How to use Sparklyr to summarize Categorical Variable LevelSparklyr/Dplyr frequencies for each categorical columnGather in sparklyrSparklyr: how to center a Spark table based on column?How to sort a dataframe by multiple column(s)?How to make a great R reproducible exampleGenerate two categorical variables with a chosen degree of association in RSparkR vs sparklyrCreating dummy variables in sparklyr?Sparklyr handing categorical variablesHow to find out the categorical variables in RCount occurrences in dataframe with two conditions without for loopGather in sparklyrHow to use dplyr in sparklyr
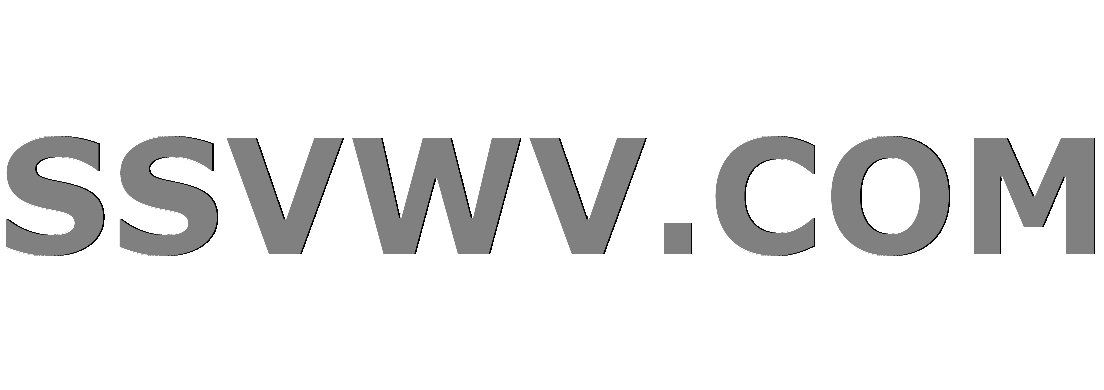
Multi tool use
Limit max CPU usage SQL SERVER with WSRM
How can I safely use "Thalidomide" in my novel while respecting the trademark?
Quoting Keynes in a lecture
How to get directions in deep space?
Can I run 125kHz RF circuit on a breadboard?
How do I tell my boss that I'm quitting in 15 days (a colleague left this week)
How to leave product feedback on macOS?
Grepping string, but include all non-blank lines following each grep match
Pre-Employment Background Check With Consent For Future Checks
I'm just a whisper. Who am I?
Visualizing the difference curve in a 2D plot?
How to test the sharpness of a knife?
Can I cause damage to electrical appliances by unplugging them when they are turned on?
In One Punch Man, is King actually weak?
Check if object is null and return null
Can I say "fingers" when referring to toes?
How would you translate "more" for use as an interface button?
Why is the Sun approximated as a black body at ~ 5800 K?
Should I assume I have passed probation?
How to preserve electronics (computers, iPads and phones) for hundreds of years
What's the name of the logical fallacy where a debater extends a statement far beyond the original statement to make it true?
Do I have to take mana from my deck or hand when tapping a dual land?
Is there a RAID 0 Equivalent for RAM?
What should be the ideal length of sentences in a blog post for ease of reading?
How to use Sparklyr to summarize Categorical Variable Level
Sparklyr/Dplyr frequencies for each categorical columnGather in sparklyrSparklyr: how to center a Spark table based on column?How to sort a dataframe by multiple column(s)?How to make a great R reproducible exampleGenerate two categorical variables with a chosen degree of association in RSparkR vs sparklyrCreating dummy variables in sparklyr?Sparklyr handing categorical variablesHow to find out the categorical variables in RCount occurrences in dataframe with two conditions without for loopGather in sparklyrHow to use dplyr in sparklyr
For each categorical variable in dataset, I want to get counts and summary stats for each level. I can do this using dlookr R package using their diagnose_category()
function. Since at work I don't have that package I recreated the function using dplyr.
In sparklye I am able to get counts for a single variable at a time. Need help to extend it all categorical variable.
