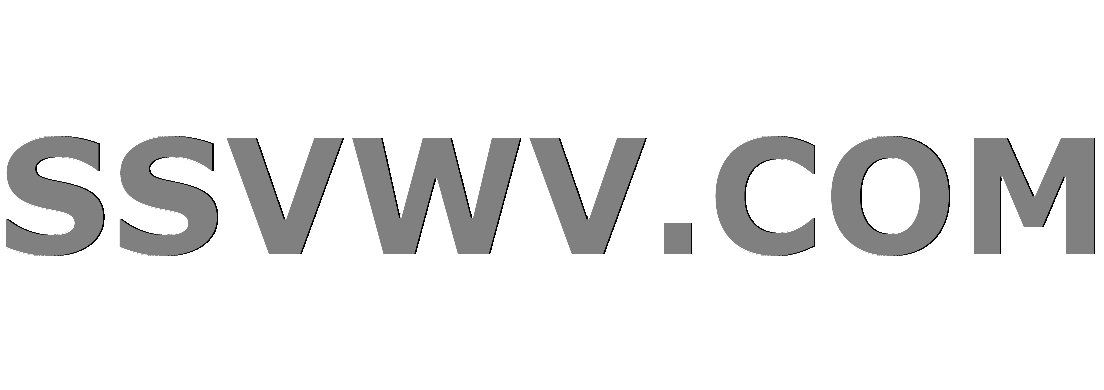
Multi tool use
Lowest total scrabble score
Is there a way to get `mathscr' with lower case letters in pdfLaTeX?
Has any country ever had 2 former presidents in jail simultaneously?
What should you do if you miss a job interview (deliberately)?
Does the Linux kernel need a file system to run?
Multiplicative persistence
Unexpected behavior of the procedure `Area` on the object 'Polygon'
Invalid date error by date command
Is there an injective, monotonically increasing, strictly concave function from the reals, to the reals?
Mimic lecturing on blackboard, facing audience
Review your own paper in Mathematics
PTIJ: Haman's bad computer
Add big quotation marks inside my colorbox
Why is the "ls" command showing permissions of files in a FAT32 partition?
Terse Method to Swap Lowest for Highest?
Does an advisor owe his/her student anything? Will an advisor keep a PhD student only out of pity?
How do you respond to a colleague from another team when they're wrongly expecting that you'll help them?
Did arcade monitors have same pixel aspect ratio as TV sets?
Why can Carol Danvers change her suit colours in the first place?
Creepy dinosaur pc game identification
Electoral considerations aside, what are potential benefits, for the US, of policy changes proposed by the tweet recognizing Golan annexation?
Does Doodling or Improvising on the Piano Have Any Benefits?
Calculating total slots
How should I respond when I lied about my education and the company finds out through background check?
How to pivot DataFrame?
Does Spark supports melt and dcastHow to transpose/pivot the rows data to column in Spark Scala?Convert row values into columns with its value from another column in spark scalaHow to transpose data in Hive, Impala or Spark?Create Spark Dataframe from existing Dataframe such that new Dataframe's columns based on existing Dataframe rowspyspark dataframe using group to get multiple fields countSpark - Transpose DataFrame ColumnsHow to “dense” a data frame in SparkConverting Values to Columns in Spark Dataset (Convert Key & Value pair of columns to regular columns)PySpark - How to transpose a DataframeHow to sort a dataframe by multiple column(s)?Is the Scala 2.8 collections library a case of “the longest suicide note in history”?How to iterate over rows in a DataFrame in Pandas?Select rows from a DataFrame based on values in a column in pandasReshaping/Pivoting data in Spark RDD and/or Spark DataFramesHow to define partitioning of DataFrame?How to apply a function to a column of a Spark DataFrame?How to pivot a dataframeHow to pivot on arbitrary column?Trouble porting Scala spark code to PySpark
I am starting to use Spark DataFrames and I need to be able to pivot the data to create multiple columns out of 1 column with multiple rows. There is built in functionality for that in Scalding and I believe in Pandas in Python, but I can't find anything for the new Spark Dataframe.
I assume I can write custom function of some sort that will do this but I'm not even sure how to start, especially since I am a novice with Spark. I anyone knows how to do this with built in functionality or suggestions for how to write something in Scala, it is greatly appreciated.
scala apache-spark dataframe apache-spark-sql pivot
add a comment |
I am starting to use Spark DataFrames and I need to be able to pivot the data to create multiple columns out of 1 column with multiple rows. There is built in functionality for that in Scalding and I believe in Pandas in Python, but I can't find anything for the new Spark Dataframe.
I assume I can write custom function of some sort that will do this but I'm not even sure how to start, especially since I am a novice with Spark. I anyone knows how to do this with built in functionality or suggestions for how to write something in Scala, it is greatly appreciated.
scala apache-spark dataframe apache-spark-sql pivot
See this similar question where I posted a native Spark approach that doesn't need to know the column/category names ahead of time.
– patricksurry
Jun 23 '15 at 15:56
add a comment |
I am starting to use Spark DataFrames and I need to be able to pivot the data to create multiple columns out of 1 column with multiple rows. There is built in functionality for that in Scalding and I believe in Pandas in Python, but I can't find anything for the new Spark Dataframe.
I assume I can write custom function of some sort that will do this but I'm not even sure how to start, especially since I am a novice with Spark. I anyone knows how to do this with built in functionality or suggestions for how to write something in Scala, it is greatly appreciated.
scala apache-spark dataframe apache-spark-sql pivot
I am starting to use Spark DataFrames and I need to be able to pivot the data to create multiple columns out of 1 column with multiple rows. There is built in functionality for that in Scalding and I believe in Pandas in Python, but I can't find anything for the new Spark Dataframe.
I assume I can write custom function of some sort that will do this but I'm not even sure how to start, especially since I am a novice with Spark. I anyone knows how to do this with built in functionality or suggestions for how to write something in Scala, it is greatly appreciated.
scala apache-spark dataframe apache-spark-sql pivot
scala apache-spark dataframe apache-spark-sql pivot
edited Jan 7 at 15:55
user6910411
35.4k1089110
35.4k1089110
asked May 14 '15 at 18:42
J CalbreathJ Calbreath
87731423
87731423
See this similar question where I posted a native Spark approach that doesn't need to know the column/category names ahead of time.
– patricksurry
Jun 23 '15 at 15:56
add a comment |
See this similar question where I posted a native Spark approach that doesn't need to know the column/category names ahead of time.
– patricksurry
Jun 23 '15 at 15:56
See this similar question where I posted a native Spark approach that doesn't need to know the column/category names ahead of time.
– patricksurry
Jun 23 '15 at 15:56
See this similar question where I posted a native Spark approach that doesn't need to know the column/category names ahead of time.
