Should I normalize or standardize my dataset for knn?“Large data” work flows using pandasHow does WEKA IBK (KNN) algorithm lead with non-normalized attributes?Categorical and ordinal feature data difference in regression analysis?Standardization before or after categorical encoding?training a kNN algorithm with different features for each recordHow to normalize and scale data in a dataset with python?Does one-hot encoding cause issues of unbalanced feature?How to change the pipeline output from array to dataframe again with headings after normalizing?How many principal components should I choose for PCA?Do I have to do fit PCA separately for train and test data
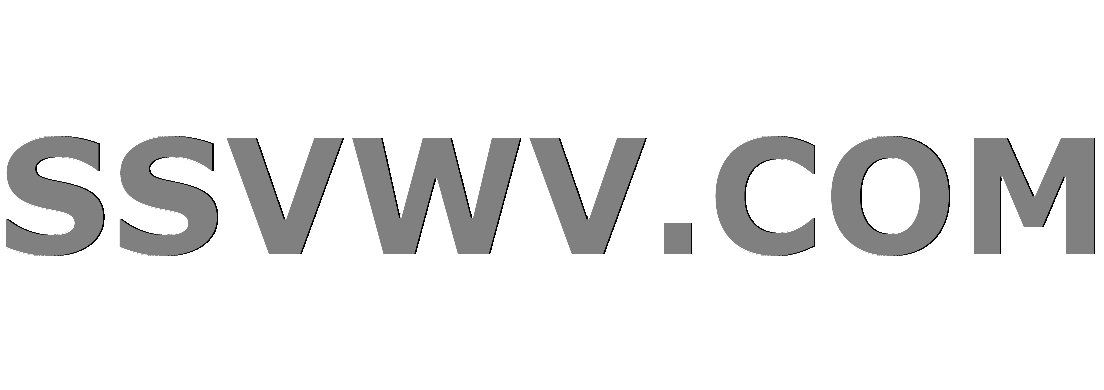
Multi tool use
Why "Having chlorophyll without photosynthesis is actually very dangerous" and "like living with a bomb"?
Why are electrically insulating heatsinks so rare? Is it just cost?
strToHex ( string to its hex representation as string)
Problem of parity - Can we draw a closed path made up of 20 line segments...
Smoothness of finite-dimensional functional calculus
Have astronauts in space suits ever taken selfies? If so, how?
How is it possible to have an ability score that is less than 3?
Watching something be written to a file live with tail
How do I create uniquely male characters?
Maximum likelihood parameters deviate from posterior distributions
Why are 150k or 200k jobs considered good when there are 300k+ births a month?
How to format long polynomial?
What's the output of a record cartridge playing an out-of-speed record
Why did the Germans forbid the possession of pet pigeons in Rostov-on-Don in 1941?
Can I ask the recruiters in my resume to put the reason why I am rejected?
Why do I get two different answers for this counting problem?
Mage Armor with Defense fighting style (for Adventurers League bladeslinger)
"to be prejudice towards/against someone" vs "to be prejudiced against/towards someone"
How to find program name(s) of an installed package?
Email Account under attack (really) - anything I can do?
Is this a crack on the carbon frame?
Minkowski space
What is the word for reserving something for yourself before others do?
TGV timetables / schedules?
Should I normalize or standardize my dataset for knn?
“Large data” work flows using pandasHow does WEKA IBK (KNN) algorithm lead with non-normalized attributes?Categorical and ordinal feature data difference in regression analysis?Standardization before or after categorical encoding?training a kNN algorithm with different features for each recordHow to normalize and scale data in a dataset with python?Does one-hot encoding cause issues of unbalanced feature?How to change the pipeline output from array to dataframe again with headings after normalizing?How many principal components should I choose for PCA?Do I have to do fit PCA separately for train and test data
.everyoneloves__top-leaderboard:empty,.everyoneloves__mid-leaderboard:empty,.everyoneloves__bot-mid-leaderboard:empty height:90px;width:728px;box-sizing:border-box;
I trying to use knn for a classification task and my dataset contains categorical features which are one hot encoded, numerical features like price etc.. and also BoW(CountVectorizer) vectors for my text column.
I know knn is affected by scaling. So I am confused what to use here?
from sklearn.preprocessing import StandardScaler
from sklearn.preprocessing import Normalizer
from sklearn.preprocessing import normalize
python python-3.x machine-learning scikit-learn knn
|
show 6 more comments
I trying to use knn for a classification task and my dataset contains categorical features which are one hot encoded, numerical features like price etc.. and also BoW(CountVectorizer) vectors for my text column.