Need Help:
Implement the function via SparklyR
Table 1: Final output needed:
# A tibble: 20 x 6
variables levels N freq ratio rank
<chr> <ord> <int> <int> <dbl> <int>
1 cut Ideal 53940 21551 40.0 1
2 cut Premium 53940 13791 25.6 2
3 cut Very Good 53940 12082 22.4 3
4 cut Good 53940 4906 9.10 4
5 cut Fair 53940 1610 2.98 5
6 color G 53940 11292 20.9 1
7 color E 53940 9797 18.2 2
8 color F 53940 9542 17.7 3
9 color H 53940 8304 15.4 4
10 color D 53940 6775 12.6 5
11 color I 53940 5422 10.1 6
12 color J 53940 2808 5.21 7
13 clarity SI1 53940 13065 24.2 1
14 clarity VS2 53940 12258 22.7 2
15 clarity SI2 53940 9194 17.0 3
16 clarity VS1 53940 8171 15.1 4
17 clarity VVS2 53940 5066 9.39 5
18 clarity VVS1 53940 3655 6.78 6
19 clarity IF 53940 1790 3.32 7
20 clarity I1 53940 741 1.37 8
R Code:
# Categorical Variable Profile
# Table based on dlookr package, diagnose_category() function
# variables : variable names
# types: the data type of the variable
# levels: level names
# N : Number of observation
# freq : Number of observation at the level
# ratio : Percentage of observation at the level
# rank : Rank of occupancy ratio of levels
library(ggplot2)
library(dplyr)
library(tidyr)
library(purrr)
library(tibble)
library(stringr)
# Helper Function
cat_level_summary <- function(df,x)
count(df,x, sort = TRUE) %>%
transmute(levels = x, N = sum(n), freq = n,
ratio = n / sum(n) * 100, rank = row_number())
# Loading
diamonds_tbl <- diamonds
# Main Code
CategoricalVariableProfile <- diamonds_tbl %>%
select_if(~!is.numeric(.)) %>%
map(~cat_level_summary(data.frame(x=.x), x)) %>%
do.call(rbind.data.frame, .) %>%
rownames_to_column(., "variables")%>%
mutate(variables = str_match(variables, ".*(?=\.)")[, 1] )
Spark Code:
#Spark data Table
diamonds_tbl <- copy_to(sc, diamonds, "diamonds", overwrite = TRUE)
CategoricalVariableProfile <- diamonds_tbl %>%
group_by(cut) %>%
summarize(count = n()) %>%
sdf_register("CategoricalVariableProfile")
r apache-spark dplyr sparklyr
add a comment |
For each categorical variable in dataset, I want to get counts and summary stats for each level. I can do this using dlookr R package using their diagnose_category()
function. Since at work I don't have that package I recreated the function using dplyr.
In sparklye I am able to get counts for a single variable at a time. Need help to extend it all categorical variable.
Need Help:
Implement the function via SparklyR
Table 1: Final output needed:
# A tibble: 20 x 6
variables levels N freq ratio rank
<chr> <ord> <int> <int> <dbl> <int>
1 cut Ideal 53940 21551 40.0 1
2 cut Premium 53940 13791 25.6 2
3 cut Very Good 53940 12082 22.4 3
4 cut Good 53940 4906 9.10 4
5 cut Fair 53940 1610 2.98 5
6 color G 53940 11292 20.9 1
7 color E 53940 9797 18.2 2
8 color F 53940 9542 17.7 3
9 color H 53940 8304 15.4 4
10 color D 53940 6775 12.6 5
11 color I 53940 5422 10.1 6
12 color J 53940 2808 5.21 7
13 clarity SI1 53940 13065 24.2 1
14 clarity VS2 53940 12258 22.7 2
15 clarity SI2 53940 9194 17.0 3
16 clarity VS1 53940 8171 15.1 4
17 clarity VVS2 53940 5066 9.39 5
18 clarity VVS1 53940 3655 6.78 6
19 clarity IF 53940 1790 3.32 7
20 clarity I1 53940 741 1.37 8
R Code:
# Categorical Variable Profile
# Table based on dlookr package, diagnose_category() function
# variables : variable names
# types: the data type of the variable
# levels: level names
# N : Number of observation
# freq : Number of observation at the level
# ratio : Percentage of observation at the level
# rank : Rank of occupancy ratio of levels
library(ggplot2)
library(dplyr)
library(tidyr)
library(purrr)
library(tibble)
library(stringr)
# Helper Function
cat_level_summary <- function(df,x)
count(df,x, sort = TRUE) %>%
transmute(levels = x, N = sum(n), freq = n,
ratio = n / sum(n) * 100, rank = row_number())
# Loading
diamonds_tbl <- diamonds
# Main Code
CategoricalVariableProfile <- diamonds_tbl %>%
select_if(~!is.numeric(.)) %>%
map(~cat_level_summary(data.frame(x=.x), x)) %>%
do.call(rbind.data.frame, .) %>%
rownames_to_column(., "variables")%>%
mutate(variables = str_match(variables, ".*(?=\.)")[, 1] )
Spark Code:
#Spark data Table
diamonds_tbl <- copy_to(sc, diamonds, "diamonds", overwrite = TRUE)
CategoricalVariableProfile <- diamonds_tbl %>%
group_by(cut) %>%
summarize(count = n()) %>%
sdf_register("CategoricalVariableProfile")
r apache-spark dplyr sparklyr
add a comment |
For each categorical variable in dataset, I want to get counts and summary stats for each level. I can do this using dlookr R package using their diagnose_category()
function. Since at work I don't have that package I recreated the function using dplyr.