– patricksurry
Jun 23 '15 at 15:56
add a comment |
6 Answers
6
active
oldest
votes
As mentioned by David Anderson Spark provides pivot
function since version 1.6. General syntax looks as follows:
df
.groupBy(grouping_columns)
.pivot(pivot_column, [values])
.agg(aggregate_expressions)
Usage examples using nycflights13
and csv
format:
Python:
from pyspark.sql.functions import avg
flights = (sqlContext
.read
.format("csv")
.options(inferSchema="true", header="true")
.load("flights.csv")
.na.drop())
flights.registerTempTable("flights")
sqlContext.cacheTable("flights")
gexprs = ("origin", "dest", "carrier")
aggexpr = avg("arr_delay")
flights.count()
## 336776
%timeit -n10 flights.groupBy(*gexprs ).pivot("hour").agg(aggexpr).count()
## 10 loops, best of 3: 1.03 s per loop
Scala:
val flights = sqlContext
.read
.format("csv")
.options(Map("inferSchema" -> "true", "header" -> "true"))
.load("flights.csv")
flights
.groupBy($"origin", $"dest", $"carrier")
.pivot("hour")
.agg(avg($"arr_delay"))
Java:
import static org.apache.spark.sql.functions.*;
import org.apache.spark.sql.*;
Dataset<Row> df = spark.read().format("csv")
.option("inferSchema", "true")
.option("header", "true")
.load("flights.csv");
df.groupBy(col("origin"), col("dest"), col("carrier"))
.pivot("hour")
.agg(avg(col("arr_delay")));
R / SparkR:
library(magrittr)
flights <- read.df("flights.csv", source="csv", header=TRUE, inferSchema=TRUE)
flights %>%
groupBy("origin", "dest", "carrier") %>%
pivot("hour") %>%
agg(avg(column("arr_delay")))
R / sparklyr
library(dplyr)
flights <- spark_read_csv(sc, "flights", "flights.csv")
avg.arr.delay <- function(gdf)
expr <- invoke_static(
sc,
"org.apache.spark.sql.functions",
"avg",
"arr_delay"
)
gdf %>% invoke("agg", expr, list())
flights %>%
sdf_pivot(origin + dest + carrier ~ hour, fun.aggregate=avg.arr.delay)
SQL:
CREATE TEMPORARY VIEW flights
USING csv
OPTIONS (header 'true', path 'flights.csv', inferSchema 'true') ;
SELECT * FROM (
SELECT origin, dest, carrier, arr_delay, hour FROM flights
) PIVOT (
avg(arr_delay)
FOR hour IN (0, 1, 2, 3, 4, 5, 6, 7, 8, 9, 10, 11, 12,
13, 14, 15, 16, 17, 18, 19, 20, 21, 22, 23)
);
Example data:
"year","month","day","dep_time","sched_dep_time","dep_delay","arr_time","sched_arr_time","arr_delay","carrier","flight","tailnum","origin","dest","air_time","distance","hour","minute","time_hour"
2013,1,1,517,515,2,830,819,11,"UA",1545,"N14228","EWR","IAH",227,1400,5,15,2013-01-01 05:00:00
2013,1,1,533,529,4,850,830,20,"UA",1714,"N24211","LGA","IAH",227,1416,5,29,2013-01-01 05:00:00
2013,1,1,542,540,2,923,850,33,"AA",1141,"N619AA","JFK","MIA",160,1089,5,40,2013-01-01 05:00:00
2013,1,1,544,545,-1,1004,1022,-18,"B6",725,"N804JB","JFK","BQN",183,1576,5,45,2013-01-01 05:00:00
2013,1,1,554,600,-6,812,837,-25,"DL",461,"N668DN","LGA","ATL",116,762,6,0,2013-01-01 06:00:00
2013,1,1,554,558,-4,740,728,12,"UA",1696,"N39463","EWR","ORD",150,719,5,58,2013-01-01 05:00:00
2013,1,1,555,600,-5,913,854,19,"B6",507,"N516JB","EWR","FLL",158,1065,6,0,2013-01-01 06:00:00
2013,1,1,557,600,-3,709,723,-14,"EV",5708,"N829AS","LGA","IAD",53,229,6,0,2013-01-01 06:00:00
2013,1,1,557,600,-3,838,846,-8,"B6",79,"N593JB","JFK","MCO",140,944,6,0,2013-01-01 06:00:00
2013,1,1,558,600,-2,753,745,8,"AA",301,"N3ALAA","LGA","ORD",138,733,6,0,2013-01-01 06:00:00
Performance considerations:
Generally speaking pivoting is an expensive operation.
if you can try to provide
values
list:vs = list(range(25))
%timeit -n10 flights.groupBy(*gexprs ).pivot("hour", vs).agg(aggexpr).count()
## 10 loops, best of 3: 392 ms per loopin some cases it proved to be beneficial (likely no longer worth the effort in 2.0 or later) to
repartition
and / or pre-aggregate the datafor reshaping only, you can use
first
: How to use pivot and calculate average on a non-numeric column (facing AnalysisException "is not a numeric column")?
Related questions:
- How to melt Spark DataFrame?
- Unpivot in spark-sql/pyspark
- Transpose column to row with Spark
add a comment |
I overcame this by writing a for loop to dynamically create a SQL query. Say I have:
id tag value
1 US 50
1 UK 100
1 Can 125
2 US 75
2 UK 150
2 Can 175
and I want:
id US UK Can
1 50 100 125
2 75 150 175
I can create a list with the value I want to pivot and then create a string containing the SQL query I need.
val countries = List("US", "UK", "Can")
val numCountries = countries.length - 1
var query = "select *, "
for (i <- 0 to numCountries-1)
query += """case when tag = """" + countries(i) + """" then value else 0 end as """ + countries(i) + ", "
query += """case when tag = """" + countries.last + """" then value else 0 end as """ + countries.last + " from myTable"
myDataFrame.registerTempTable("myTable")
val myDF1 = sqlContext.sql(query)
I can create similar query to then do the aggregation. Not a very elegant solution but it works and is flexible for any list of values, which can also be passed in as an argument when your code is called.
I am trying to reproduce your example, but I get an "org.apache.spark.sql.AnalysisException: cannot resolve 'US' given input columns id, tag, value"
– user299791
Feb 29 '16 at 8:59
That has to do with the quotes. If you look at the resulting text string what you would get is 'case when tag = US', so Spark thinks thats a column name rather than a text value. What you really want to see is 'case when tag = "US" '. I have edited the above answer to have the correct set up for quotes.
– J Calbreath
Feb 29 '16 at 14:03
2
But as also mentioned, this is fuctionality is now native to Spark using the pivot command.
– J Calbreath
Feb 29 '16 at 14:03
add a comment |
A pivot operator has been added to the Spark dataframe API, and is part of Spark 1.6.
See https://github.com/apache/spark/pull/7841 for details.
add a comment |
I have solved a similar problem using dataframes with the following steps:
Create columns for all your countries, with 'value' as the value:
import org.apache.spark.sql.functions._
val countries = List("US", "UK", "Can")
val countryValue = udf(countryToCheck: String, countryInRow: String, value: Long) =>
if(countryToCheck == countryInRow) value else 0
val countryFuncs = countries.mapcountry => (dataFrame: DataFrame) => dataFrame.withColumn(country, countryValue(lit(country), df("tag"), df("value")))
val dfWithCountries = Function.chain(countryFuncs)(df).drop("tag").drop("value")
Your dataframe 'dfWithCountries' will look like this:
+--+--+---+---+
|id|US| UK|Can|
+--+--+---+---+
| 1|50| 0| 0|
| 1| 0|100| 0|
| 1| 0| 0|125|
| 2|75| 0| 0|
| 2| 0|150| 0|
| 2| 0| 0|175|
+--+--+---+---+
Now you can sum together all the values for your desired result:
dfWithCountries.groupBy("id").sum(countries: _*).show
Result:
+--+-------+-------+--------+
|id|SUM(US)|SUM(UK)|SUM(Can)|
+--+-------+-------+--------+
| 1| 50| 100| 125|
| 2| 75| 150| 175|
+--+-------+-------+--------+
It's not a very elegant solution though. I had to create a chain of functions to add in all the columns. Also if I have lots of countries, I will expand my temporary data set to a very wide set with lots of zeroes.
add a comment |
Initially i adopted Al M's solution. Later took the same thought and rewrote this function as a transpose function.
This method transposes any df rows to columns of any data-format with using key and value column
for input csv
id,tag,value
1,US,50a
1,UK,100
1,Can,125
2,US,75
2,UK,150
2,Can,175
ouput
+--+---+---+---+
|id| UK| US|Can|
+--+---+---+---+
| 2|150| 75|175|
| 1|100|50a|125|
+--+---+---+---+
transpose method :
def transpose(hc : HiveContext , df: DataFrame,compositeId: List[String], key: String, value: String) =
val distinctCols = df.select(key).distinct.map r => r(0) .collect().toList
val rdd = df.map row =>
(compositeId.collect case id => row.getAs(id).asInstanceOf[Any] ,
scala.collection.mutable.Map(row.getAs(key).asInstanceOf[Any] -> row.getAs(value).asInstanceOf[Any]))
val pairRdd = rdd.reduceByKey(_ ++ _)
val rowRdd = pairRdd.map(r => dynamicRow(r, distinctCols))
hc.createDataFrame(rowRdd, getSchema(df.schema, compositeId, (key, distinctCols)))
private def dynamicRow(r: (List[Any], scala.collection.mutable.Map[Any, Any]), colNames: List[Any]) =
val cols = colNames.collect case col => r._2.getOrElse(col.toString(), null)
val array = r._1 ++ cols
Row(array: _*)
private def getSchema(srcSchema: StructType, idCols: List[String], distinctCols: (String, List[Any])): StructType =
val idSchema = idCols.map idCol => srcSchema.apply(idCol)
val colSchema = srcSchema.apply(distinctCols._1)
val colsSchema = distinctCols._2.map col => StructField(col.asInstanceOf[String], colSchema.dataType, colSchema.nullable)
StructType(idSchema ++ colsSchema)
main snippet
import java.util.Date
import org.apache.spark.SparkConf
import org.apache.spark.SparkContext
import org.apache.spark.sql.Row
import org.apache.spark.sql.DataFrame
import org.apache.spark.sql.types.StructType
import org.apache.spark.sql.hive.HiveContext
import org.apache.spark.sql.types.StructField
...