I know knn is affected by scaling. So I am confused what to use here?
from sklearn.preprocessing import StandardScaler
from sklearn.preprocessing import Normalizer
from sklearn.preprocessing import normalize
python python-3.x machine-learning scikit-learn knn
1
StandardScaler
for numerical features should be enough.
– Sergey Bushmanov
Mar 9 at 3:10
@SergeyBushmanov Many rows in the price column are zeros? Can I still standardize using standardscaler?
– user214
Mar 9 at 3:34
1
Your observation that many prices are zeroes may lead you to an other feature preprocessing pipeline, but in general, one would applyStandardScaler
on numerical features with differing scale. This is important for KNN
– Sergey Bushmanov
Mar 9 at 4:07
@SergeyBushmanov I've a small query. You mentioned to standardize only my numerical features but I've applied PCA on my BoW features and I was thinking whether I should standardize them as well along with numerical features and leave out categorical features.
– user214
Mar 9 at 17:42
BoW are already well behaving features. I would guess you do not need to standardize them. However, if you wish to you can always cross validate if that makes sense.
– Sergey Bushmanov
Mar 9 at 17:52
|
show 6 more comments
I trying to use knn for a classification task and my dataset contains categorical features which are one hot encoded, numerical features like price etc.. and also BoW(CountVectorizer) vectors for my text column.
I know knn is affected by scaling. So I am confused what to use here?
from sklearn.preprocessing import StandardScaler
from sklearn.preprocessing import Normalizer
from sklearn.preprocessing import normalize
python python-3.x machine-learning scikit-learn knn
I trying to use knn for a classification task and my dataset contains categorical features which are one hot encoded, numerical features like price etc.. and also BoW(CountVectorizer) vectors for my text column.
I know knn is affected by scaling. So I am confused what to use here?
from sklearn.preprocessing import StandardScaler
from sklearn.preprocessing import Normalizer
from sklearn.preprocessing import normalize
python python-3.x machine-learning scikit-learn knn
python python-3.x machine-learning scikit-learn knn
asked Mar 9 at 2:26
user214user214
533115
533115
1
StandardScaler
for numerical features should be enough.
– Sergey Bushmanov
Mar 9 at 3:10
@SergeyBushmanov Many rows in the price column are zeros? Can I still standardize using standardscaler?
– user214
Mar 9 at 3:34
1
Your observation that many prices are zeroes may lead you to an other feature preprocessing pipeline, but in general, one would applyStandardScaler
on numerical features with differing scale. This is important for KNN
– Sergey Bushmanov
Mar 9 at 4:07
@SergeyBushmanov I've a small query. You mentioned to standardize only my numerical features but I've applied PCA on my BoW features and I was thinking whether I should standardize them as well along with numerical features and leave out categorical features.
– user214
Mar 9 at 17:42
BoW are already well behaving features. I would guess you do not need to standardize them. However, if you wish to you can always cross validate if that makes sense.
– Sergey Bushmanov
Mar 9 at 17:52
|
show 6 more comments
1
StandardScaler
for numerical features should be enough.
– Sergey Bushmanov
Mar 9 at 3:10
@SergeyBushmanov Many rows in the price column are zeros? Can I still standardize using standardscaler?
– user214
Mar 9 at 3:34
1
Your observation that many prices are zeroes may lead you to an other feature preprocessing pipeline, but in general, one would applyStandardScaler
on numerical features with differing scale. This is important for KNN
– Sergey Bushmanov
Mar 9 at 4:07
@SergeyBushmanov I've a small query. You mentioned to standardize only my numerical features but I've applied PCA on my BoW features and I was thinking whether I should standardize them as well along with numerical features and leave out categorical features.
– user214
Mar 9 at 17:42
BoW are already well behaving features. I would guess you do not need to standardize them. However, if you wish to you can always cross validate if that makes sense.
– Sergey Bushmanov
Mar 9 at 17:52
1
1
StandardScaler
for numerical features should be enough.– Sergey Bushmanov
Mar 9 at 3:10
StandardScaler
for numerical features should be enough.– Sergey Bushmanov
Mar 9 at 3:10
@SergeyBushmanov Many rows in the price column are zeros? Can I still standardize using standardscaler?
– user214
Mar 9 at 3:34
@SergeyBushmanov Many rows in the price column are zeros? Can I still standardize using standardscaler?