...
def main(args: Array[String]): Unit =
val sc = new SparkContext(conf)
val sqlContext = new org.apache.spark.sql.SQLContext(sc)
val dfdata1 = sqlContext.read.format("com.databricks.spark.csv").option("header", "true").option("inferSchema", "true")
.load("data.csv")
dfdata1.show()
val dfOutput = transpose(new HiveContext(sc), dfdata1, List("id"), "tag", "value")
dfOutput.show
2
This methods transposes rows to columns...
– Jaigates
Sep 6 '16 at 18:38
add a comment |
There is simple and elegant solution.
scala> spark.sql("select * from k_tags limit 10").show()
+---------------+-------------+------+
| imsi| name| value|
+---------------+-------------+------+
|246021000000000| age| 37|
|246021000000000| gender|Female|
|246021000000000| arpu| 22|
|246021000000000| DeviceType| Phone|
|246021000000000|DataAllowance| 6GB|
+---------------+-------------+------+
scala> spark.sql("select * from k_tags limit 10").groupBy($"imsi").pivot("name").agg(min($"value")).show()
+---------------+-------------+----------+---+----+------+
| imsi|DataAllowance|DeviceType|age|arpu|gender|
+---------------+-------------+----------+---+----+------+
|246021000000000| 6GB| Phone| 37| 22|Female|
|246021000000001| 1GB| Phone| 72| 10| Male|
+---------------+-------------+----------+---+----+------+
add a comment |
protected by user8371915 Jul 15 '18 at 19:20
Thank you for your interest in this question.
Because it has attracted low-quality or spam answers that had to be removed, posting an answer now requires 10 reputation on this site (the association bonus does not count).
Would you like to answer one of these unanswered questions instead?
6 Answers
6
active
oldest
votes
6 Answers
6
active
oldest
votes
active
oldest
votes
active
oldest
votes
As mentioned by David Anderson Spark provides pivot
function since version 1.6. General syntax looks as follows:
df
.groupBy(grouping_columns)
.pivot(pivot_column, [values])
.agg(aggregate_expressions)
Usage examples using nycflights13
and csv
format:
Python:
from pyspark.sql.functions import avg
flights = (sqlContext
.read
.format("csv")
.options(inferSchema="true", header="true")
.load("flights.csv")
.na.drop())
flights.registerTempTable("flights")
sqlContext.cacheTable("flights")
gexprs = ("origin", "dest", "carrier")
aggexpr = avg("arr_delay")
flights.count()
## 336776
%timeit -n10 flights.groupBy(*gexprs ).pivot("hour").agg(aggexpr).count()
## 10 loops, best of 3: 1.03 s per loop
Scala:
val flights = sqlContext
.read
.format("csv")
.options(Map("inferSchema" -> "true", "header" -> "true"))
.load("flights.csv")
flights
.groupBy($"origin", $"dest", $"carrier")
.pivot("hour")
.agg(avg($"arr_delay"))
Java:
import static org.apache.spark.sql.functions.*;
import org.apache.spark.sql.*;
Dataset<Row> df = spark.read().format("csv")
.option("inferSchema", "true")
.option("header", "true")
.load("flights.csv");
df.groupBy(col("origin"), col("dest"), col("carrier"))
.pivot("hour")
.agg(avg(col("arr_delay")));
R / SparkR:
library(magrittr)
flights <- read.df("flights.csv", source="csv", header=TRUE, inferSchema=TRUE)
flights %>%
groupBy("origin", "dest", "carrier") %>%
pivot("hour") %>%
agg(avg(column("arr_delay")))
R / sparklyr
library(dplyr)
flights <- spark_read_csv(sc, "flights", "flights.csv")
avg.arr.delay <- function(gdf)
expr <- invoke_static(
sc,
"org.apache.spark.sql.functions",
"avg",
"arr_delay"
)
gdf %>% invoke("agg", expr, list())
flights %>%
sdf_pivot(origin + dest + carrier ~ hour, fun.aggregate=avg.arr.delay)
SQL:
CREATE TEMPORARY VIEW flights
USING csv
OPTIONS (header 'true', path 'flights.csv', inferSchema 'true') ;
SELECT * FROM (
SELECT origin, dest, carrier, arr_delay, hour FROM flights
) PIVOT (
avg(arr_delay)
FOR hour IN (0, 1, 2, 3, 4, 5, 6, 7, 8, 9, 10, 11, 12,
13, 14, 15, 16, 17, 18, 19, 20, 21, 22, 23)
);
Example data:
"year","month","day","dep_time","sched_dep_time","dep_delay","arr_time","sched_arr_time","arr_delay","carrier","flight","tailnum","origin","dest","air_time","distance","hour","minute","time_hour"
2013,1,1,517,515,2,830,819,11,"UA",1545,"N14228","EWR","IAH",227,1400,5,15,2013-01-01 05:00:00
2013,1,1,533,529,4,850,830,20,"UA",1714,"N24211","LGA","IAH",227,1416,5,29,2013-01-01 05:00:00
2013,1,1,542,540,2,923,850,33,"AA",1141,"N619AA","JFK","MIA",160,1089,5,40,2013-01-01 05:00:00
2013,1,1,544,545,-1,1004,1022,-18,"B6",725,"N804JB","JFK","BQN",183,1576,5,45,2013-01-01 05:00:00
2013,1,1,554,600,-6,812,837,-25,"DL",461,"N668DN","LGA","ATL",116,762,6,0,2013-01-01 06:00:00
2013,1,1,554,558,-4,740,728,12,"UA",1696,"N39463","EWR","ORD",150,719,5,58,2013-01-01 05:00:00
2013,1,1,555,600,-5,913,854,19,"B6",507,"N516JB","EWR","FLL",158,1065,6,0,2013-01-01 06:00:00
2013,1,1,557,600,-3,709,723,-14,"EV",5708,"N829AS","LGA","IAD",53,229,6,0,2013-01-01 06:00:00
2013,1,1,557,600,-3,838,846,-8,"B6",79,"N593JB","JFK","MCO",140,944,6,0,2013-01-01 06:00:00
2013,1,1,558,600,-2,753,745,8,"AA",301,"N3ALAA","LGA","ORD",138,733,6,0,2013-01-01 06:00:00
Performance considerations:
Generally speaking pivoting is an expensive operation.
if you can try to provide
values
list:vs = list(range(25))
%timeit -n10 flights.groupBy(*gexprs ).pivot("hour", vs).agg(aggexpr).count()
## 10 loops, best of 3: 392 ms per loopin some cases it proved to be beneficial (likely no longer worth the effort in 2.0 or later) to
repartition
and / or pre-aggregate the datafor reshaping only, you can use
first
: How to use pivot and calculate average on a non-numeric column (facing AnalysisException "is not a numeric column")?
Related questions:
- How to melt Spark DataFrame?
- Unpivot in spark-sql/pyspark
- Transpose column to row with Spark
add a comment |
As mentioned by David Anderson Spark provides pivot
function since version 1.6. General syntax looks as follows:
df
.groupBy(grouping_columns)
.pivot(pivot_column, [values])
.agg(aggregate_expressions)
Usage examples using nycflights13
and csv
format:
Python:
from pyspark.sql.functions import avg
flights = (sqlContext
.read
.format("csv")
.options(inferSchema="true", header="true")
.load("flights.csv")
.na.drop())
flights.registerTempTable("flights")
sqlContext.cacheTable("flights")
gexprs = ("origin", "dest", "carrier")
aggexpr = avg("arr_delay")
flights.count()
## 336776
%timeit -n10 flights.groupBy(*gexprs ).pivot("hour").agg(aggexpr).count()
## 10 loops, best of 3: 1.03 s per loop
Scala:
val flights = sqlContext
.read
.format("csv")
.options(Map("inferSchema" -> "true", "header" -> "true"))
.load("flights.csv")
flights
.groupBy($"origin", $"dest", $"carrier")
.pivot("hour")
.agg(avg($"arr_delay"))
Java:
import static org.apache.spark.sql.functions.*;
import org.apache.spark.sql.*;
Dataset<Row> df = spark.read().format("csv")
.option("inferSchema", "true")
.option("header", "true")
.load("flights.csv");
df.groupBy(col("origin"), col("dest"), col("carrier"))
.pivot("hour")
.agg(avg(col("arr_delay")));
R / SparkR:
library(magrittr)
flights <- read.df("flights.csv", source="csv", header=TRUE, inferSchema=TRUE)
flights %>%
groupBy("origin", "dest", "carrier") %>%
pivot("hour") %>%
agg(avg(column("arr_delay")))
R / sparklyr
library(dplyr)
flights <- spark_read_csv(sc, "flights", "flights.csv")
avg.arr.delay <- function(gdf)
expr <- invoke_static(
sc,
"org.apache.spark.sql.functions",
"avg",
"arr_delay"
)
gdf %>% invoke("agg", expr, list())
flights %>%
sdf_pivot(origin + dest + carrier ~ hour, fun.aggregate=avg.arr.delay)
SQL:
CREATE TEMPORARY VIEW flights
USING csv
OPTIONS (header 'true', path 'flights.csv', inferSchema 'true') ;
SELECT * FROM (
SELECT origin, dest, carrier, arr_delay, hour FROM flights
) PIVOT (
avg(arr_delay)
FOR hour IN (0, 1, 2, 3, 4, 5, 6, 7, 8, 9, 10, 11, 12,
13, 14, 15, 16, 17, 18, 19, 20, 21, 22, 23)
);
Example data:
"year","month","day","dep_time","sched_dep_time","dep_delay","arr_time","sched_arr_time","arr_delay","carrier","flight","tailnum","origin","dest","air_time","distance","hour","minute","time_hour"
2013,1,1,517,515,2,830,819,11,"UA",1545,"N14228","EWR","IAH",227,1400,5,15,2013-01-01 05:00:00
2013,1,1,533,529,4,850,830,20,"UA",1714,"N24211","LGA","IAH",227,1416,5,29,2013-01-01 05:00:00
2013,1,1,542,540,2,923,850,33,"AA",1141,"N619AA","JFK","MIA",160,1089,5,40,2013-01-01 05:00:00
2013,1,1,544,545,-1,1004,1022,-18,"B6",725,"N804JB","JFK","BQN",183,1576,5,45,2013-01-01 05:00:00
2013,1,1,554,600,-6,812,837,-25,"DL",461,"N668DN","LGA","ATL",116,762,6,0,2013-01-01 06:00:00
2013,1,1,554,558,-4,740,728,12,"UA",1696,"N39463","EWR","ORD",150,719,5,58,2013-01-01 05:00:00
2013,1,1,555,600,-5,913,854,19,"B6",507,"N516JB","EWR","FLL",158,1065,6,0,2013-01-01 06:00:00
2013,1,1,557,600,-3,709,723,-14,"EV",5708,"N829AS","LGA","IAD",53,229,6,0,2013-01-01 06:00:00
2013,1,1,557,600,-3,838,846,-8,"B6",79,"N593JB","JFK","MCO",140,944,6,0,2013-01-01 06:00:00
2013,1,1,558,600,-2,753,745,8,"AA",301,"N3ALAA","LGA","ORD",138,733,6,0,2013-01-01 06:00:00
Performance considerations:
Generally speaking pivoting is an expensive operation.
if you can try to provide
values
list:vs = list(range(25))
%timeit -n10 flights.groupBy(*gexprs ).pivot("hour", vs).agg(aggexpr).count()
## 10 loops, best of 3: 392 ms per loopin some cases it proved to be beneficial (likely no longer worth the effort in 2.0 or later) to
repartition
and / or pre-aggregate the datafor reshaping only, you can use
first
: How to use pivot and calculate average on a non-numeric column (facing AnalysisException "is not a numeric column")?
Related questions:
- How to melt Spark DataFrame?
- Unpivot in spark-sql/pyspark
- Transpose column to row with Spark
add a comment |
As mentioned by David Anderson Spark provides pivot
function since version 1.6. General syntax looks as follows:
df
.groupBy(grouping_columns)
.pivot(pivot_column, [values])
.agg(aggregate_expressions)
Usage examples using nycflights13
and csv
format:
Python:
from pyspark.sql.functions import avg
flights = (sqlContext
.read
.format("csv")
.options(inferSchema="true", header="true")
.load("flights.csv")
.na.drop())
flights.registerTempTable("flights")
sqlContext.cacheTable("flights")
gexprs = ("origin", "dest", "carrier")
aggexpr = avg("arr_delay")
flights.count()
## 336776
%timeit -n10 flights.groupBy(*gexprs ).pivot("hour").agg(aggexpr).count()
## 10 loops, best of 3: 1.03 s per loop
Scala:
val flights = sqlContext
.read
.format("csv")
.options(Map("inferSchema" -> "true", "header" -> "true"))
.load("flights.csv")
flights
.groupBy($"origin", $"dest", $"carrier")
.pivot("hour")
.agg(avg($"arr_delay"))
Java:
import static org.apache.spark.sql.functions.*;
import org.apache.spark.sql.*;
Dataset<Row> df = spark.read().format("csv")
.option("inferSchema", "true")
.option("header", "true")
.load("flights.csv");
df.groupBy(col("origin"), col("dest"), col("carrier"))
.pivot("hour")
.agg(avg(col("arr_delay")));
R / SparkR:
library(magrittr)
flights <- read.df("flights.csv", source="csv", header=TRUE, inferSchema=TRUE)
flights %>%
groupBy("origin", "dest", "carrier") %>%
pivot("hour") %>%
agg(avg(column("arr_delay")))
R / sparklyr
library(dplyr)
flights <- spark_read_csv(sc, "flights", "flights.csv")
avg.arr.delay <- function(gdf)
expr <- invoke_static(
sc,
"org.apache.spark.sql.functions",
"avg",
"arr_delay"
)
gdf %>% invoke("agg", expr, list())
flights %>%
sdf_pivot(origin + dest + carrier ~ hour, fun.aggregate=avg.arr.delay)
SQL:
CREATE TEMPORARY VIEW flights
USING csv
OPTIONS (header 'true', path 'flights.csv', inferSchema 'true') ;
SELECT * FROM (
SELECT origin, dest, carrier, arr_delay, hour FROM flights
) PIVOT (
avg(arr_delay)
FOR hour IN (0, 1, 2, 3, 4, 5, 6, 7, 8, 9, 10, 11, 12,
13, 14, 15, 16, 17, 18, 19, 20, 21, 22, 23)
);
Example data:
"year","month","day","dep_time","sched_dep_time","dep_delay","arr_time","sched_arr_time","arr_delay","carrier","flight","tailnum","origin","dest","air_time","distance","hour","minute","time_hour"
2013,1,1,517,515,2,830,819,11,"UA",1545,"N14228","EWR","IAH",227,1400,5,15,2013-01-01 05:00:00
2013,1,1,533,529,4,850,830,20,"UA",1714,"N24211","LGA","IAH",227,1416,5,29,2013-01-01 05:00:00
2013,1,1,542,540,2,923,850,33,"AA",1141,"N619AA","JFK","MIA",160,1089,5,40,2013-01-01 05:00:00
2013,1,1,544,545,-1,1004,1022,-18,"B6",725,"N804JB","JFK","BQN",183,1576,5,45,2013-01-01 05:00:00
2013,1,1,554,600,-6,812,837,-25,"DL",461,"N668DN","LGA","ATL",116,762,6,0,2013-01-01 06:00:00
2013,1,1,554,558,-4,740,728,12,"UA",1696,"N39463","EWR","ORD",150,719,5,58,2013-01-01 05:00:00
2013,1,1,555,600,-5,913,854,19,"B6",507,"N516JB","EWR","FLL",158,1065,6,0,2013-01-01 06:00:00
2013,1,1,557,600,-3,709,723,-14,"EV",5708,"N829AS","LGA","IAD",53,229,6,0,2013-01-01 06:00:00
2013,1,1,557,600,-3,838,846,-8,"B6",79,"N593JB","JFK","MCO",140,944,6,0,2013-01-01 06:00:00
2013,1,1,558,600,-2,753,745,8,"AA",301,"N3ALAA","LGA","ORD",138,733,6,0,2013-01-01 06:00:00
Performance considerations:
Generally speaking pivoting is an expensive operation.
if you can try to provide
values
list:vs = list(range(25))
%timeit -n10 flights.groupBy(*gexprs ).pivot("hour", vs).agg(aggexpr).count()
## 10 loops, best of 3: 392 ms per loopin some cases it proved to be beneficial (likely no longer worth the effort in 2.0 or later) to
repartition
and / or pre-aggregate the datafor reshaping only, you can use
first
: How to use pivot and calculate average on a non-numeric column (facing AnalysisException "is not a numeric column")?
Related questions:
- How to melt Spark DataFrame?