– user214
Mar 9 at 3:34
1
1
Your observation that many prices are zeroes may lead you to an other feature preprocessing pipeline, but in general, one would apply
StandardScaler
on numerical features with differing scale. This is important for KNN– Sergey Bushmanov
Mar 9 at 4:07
Your observation that many prices are zeroes may lead you to an other feature preprocessing pipeline, but in general, one would apply
StandardScaler
on numerical features with differing scale. This is important for KNN– Sergey Bushmanov
Mar 9 at 4:07
@SergeyBushmanov I've a small query. You mentioned to standardize only my numerical features but I've applied PCA on my BoW features and I was thinking whether I should standardize them as well along with numerical features and leave out categorical features.
– user214
Mar 9 at 17:42
@SergeyBushmanov I've a small query. You mentioned to standardize only my numerical features but I've applied PCA on my BoW features and I was thinking whether I should standardize them as well along with numerical features and leave out categorical features.
– user214
Mar 9 at 17:42
BoW are already well behaving features. I would guess you do not need to standardize them. However, if you wish to you can always cross validate if that makes sense.
– Sergey Bushmanov
Mar 9 at 17:52
BoW are already well behaving features. I would guess you do not need to standardize them. However, if you wish to you can always cross validate if that makes sense.
– Sergey Bushmanov
Mar 9 at 17:52
|
show 6 more comments
1 Answer
1
active
oldest
votes
My suggestion would be to go for MinMaxScaler
One of the major reason is that your features such as price can't have negative values and as you mentioned, it could be sparse.
From Documentation:
The motivation to use this scaling include robustness to very small
standard deviations of features and preserving zero entries in sparse
data.
At the same time, if your numerical variable has a huge variance, then go for RobustScaler or StandardScaler.
You dont have to scale the one hot encoded features.
For BoW, it is important to preserve the sparsity of the data. If you apply the StandardScaler, you will lose the sparsity. You definitely have to go for MinMaxScaler.
Another option would be to go for TfidfVectorizer, which does the l2 normalization by default.
1
Can I know what you meant by preserving the sparsity of the data. Actually I've used PCA on my BoW and transformed into fewer dimension. So can I now apply scaling to it or let it be.
– user214
Mar 9 at 13:44
1
preserving the sparsity of the data
means zeros in the features would be kept as zeros even after Normalization. Sparsity simply means having less number of nonzero values. BoW usually have a lot zeros because every document does not contain all the words in the vocabulary.
– ai_learning
Mar 9 at 13:47
1
yes, you can apply scaling on pca features.
– ai_learning
Mar 9 at 13:49
add a comment |
Your Answer
StackExchange.ifUsing("editor", function ()
StackExchange.using("externalEditor", function ()
StackExchange.using("snippets", function ()
StackExchange.snippets.init();
);
);
, "code-snippets");
StackExchange.ready(function()
var channelOptions =
tags: "".split(" "),
id: "1"
;
initTagRenderer("".split(" "), "".split(" "), channelOptions);
StackExchange.using("externalEditor", function()
// Have to fire editor after snippets, if snippets enabled
if (StackExchange.settings.snippets.snippetsEnabled)
StackExchange.using("snippets", function()
createEditor();
);
else
createEditor();
);
function createEditor()
StackExchange.prepareEditor(
heartbeatType: 'answer',
autoActivateHeartbeat: false,
convertImagesToLinks: true,
noModals: true,
showLowRepImageUploadWarning: true,
reputationToPostImages: 10,
bindNavPrevention: true,
postfix: "",
imageUploader:
brandingHtml: "Powered by u003ca class="icon-imgur-white" href="https://imgur.com/"u003eu003c/au003e",
contentPolicyHtml: "User contributions licensed under u003ca href="https://creativecommons.org/licenses/by-sa/3.0/"u003ecc by-sa 3.0 with attribution requiredu003c/au003e u003ca href="https://stackoverflow.com/legal/content-policy"u003e(content policy)u003c/au003e",
allowUrls: true
,
onDemand: true,
discardSelector: ".discard-answer"
,immediatelyShowMarkdownHelp:true
);
);
Sign up or log in
StackExchange.ready(function ()
StackExchange.helpers.onClickDraftSave('#login-link');
);
Sign up using Google
Sign up using Facebook
Sign up using Email and Password
Post as a guest
Required, but never shown
StackExchange.ready(
function ()
StackExchange.openid.initPostLogin('.new-post-login', 'https%3a%2f%2fstackoverflow.com%2fquestions%2f55073423%2fshould-i-normalize-or-standardize-my-dataset-for-knn%23new-answer', 'question_page');
);
Post as a guest
Required, but never shown
1 Answer
1
active
oldest
votes
1 Answer
1
active
oldest
votes
active
oldest
votes
active
oldest
votes
My suggestion would be to go for MinMaxScaler
One of the major reason is that your features such as price can't have negative values and as you mentioned, it could be sparse.