- Unpivot in spark-sql/pyspark
- Transpose column to row with Spark
As mentioned by David Anderson Spark provides pivot
function since version 1.6. General syntax looks as follows:
df
.groupBy(grouping_columns)
.pivot(pivot_column, [values])
.agg(aggregate_expressions)
Usage examples using nycflights13
and csv
format:
Python:
from pyspark.sql.functions import avg
flights = (sqlContext
.read
.format("csv")
.options(inferSchema="true", header="true")
.load("flights.csv")
.na.drop())
flights.registerTempTable("flights")
sqlContext.cacheTable("flights")
gexprs = ("origin", "dest", "carrier")
aggexpr = avg("arr_delay")
flights.count()
## 336776
%timeit -n10 flights.groupBy(*gexprs ).pivot("hour").agg(aggexpr).count()
## 10 loops, best of 3: 1.03 s per loop
Scala:
val flights = sqlContext
.read
.format("csv")
.options(Map("inferSchema" -> "true", "header" -> "true"))
.load("flights.csv")
flights
.groupBy($"origin", $"dest", $"carrier")
.pivot("hour")
.agg(avg($"arr_delay"))
Java:
import static org.apache.spark.sql.functions.*;
import org.apache.spark.sql.*;
Dataset<Row> df = spark.read().format("csv")
.option("inferSchema", "true")
.option("header", "true")
.load("flights.csv");
df.groupBy(col("origin"), col("dest"), col("carrier"))
.pivot("hour")
.agg(avg(col("arr_delay")));
R / SparkR:
library(magrittr)
flights <- read.df("flights.csv", source="csv", header=TRUE, inferSchema=TRUE)
flights %>%
groupBy("origin", "dest", "carrier") %>%
pivot("hour") %>%
agg(avg(column("arr_delay")))
R / sparklyr
library(dplyr)
flights <- spark_read_csv(sc, "flights", "flights.csv")
avg.arr.delay <- function(gdf)
expr <- invoke_static(
sc,
"org.apache.spark.sql.functions",
"avg",
"arr_delay"
)
gdf %>% invoke("agg", expr, list())
flights %>%
sdf_pivot(origin + dest + carrier ~ hour, fun.aggregate=avg.arr.delay)
SQL:
CREATE TEMPORARY VIEW flights
USING csv
OPTIONS (header 'true', path 'flights.csv', inferSchema 'true') ;
SELECT * FROM (
SELECT origin, dest, carrier, arr_delay, hour FROM flights
) PIVOT (
avg(arr_delay)
FOR hour IN (0, 1, 2, 3, 4, 5, 6, 7, 8, 9, 10, 11, 12,
13, 14, 15, 16, 17, 18, 19, 20, 21, 22, 23)
);
Example data:
"year","month","day","dep_time","sched_dep_time","dep_delay","arr_time","sched_arr_time","arr_delay","carrier","flight","tailnum","origin","dest","air_time","distance","hour","minute","time_hour"
2013,1,1,517,515,2,830,819,11,"UA",1545,"N14228","EWR","IAH",227,1400,5,15,2013-01-01 05:00:00
2013,1,1,533,529,4,850,830,20,"UA",1714,"N24211","LGA","IAH",227,1416,5,29,2013-01-01 05:00:00
2013,1,1,542,540,2,923,850,33,"AA",1141,"N619AA","JFK","MIA",160,1089,5,40,2013-01-01 05:00:00
2013,1,1,544,545,-1,1004,1022,-18,"B6",725,"N804JB","JFK","BQN",183,1576,5,45,2013-01-01 05:00:00
2013,1,1,554,600,-6,812,837,-25,"DL",461,"N668DN","LGA","ATL",116,762,6,0,2013-01-01 06:00:00
2013,1,1,554,558,-4,740,728,12,"UA",1696,"N39463","EWR","ORD",150,719,5,58,2013-01-01 05:00:00
2013,1,1,555,600,-5,913,854,19,"B6",507,"N516JB","EWR","FLL",158,1065,6,0,2013-01-01 06:00:00
2013,1,1,557,600,-3,709,723,-14,"EV",5708,"N829AS","LGA","IAD",53,229,6,0,2013-01-01 06:00:00
2013,1,1,557,600,-3,838,846,-8,"B6",79,"N593JB","JFK","MCO",140,944,6,0,2013-01-01 06:00:00
2013,1,1,558,600,-2,753,745,8,"AA",301,"N3ALAA","LGA","ORD",138,733,6,0,2013-01-01 06:00:00
Performance considerations:
Generally speaking pivoting is an expensive operation.
if you can try to provide
values
list:vs = list(range(25))
%timeit -n10 flights.groupBy(*gexprs ).pivot("hour", vs).agg(aggexpr).count()
## 10 loops, best of 3: 392 ms per loopin some cases it proved to be beneficial (likely no longer worth the effort in 2.0 or later) to
repartition
and / or pre-aggregate the datafor reshaping only, you can use
first
: How to use pivot and calculate average on a non-numeric column (facing AnalysisException "is not a numeric column")?
Related questions:
- How to melt Spark DataFrame?
- Unpivot in spark-sql/pyspark
- Transpose column to row with Spark
edited Nov 22 '18 at 11:11
community wiki
13 revs, 3 users 60%
zero323
add a comment |
add a comment |
I overcame this by writing a for loop to dynamically create a SQL query. Say I have:
id tag value
1 US 50
1 UK 100
1 Can 125
2 US 75
2 UK 150
2 Can 175
and I want:
id US UK Can
1 50 100 125
2 75 150 175
I can create a list with the value I want to pivot and then create a string containing the SQL query I need.
val countries = List("US", "UK", "Can")
val numCountries = countries.length - 1
var query = "select *, "
for (i <- 0 to numCountries-1)
query += """case when tag = """" + countries(i) + """" then value else 0 end as """ + countries(i) + ", "
query += """case when tag = """" + countries.last + """" then value else 0 end as """ + countries.last + " from myTable"
myDataFrame.registerTempTable("myTable")
val myDF1 = sqlContext.sql(query)
I can create similar query to then do the aggregation. Not a very elegant solution but it works and is flexible for any list of values, which can also be passed in as an argument when your code is called.
I am trying to reproduce your example, but I get an "org.apache.spark.sql.AnalysisException: cannot resolve 'US' given input columns id, tag, value"
– user299791
Feb 29 '16 at 8:59
That has to do with the quotes. If you look at the resulting text string what you would get is 'case when tag = US', so Spark thinks thats a column name rather than a text value. What you really want to see is 'case when tag = "US" '. I have edited the above answer to have the correct set up for quotes.
– J Calbreath
Feb 29 '16 at 14:03
2
But as also mentioned, this is fuctionality is now native to Spark using the pivot command.
– J Calbreath
Feb 29 '16 at 14:03
add a comment |
I overcame this by writing a for loop to dynamically create a SQL query. Say I have:
id tag value
1 US 50
1 UK 100
1 Can 125
2 US 75
2 UK 150
2 Can 175
and I want:
id US UK Can
1 50 100 125
2 75 150 175
I can create a list with the value I want to pivot and then create a string containing the SQL query I need.
val countries = List("US", "UK", "Can")
val numCountries = countries.length - 1
var query = "select *, "
for (i <- 0 to numCountries-1)
query += """case when tag = """" + countries(i) + """" then value else 0 end as """ + countries(i) + ", "
query += """case when tag = """" + countries.last + """" then value else 0 end as """ + countries.last + " from myTable"
myDataFrame.registerTempTable("myTable")
val myDF1 = sqlContext.sql(query)
I can create similar query to then do the aggregation. Not a very elegant solution but it works and is flexible for any list of values, which can also be passed in as an argument when your code is called.
I am trying to reproduce your example, but I get an "org.apache.spark.sql.AnalysisException: cannot resolve 'US' given input columns id, tag, value"
– user299791
Feb 29 '16 at 8:59
That has to do with the quotes. If you look at the resulting text string what you would get is 'case when tag = US', so Spark thinks thats a column name rather than a text value. What you really want to see is 'case when tag = "US" '. I have edited the above answer to have the correct set up for quotes.
– J Calbreath
Feb 29 '16 at 14:03
2
But as also mentioned, this is fuctionality is now native to Spark using the pivot command.
– J Calbreath
Feb 29 '16 at 14:03
add a comment |
I overcame this by writing a for loop to dynamically create a SQL query. Say I have:
id tag value
1 US 50
1 UK 100
1 Can 125
2 US 75
2 UK 150
2 Can 175
and I want:
id US UK Can
1 50 100 125
2 75 150 175
I can create a list with the value I want to pivot and then create a string containing the SQL query I need.
val countries = List("US", "UK", "Can")
val numCountries = countries.length - 1
var query = "select *, "
for (i <- 0 to numCountries-1)
query += """case when tag = """" + countries(i) + """" then value else 0 end as """ + countries(i) + ", "
query += """case when tag = """" + countries.last + """" then value else 0 end as """ + countries.last + " from myTable"
myDataFrame.registerTempTable("myTable")
val myDF1 = sqlContext.sql(query)
I can create similar query to then do the aggregation. Not a very elegant solution but it works and is flexible for any list of values, which can also be passed in as an argument when your code is called.
I overcame this by writing a for loop to dynamically create a SQL query. Say I have:
id tag value
1 US 50
1 UK 100
1 Can 125
2 US 75
2 UK 150
2 Can 175
and I want:
id US UK Can
1 50 100 125
2 75 150 175
I can create a list with the value I want to pivot and then create a string containing the SQL query I need.
val countries = List("US", "UK", "Can")
val numCountries = countries.length - 1
var query = "select *, "
for (i <- 0 to numCountries-1)
query += """case when tag = """" + countries(i) + """" then value else 0 end as """ + countries(i) + ", "
query += """case when tag = """" + countries.last + """" then value else 0 end as """ + countries.last + " from myTable"
myDataFrame.registerTempTable("myTable")
val myDF1 = sqlContext.sql(query)
I can create similar query to then do the aggregation. Not a very elegant solution but it works and is flexible for any list of values, which can also be passed in as an argument when your code is called.
edited Sep 3 '17 at 20:31
zero323
172k42503587
172k42503587
answered May 22 '15 at 13:21
J CalbreathJ Calbreath
87731423
87731423
I am trying to reproduce your example, but I get an "org.apache.spark.sql.AnalysisException: cannot resolve 'US' given input columns id, tag, value"
– user299791
Feb 29 '16 at 8:59
That has to do with the quotes. If you look at the resulting text string what you would get is 'case when tag = US', so Spark thinks thats a column name rather than a text value. What you really want to see is 'case when tag = "US" '. I have edited the above answer to have the correct set up for quotes.
– J Calbreath
Feb 29 '16 at 14:03
2
But as also mentioned, this is fuctionality is now native to Spark using the pivot command.
– J Calbreath
Feb 29 '16 at 14:03
add a comment |
I am trying to reproduce your example, but I get an "org.apache.spark.sql.AnalysisException: cannot resolve 'US' given input columns id, tag, value"
– user299791
Feb 29 '16 at 8:59
That has to do with the quotes. If you look at the resulting text string what you would get is 'case when tag = US', so Spark thinks thats a column name rather than a text value. What you really want to see is 'case when tag = "US" '. I have edited the above answer to have the correct set up for quotes.
– J Calbreath
Feb 29 '16 at 14:03
2
But as also mentioned, this is fuctionality is now native to Spark using the pivot command.
– J Calbreath
Feb 29 '16 at 14:03
I am trying to reproduce your example, but I get an "org.apache.spark.sql.AnalysisException: cannot resolve 'US' given input columns id, tag, value"
– user299791
Feb 29 '16 at 8:59
I am trying to reproduce your example, but I get an "org.apache.spark.sql.AnalysisException: cannot resolve 'US' given input columns id, tag, value"
– user299791
Feb 29 '16 at 8:59
That has to do with the quotes. If you look at the resulting text string what you would get is 'case when tag = US', so Spark thinks thats a column name rather than a text value. What you really want to see is 'case when tag = "US" '. I have edited the above answer to have the correct set up for quotes.
– J Calbreath
Feb 29 '16 at 14:03
That has to do with the quotes. If you look at the resulting text string what you would get is 'case when tag = US', so Spark thinks thats a column name rather than a text value. What you really want to see is 'case when tag = "US" '. I have edited the above answer to have the correct set up for quotes.
– J Calbreath
Feb 29 '16 at 14:03
2
2
But as also mentioned, this is fuctionality is now native to Spark using the pivot command.
– J Calbreath
Feb 29 '16 at 14:03
But as also mentioned, this is fuctionality is now native to Spark using the pivot command.
– J Calbreath
Feb 29 '16 at 14:03
add a comment |
A pivot operator has been added to the Spark dataframe API, and is part of Spark 1.6.
See https://github.com/apache/spark/pull/7841 for details.
add a comment |
A pivot operator has been added to the Spark dataframe API, and is part of Spark 1.6.
See https://github.com/apache/spark/pull/7841 for details.
add a comment |
A pivot operator has been added to the Spark dataframe API, and is part of Spark 1.6.
See https://github.com/apache/spark/pull/7841 for details.
A pivot operator has been added to the Spark dataframe API, and is part of Spark 1.6.
See https://github.com/apache/spark/pull/7841 for details.
answered Nov 19 '15 at 8:47


David AndersonDavid Anderson
6,51421424
6,51421424
add a comment |
add a comment |
I have solved a similar problem using dataframes with the following steps:
Create columns for all your countries, with 'value' as the value:
import org.apache.spark.sql.functions._
val countries = List("US", "UK", "Can")
val countryValue = udf(countryToCheck: String, countryInRow: String, value: Long) =>
if(countryToCheck == countryInRow) value else 0
val countryFuncs = countries.mapcountry => (dataFrame: DataFrame) => dataFrame.withColumn(country, countryValue(lit(country), df("tag"), df("value")))
val dfWithCountries = Function.chain(countryFuncs)(df).drop("tag").drop("value")
Your dataframe 'dfWithCountries' will look like this:
+--+--+---+---+
|id|US| UK|Can|
+--+--+---+---+
| 1|50| 0| 0|
| 1| 0|100| 0|
| 1| 0| 0|125|
| 2|75| 0| 0|
| 2| 0|150| 0|
| 2| 0| 0|175|
+--+--+---+---+
Now you can sum together all the values for your desired result:
dfWithCountries.groupBy("id").sum(countries: _*).show
Result:
+--+-------+-------+--------+
|id|SUM(US)|SUM(UK)|SUM(Can)|
+--+-------+-------+--------+
| 1| 50| 100| 125|
| 2| 75| 150| 175|
+--+-------+-------+--------+
It's not a very elegant solution though. I had to create a chain of functions to add in all the columns. Also if I have lots of countries, I will expand my temporary data set to a very wide set with lots of zeroes.
add a comment |
I have solved a similar problem using dataframes with the following steps:
Create columns for all your countries, with 'value' as the value:
import org.apache.spark.sql.functions._
val countries = List("US", "UK", "Can")
val countryValue = udf(countryToCheck: String, countryInRow: String, value: Long) =>
if(countryToCheck == countryInRow) value else 0
val countryFuncs = countries.mapcountry => (dataFrame: DataFrame) => dataFrame.withColumn(country, countryValue(lit(country), df("tag"), df("value")))
val dfWithCountries = Function.chain(countryFuncs)(df).drop("tag").drop("value")
Your dataframe 'dfWithCountries' will look like this:
+--+--+---+---+
|id|US| UK|Can|
+--+--+---+---+
| 1|50| 0| 0|
| 1| 0|100| 0|
| 1| 0| 0|125|
| 2|75| 0| 0|
| 2| 0|150| 0|
| 2| 0| 0|175|
+--+--+---+---+
Now you can sum together all the values for your desired result:
dfWithCountries.groupBy("id").sum(countries: _*).show
Result:
+--+-------+-------+--------+
|id|SUM(US)|SUM(UK)|SUM(Can)|
+--+-------+-------+--------+
| 1| 50| 100| 125|
| 2| 75| 150| 175|
+--+-------+-------+--------+
It's not a very elegant solution though. I had to create a chain of functions to add in all the columns. Also if I have lots of countries, I will expand my temporary data set to a very wide set with lots of zeroes.
add a comment |
I have solved a similar problem using dataframes with the following steps:
Create columns for all your countries, with 'value' as the value:
import org.apache.spark.sql.functions._
val countries = List("US", "UK", "Can")
val countryValue = udf(countryToCheck: String, countryInRow: String, value: Long) =>
if(countryToCheck == countryInRow) value else 0
val countryFuncs = countries.mapcountry => (dataFrame: DataFrame) => dataFrame.withColumn(country, countryValue(lit(country), df("tag"), df("value")))
val dfWithCountries = Function.chain(countryFuncs)(df).drop("tag").drop("value")
Your dataframe 'dfWithCountries' will look like this:
+--+--+---+---+
|id|US| UK|Can|
+--+--+---+---+
| 1|50| 0| 0|
| 1| 0|100| 0|
| 1| 0| 0|125|
| 2|75| 0| 0|
| 2| 0|150| 0|
| 2| 0| 0|175|
+--+--+---+---+
Now you can sum together all the values for your desired result:
dfWithCountries.groupBy("id").sum(countries: _*).show
Result:
+--+-------+-------+--------+
|id|SUM(US)|SUM(UK)|SUM(Can)|
+--+-------+-------+--------+
| 1| 50| 100| 125|
| 2| 75| 150| 175|
+--+-------+-------+--------+
It's not a very elegant solution though. I had to create a chain of functions to add in all the columns. Also if I have lots of countries, I will expand my temporary data set to a very wide set with lots of zeroes.