From Documentation:
The motivation to use this scaling include robustness to very small
standard deviations of features and preserving zero entries in sparse
data.
At the same time, if your numerical variable has a huge variance, then go for RobustScaler or StandardScaler.
You dont have to scale the one hot encoded features.
For BoW, it is important to preserve the sparsity of the data. If you apply the StandardScaler, you will lose the sparsity. You definitely have to go for MinMaxScaler.
Another option would be to go for TfidfVectorizer, which does the l2 normalization by default.
1
Can I know what you meant by preserving the sparsity of the data. Actually I've used PCA on my BoW and transformed into fewer dimension. So can I now apply scaling to it or let it be.
– user214
Mar 9 at 13:44
1
preserving the sparsity of the data
means zeros in the features would be kept as zeros even after Normalization. Sparsity simply means having less number of nonzero values. BoW usually have a lot zeros because every document does not contain all the words in the vocabulary.
– ai_learning
Mar 9 at 13:47
1
yes, you can apply scaling on pca features.
– ai_learning
Mar 9 at 13:49
add a comment |
My suggestion would be to go for MinMaxScaler
One of the major reason is that your features such as price can't have negative values and as you mentioned, it could be sparse.
From Documentation:
The motivation to use this scaling include robustness to very small
standard deviations of features and preserving zero entries in sparse
data.
At the same time, if your numerical variable has a huge variance, then go for RobustScaler or StandardScaler.
You dont have to scale the one hot encoded features.
For BoW, it is important to preserve the sparsity of the data. If you apply the StandardScaler, you will lose the sparsity. You definitely have to go for MinMaxScaler.
Another option would be to go for TfidfVectorizer, which does the l2 normalization by default.
1
Can I know what you meant by preserving the sparsity of the data. Actually I've used PCA on my BoW and transformed into fewer dimension. So can I now apply scaling to it or let it be.
– user214
Mar 9 at 13:44
1
preserving the sparsity of the data
means zeros in the features would be kept as zeros even after Normalization. Sparsity simply means having less number of nonzero values. BoW usually have a lot zeros because every document does not contain all the words in the vocabulary.
– ai_learning
Mar 9 at 13:47
1
yes, you can apply scaling on pca features.
– ai_learning
Mar 9 at 13:49
add a comment |
My suggestion would be to go for MinMaxScaler
One of the major reason is that your features such as price can't have negative values and as you mentioned, it could be sparse.
From Documentation:
The motivation to use this scaling include robustness to very small
standard deviations of features and preserving zero entries in sparse
data.
At the same time, if your numerical variable has a huge variance, then go for RobustScaler or StandardScaler.
You dont have to scale the one hot encoded features.
For BoW, it is important to preserve the sparsity of the data. If you apply the StandardScaler, you will lose the sparsity. You definitely have to go for MinMaxScaler.
Another option would be to go for TfidfVectorizer, which does the l2 normalization by default.
My suggestion would be to go for MinMaxScaler
One of the major reason is that your features such as price can't have negative values and as you mentioned, it could be sparse.
From Documentation:
The motivation to use this scaling include robustness to very small
standard deviations of features and preserving zero entries in sparse
data.
At the same time, if your numerical variable has a huge variance, then go for RobustScaler or StandardScaler.
You dont have to scale the one hot encoded features.
For BoW, it is important to preserve the sparsity of the data. If you apply the StandardScaler, you will lose the sparsity. You definitely have to go for MinMaxScaler.
Another option would be to go for TfidfVectorizer, which does the l2 normalization by default.
answered Mar 9 at 8:43


ai_learningai_learning
4,27521036
4,27521036
1
Can I know what you meant by preserving the sparsity of the data. Actually I've used PCA on my BoW and transformed into fewer dimension. So can I now apply scaling to it or let it be.
– user214
Mar 9 at 13:44
1
preserving the sparsity of the data
means zeros in the features would be kept as zeros even after Normalization. Sparsity simply means having less number of nonzero values. BoW usually have a lot zeros because every document does not contain all the words in the vocabulary.
– ai_learning
Mar 9 at 13:47
1
yes, you can apply scaling on pca features.