I have solved a similar problem using dataframes with the following steps:
Create columns for all your countries, with 'value' as the value:
import org.apache.spark.sql.functions._
val countries = List("US", "UK", "Can")
val countryValue = udf(countryToCheck: String, countryInRow: String, value: Long) =>
if(countryToCheck == countryInRow) value else 0
val countryFuncs = countries.mapcountry => (dataFrame: DataFrame) => dataFrame.withColumn(country, countryValue(lit(country), df("tag"), df("value")))
val dfWithCountries = Function.chain(countryFuncs)(df).drop("tag").drop("value")
Your dataframe 'dfWithCountries' will look like this:
+--+--+---+---+
|id|US| UK|Can|
+--+--+---+---+
| 1|50| 0| 0|
| 1| 0|100| 0|
| 1| 0| 0|125|
| 2|75| 0| 0|
| 2| 0|150| 0|
| 2| 0| 0|175|
+--+--+---+---+
Now you can sum together all the values for your desired result:
dfWithCountries.groupBy("id").sum(countries: _*).show
Result:
+--+-------+-------+--------+
|id|SUM(US)|SUM(UK)|SUM(Can)|
+--+-------+-------+--------+
| 1| 50| 100| 125|
| 2| 75| 150| 175|
+--+-------+-------+--------+
It's not a very elegant solution though. I had to create a chain of functions to add in all the columns. Also if I have lots of countries, I will expand my temporary data set to a very wide set with lots of zeroes.
answered Aug 4 '15 at 13:27
Al MAl M
487410
487410
add a comment |
add a comment |
Initially i adopted Al M's solution. Later took the same thought and rewrote this function as a transpose function.
This method transposes any df rows to columns of any data-format with using key and value column
for input csv
id,tag,value
1,US,50a
1,UK,100
1,Can,125
2,US,75
2,UK,150
2,Can,175
ouput
+--+---+---+---+
|id| UK| US|Can|
+--+---+---+---+
| 2|150| 75|175|
| 1|100|50a|125|
+--+---+---+---+
transpose method :
def transpose(hc : HiveContext , df: DataFrame,compositeId: List[String], key: String, value: String) =
val distinctCols = df.select(key).distinct.map r => r(0) .collect().toList
val rdd = df.map row =>
(compositeId.collect case id => row.getAs(id).asInstanceOf[Any] ,
scala.collection.mutable.Map(row.getAs(key).asInstanceOf[Any] -> row.getAs(value).asInstanceOf[Any]))
val pairRdd = rdd.reduceByKey(_ ++ _)
val rowRdd = pairRdd.map(r => dynamicRow(r, distinctCols))
hc.createDataFrame(rowRdd, getSchema(df.schema, compositeId, (key, distinctCols)))
private def dynamicRow(r: (List[Any], scala.collection.mutable.Map[Any, Any]), colNames: List[Any]) =
val cols = colNames.collect case col => r._2.getOrElse(col.toString(), null)
val array = r._1 ++ cols
Row(array: _*)
private def getSchema(srcSchema: StructType, idCols: List[String], distinctCols: (String, List[Any])): StructType =
val idSchema = idCols.map idCol => srcSchema.apply(idCol)
val colSchema = srcSchema.apply(distinctCols._1)
val colsSchema = distinctCols._2.map col => StructField(col.asInstanceOf[String], colSchema.dataType, colSchema.nullable)
StructType(idSchema ++ colsSchema)
main snippet
import java.util.Date
import org.apache.spark.SparkConf
import org.apache.spark.SparkContext
import org.apache.spark.sql.Row
import org.apache.spark.sql.DataFrame
import org.apache.spark.sql.types.StructType
import org.apache.spark.sql.hive.HiveContext
import org.apache.spark.sql.types.StructField
...
...
def main(args: Array[String]): Unit =
val sc = new SparkContext(conf)
val sqlContext = new org.apache.spark.sql.SQLContext(sc)
val dfdata1 = sqlContext.read.format("com.databricks.spark.csv").option("header", "true").option("inferSchema", "true")
.load("data.csv")
dfdata1.show()
val dfOutput = transpose(new HiveContext(sc), dfdata1, List("id"), "tag", "value")
dfOutput.show
2
This methods transposes rows to columns...
– Jaigates
Sep 6 '16 at 18:38
add a comment |
Initially i adopted Al M's solution. Later took the same thought and rewrote this function as a transpose function.
This method transposes any df rows to columns of any data-format with using key and value column
for input csv
id,tag,value
1,US,50a
1,UK,100
1,Can,125
2,US,75
2,UK,150
2,Can,175
ouput
+--+---+---+---+
|id| UK| US|Can|
+--+---+---+---+
| 2|150| 75|175|
| 1|100|50a|125|
+--+---+---+---+
transpose method :
def transpose(hc : HiveContext , df: DataFrame,compositeId: List[String], key: String, value: String) =
val distinctCols = df.select(key).distinct.map r => r(0) .collect().toList
val rdd = df.map row =>
(compositeId.collect case id => row.getAs(id).asInstanceOf[Any] ,
scala.collection.mutable.Map(row.getAs(key).asInstanceOf[Any] -> row.getAs(value).asInstanceOf[Any]))
val pairRdd = rdd.reduceByKey(_ ++ _)
val rowRdd = pairRdd.map(r => dynamicRow(r, distinctCols))
hc.createDataFrame(rowRdd, getSchema(df.schema, compositeId, (key, distinctCols)))
private def dynamicRow(r: (List[Any], scala.collection.mutable.Map[Any, Any]), colNames: List[Any]) =
val cols = colNames.collect case col => r._2.getOrElse(col.toString(), null)
val array = r._1 ++ cols
Row(array: _*)
private def getSchema(srcSchema: StructType, idCols: List[String], distinctCols: (String, List[Any])): StructType =
val idSchema = idCols.map idCol => srcSchema.apply(idCol)
val colSchema = srcSchema.apply(distinctCols._1)
val colsSchema = distinctCols._2.map col => StructField(col.asInstanceOf[String], colSchema.dataType, colSchema.nullable)
StructType(idSchema ++ colsSchema)
main snippet
import java.util.Date
import org.apache.spark.SparkConf
import org.apache.spark.SparkContext
import org.apache.spark.sql.Row
import org.apache.spark.sql.DataFrame
import org.apache.spark.sql.types.StructType
import org.apache.spark.sql.hive.HiveContext
import org.apache.spark.sql.types.StructField
...
...
def main(args: Array[String]): Unit =
val sc = new SparkContext(conf)
val sqlContext = new org.apache.spark.sql.SQLContext(sc)
val dfdata1 = sqlContext.read.format("com.databricks.spark.csv").option("header", "true").option("inferSchema", "true")
.load("data.csv")
dfdata1.show()
val dfOutput = transpose(new HiveContext(sc), dfdata1, List("id"), "tag", "value")
dfOutput.show
2
This methods transposes rows to columns...
– Jaigates
Sep 6 '16 at 18:38
add a comment |
Initially i adopted Al M's solution. Later took the same thought and rewrote this function as a transpose function.
This method transposes any df rows to columns of any data-format with using key and value column
for input csv
id,tag,value
1,US,50a
1,UK,100
1,Can,125
2,US,75
2,UK,150
2,Can,175
ouput
+--+---+---+---+
|id| UK| US|Can|
+--+---+---+---+
| 2|150| 75|175|
| 1|100|50a|125|
+--+---+---+---+
transpose method :
def transpose(hc : HiveContext , df: DataFrame,compositeId: List[String], key: String, value: String) =
val distinctCols = df.select(key).distinct.map r => r(0) .collect().toList
val rdd = df.map row =>
(compositeId.collect case id => row.getAs(id).asInstanceOf[Any] ,
scala.collection.mutable.Map(row.getAs(key).asInstanceOf[Any] -> row.getAs(value).asInstanceOf[Any]))
val pairRdd = rdd.reduceByKey(_ ++ _)
val rowRdd = pairRdd.map(r => dynamicRow(r, distinctCols))
hc.createDataFrame(rowRdd, getSchema(df.schema, compositeId, (key, distinctCols)))
private def dynamicRow(r: (List[Any], scala.collection.mutable.Map[Any, Any]), colNames: List[Any]) =
val cols = colNames.collect case col => r._2.getOrElse(col.toString(), null)
val array = r._1 ++ cols
Row(array: _*)
private def getSchema(srcSchema: StructType, idCols: List[String], distinctCols: (String, List[Any])): StructType =
val idSchema = idCols.map idCol => srcSchema.apply(idCol)
val colSchema = srcSchema.apply(distinctCols._1)
val colsSchema = distinctCols._2.map col => StructField(col.asInstanceOf[String], colSchema.dataType, colSchema.nullable)
StructType(idSchema ++ colsSchema)
main snippet
import java.util.Date
import org.apache.spark.SparkConf
import org.apache.spark.SparkContext
import org.apache.spark.sql.Row
import org.apache.spark.sql.DataFrame
import org.apache.spark.sql.types.StructType
import org.apache.spark.sql.hive.HiveContext
import org.apache.spark.sql.types.StructField
...
...
def main(args: Array[String]): Unit =
val sc = new SparkContext(conf)
val sqlContext = new org.apache.spark.sql.SQLContext(sc)
val dfdata1 = sqlContext.read.format("com.databricks.spark.csv").option("header", "true").option("inferSchema", "true")
.load("data.csv")
dfdata1.show()
val dfOutput = transpose(new HiveContext(sc), dfdata1, List("id"), "tag", "value")
dfOutput.show
Initially i adopted Al M's solution. Later took the same thought and rewrote this function as a transpose function.
This method transposes any df rows to columns of any data-format with using key and value column
for input csv
id,tag,value
1,US,50a
1,UK,100
1,Can,125
2,US,75
2,UK,150
2,Can,175
ouput
+--+---+---+---+
|id| UK| US|Can|
+--+---+---+---+
| 2|150| 75|175|
| 1|100|50a|125|
+--+---+---+---+
transpose method :
def transpose(hc : HiveContext , df: DataFrame,compositeId: List[String], key: String, value: String) =
val distinctCols = df.select(key).distinct.map r => r(0) .collect().toList
val rdd = df.map row =>
(compositeId.collect case id => row.getAs(id).asInstanceOf[Any] ,
scala.collection.mutable.Map(row.getAs(key).asInstanceOf[Any] -> row.getAs(value).asInstanceOf[Any]))
val pairRdd = rdd.reduceByKey(_ ++ _)
val rowRdd = pairRdd.map(r => dynamicRow(r, distinctCols))
hc.createDataFrame(rowRdd, getSchema(df.schema, compositeId, (key, distinctCols)))
private def dynamicRow(r: (List[Any], scala.collection.mutable.Map[Any, Any]), colNames: List[Any]) =
val cols = colNames.collect case col => r._2.getOrElse(col.toString(), null)
val array = r._1 ++ cols
Row(array: _*)
private def getSchema(srcSchema: StructType, idCols: List[String], distinctCols: (String, List[Any])): StructType =
val idSchema = idCols.map idCol => srcSchema.apply(idCol)
val colSchema = srcSchema.apply(distinctCols._1)
val colsSchema = distinctCols._2.map col => StructField(col.asInstanceOf[String], colSchema.dataType, colSchema.nullable)
StructType(idSchema ++ colsSchema)
main snippet
import java.util.Date
import org.apache.spark.SparkConf
import org.apache.spark.SparkContext
import org.apache.spark.sql.Row
import org.apache.spark.sql.DataFrame
import org.apache.spark.sql.types.StructType
import org.apache.spark.sql.hive.HiveContext
import org.apache.spark.sql.types.StructField
...
...
def main(args: Array[String]): Unit =
val sc = new SparkContext(conf)
val sqlContext = new org.apache.spark.sql.SQLContext(sc)
val dfdata1 = sqlContext.read.format("com.databricks.spark.csv").option("header", "true").option("inferSchema", "true")
.load("data.csv")
dfdata1.show()
val dfOutput = transpose(new HiveContext(sc), dfdata1, List("id"), "tag", "value")
dfOutput.show
edited Sep 6 '16 at 18:33
answered Aug 30 '16 at 18:13
JaigatesJaigates
1008
1008
2
This methods transposes rows to columns...
– Jaigates
Sep 6 '16 at 18:38
add a comment |
2
This methods transposes rows to columns...
– Jaigates
Sep 6 '16 at 18:38
2
2
This methods transposes rows to columns...
– Jaigates
Sep 6 '16 at 18:38
This methods transposes rows to columns...
– Jaigates
Sep 6 '16 at 18:38
add a comment |
There is simple and elegant solution.
scala> spark.sql("select * from k_tags limit 10").show()
+---------------+-------------+------+
| imsi| name| value|
+---------------+-------------+------+
|246021000000000| age| 37|
|246021000000000| gender|Female|
|246021000000000| arpu| 22|
|246021000000000| DeviceType| Phone|
|246021000000000|DataAllowance| 6GB|
+---------------+-------------+------+
scala> spark.sql("select * from k_tags limit 10").groupBy($"imsi").pivot("name").agg(min($"value")).show()
+---------------+-------------+----------+---+----+------+
| imsi|DataAllowance|DeviceType|age|arpu|gender|
+---------------+-------------+----------+---+----+------+
|246021000000000| 6GB| Phone| 37| 22|Female|
|246021000000001| 1GB| Phone| 72| 10| Male|
+---------------+-------------+----------+---+----+------+
add a comment |
There is simple and elegant solution.
scala> spark.sql("select * from k_tags limit 10").show()
+---------------+-------------+------+
| imsi| name| value|
+---------------+-------------+------+
|246021000000000| age| 37|
|246021000000000| gender|Female|
|246021000000000| arpu| 22|
|246021000000000| DeviceType| Phone|
|246021000000000|DataAllowance| 6GB|
+---------------+-------------+------+
scala> spark.sql("select * from k_tags limit 10").groupBy($"imsi").pivot("name").agg(min($"value")).show()
+---------------+-------------+----------+---+----+------+
| imsi|DataAllowance|DeviceType|age|arpu|gender|
+---------------+-------------+----------+---+----+------+
|246021000000000| 6GB| Phone| 37| 22|Female|
|246021000000001| 1GB| Phone| 72| 10| Male|
+---------------+-------------+----------+---+----+------+
add a comment |
There is simple and elegant solution.
scala> spark.sql("select * from k_tags limit 10").show()
+---------------+-------------+------+
| imsi| name| value|
+---------------+-------------+------+
|246021000000000| age| 37|
|246021000000000| gender|Female|
|246021000000000| arpu| 22|
|246021000000000| DeviceType| Phone|
|246021000000000|DataAllowance| 6GB|
+---------------+-------------+------+
scala> spark.sql("select * from k_tags limit 10").groupBy($"imsi").pivot("name").agg(min($"value")).show()
+---------------+-------------+----------+---+----+------+
| imsi|DataAllowance|DeviceType|age|arpu|gender|
+---------------+-------------+----------+---+----+------+
|246021000000000| 6GB| Phone| 37| 22|Female|
|246021000000001| 1GB| Phone| 72| 10| Male|
+---------------+-------------+----------+---+----+------+
There is simple and elegant solution.
scala> spark.sql("select * from k_tags limit 10").show()
+---------------+-------------+------+
| imsi| name| value|
+---------------+-------------+------+
|246021000000000| age| 37|
|246021000000000| gender|Female|
|246021000000000| arpu| 22|
|246021000000000| DeviceType| Phone|
|246021000000000|DataAllowance| 6GB|
+---------------+-------------+------+
scala> spark.sql("select * from k_tags limit 10").groupBy($"imsi").pivot("name").agg(min($"value")).show()
+---------------+-------------+----------+---+----+------+
| imsi|DataAllowance|DeviceType|age|arpu|gender|
+---------------+-------------+----------+---+----+------+
|246021000000000| 6GB| Phone| 37| 22|Female|
|246021000000001| 1GB| Phone| 72| 10| Male|
+---------------+-------------+----------+---+----+------+
edited Feb 5 '18 at 8:52


clemens
10.5k102643
10.5k102643
answered Feb 5 '18 at 8:35
MantasMantas
9
9
add a comment |
add a comment |
protected by user8371915 Jul 15 '18 at 19:20
Thank you for your interest in this question.
Because it has attracted low-quality or spam answers that had to be removed, posting an answer now requires 10 reputation on this site (the association bonus does not count).
Would you like to answer one of these unanswered questions instead?
yuVRbdv,F4cPOAEd050aY5,V,GMVze00HVefT3W8Hi
See this similar question where I posted a native Spark approach that doesn't need to know the column/category names ahead of time.
– patricksurry
Jun 23 '15 at 15:56