– ai_learning
Mar 9 at 13:49
add a comment |
1
Can I know what you meant by preserving the sparsity of the data. Actually I've used PCA on my BoW and transformed into fewer dimension. So can I now apply scaling to it or let it be.
– user214
Mar 9 at 13:44
1
preserving the sparsity of the data
means zeros in the features would be kept as zeros even after Normalization. Sparsity simply means having less number of nonzero values. BoW usually have a lot zeros because every document does not contain all the words in the vocabulary.
– ai_learning
Mar 9 at 13:47
1
yes, you can apply scaling on pca features.
– ai_learning
Mar 9 at 13:49
1
1
Can I know what you meant by preserving the sparsity of the data. Actually I've used PCA on my BoW and transformed into fewer dimension. So can I now apply scaling to it or let it be.
– user214
Mar 9 at 13:44
Can I know what you meant by preserving the sparsity of the data. Actually I've used PCA on my BoW and transformed into fewer dimension. So can I now apply scaling to it or let it be.
– user214
Mar 9 at 13:44
1
1
preserving the sparsity of the data
means zeros in the features would be kept as zeros even after Normalization. Sparsity simply means having less number of nonzero values. BoW usually have a lot zeros because every document does not contain all the words in the vocabulary.– ai_learning
Mar 9 at 13:47
preserving the sparsity of the data
means zeros in the features would be kept as zeros even after Normalization. Sparsity simply means having less number of nonzero values. BoW usually have a lot zeros because every document does not contain all the words in the vocabulary.– ai_learning
Mar 9 at 13:47
1
1
yes, you can apply scaling on pca features.
– ai_learning
Mar 9 at 13:49
yes, you can apply scaling on pca features.
– ai_learning
Mar 9 at 13:49
add a comment |
Thanks for contributing an answer to Stack Overflow!
- Please be sure to answer the question. Provide details and share your research!
But avoid …
- Asking for help, clarification, or responding to other answers.
- Making statements based on opinion; back them up with references or personal experience.
To learn more, see our tips on writing great answers.
Sign up or log in
StackExchange.ready(function ()
StackExchange.helpers.onClickDraftSave('#login-link');
);
Sign up using Google
Sign up using Facebook
Sign up using Email and Password
Post as a guest
Required, but never shown
StackExchange.ready(
function ()
StackExchange.openid.initPostLogin('.new-post-login', 'https%3a%2f%2fstackoverflow.com%2fquestions%2f55073423%2fshould-i-normalize-or-standardize-my-dataset-for-knn%23new-answer', 'question_page');
);
Post as a guest
Required, but never shown
Sign up or log in
StackExchange.ready(function ()
StackExchange.helpers.onClickDraftSave('#login-link');
);
Sign up using Google
Sign up using Facebook
Sign up using Email and Password
Post as a guest
Required, but never shown
Sign up or log in
StackExchange.ready(function ()
StackExchange.helpers.onClickDraftSave('#login-link');
);
Sign up using Google
Sign up using Facebook
Sign up using Email and Password
Post as a guest
Required, but never shown
Sign up or log in
StackExchange.ready(function ()
StackExchange.helpers.onClickDraftSave('#login-link');
);
Sign up using Google
Sign up using Facebook
Sign up using Email and Password
Sign up using Google
Sign up using Facebook
Sign up using Email and Password
Post as a guest
Required, but never shown
Required, but never shown
Required, but never shown
Required, but never shown
Required, but never shown
Required, but never shown
Required, but never shown
Required, but never shown
Required, but never shown
FAU1ogq92 Lun,A0wJajrH0q lp Iog5 GLAz099c2AFPs9l L2iEmu,mVj,zSpO65xRVmNR2,9QTG8Esmlij3O2YThZw 6H
1
StandardScaler
for numerical features should be enough.– Sergey Bushmanov
Mar 9 at 3:10
@SergeyBushmanov Many rows in the price column are zeros? Can I still standardize using standardscaler?
– user214
Mar 9 at 3:34
1
Your observation that many prices are zeroes may lead you to an other feature preprocessing pipeline, but in general, one would apply
StandardScaler
on numerical features with differing scale. This is important for KNN– Sergey Bushmanov
Mar 9 at 4:07
@SergeyBushmanov I've a small query. You mentioned to standardize only my numerical features but I've applied PCA on my BoW features and I was thinking whether I should standardize them as well along with numerical features and leave out categorical features.
– user214
Mar 9 at 17:42
BoW are already well behaving features. I would guess you do not need to standardize them. However, if you wish to you can always cross validate if that makes sense.
– Sergey Bushmanov
Mar 9 at 17:52