In sparklye I am able to get counts for a single variable at a time. Need help to extend it all categorical variable.
Need Help:
Implement the function via SparklyR
Table 1: Final output needed:
# A tibble: 20 x 6
variables levels N freq ratio rank
<chr> <ord> <int> <int> <dbl> <int>
1 cut Ideal 53940 21551 40.0 1
2 cut Premium 53940 13791 25.6 2
3 cut Very Good 53940 12082 22.4 3
4 cut Good 53940 4906 9.10 4
5 cut Fair 53940 1610 2.98 5
6 color G 53940 11292 20.9 1
7 color E 53940 9797 18.2 2
8 color F 53940 9542 17.7 3
9 color H 53940 8304 15.4 4
10 color D 53940 6775 12.6 5
11 color I 53940 5422 10.1 6
12 color J 53940 2808 5.21 7
13 clarity SI1 53940 13065 24.2 1
14 clarity VS2 53940 12258 22.7 2
15 clarity SI2 53940 9194 17.0 3
16 clarity VS1 53940 8171 15.1 4
17 clarity VVS2 53940 5066 9.39 5
18 clarity VVS1 53940 3655 6.78 6
19 clarity IF 53940 1790 3.32 7
20 clarity I1 53940 741 1.37 8
R Code:
# Categorical Variable Profile
# Table based on dlookr package, diagnose_category() function
# variables : variable names
# types: the data type of the variable
# levels: level names
# N : Number of observation
# freq : Number of observation at the level
# ratio : Percentage of observation at the level
# rank : Rank of occupancy ratio of levels
library(ggplot2)
library(dplyr)
library(tidyr)
library(purrr)
library(tibble)
library(stringr)
# Helper Function
cat_level_summary <- function(df,x)
count(df,x, sort = TRUE) %>%
transmute(levels = x, N = sum(n), freq = n,
ratio = n / sum(n) * 100, rank = row_number())
# Loading
diamonds_tbl <- diamonds
# Main Code
CategoricalVariableProfile <- diamonds_tbl %>%
select_if(~!is.numeric(.)) %>%
map(~cat_level_summary(data.frame(x=.x), x)) %>%
do.call(rbind.data.frame, .) %>%
rownames_to_column(., "variables")%>%
mutate(variables = str_match(variables, ".*(?=\.)")[, 1] )
Spark Code:
#Spark data Table
diamonds_tbl <- copy_to(sc, diamonds, "diamonds", overwrite = TRUE)
CategoricalVariableProfile <- diamonds_tbl %>%
group_by(cut) %>%
summarize(count = n()) %>%
sdf_register("CategoricalVariableProfile")
r apache-spark dplyr sparklyr
For each categorical variable in dataset, I want to get counts and summary stats for each level. I can do this using dlookr R package using their diagnose_category()
function. Since at work I don't have that package I recreated the function using dplyr.
In sparklye I am able to get counts for a single variable at a time. Need help to extend it all categorical variable.
Need Help:
Implement the function via SparklyR
Table 1: Final output needed:
# A tibble: 20 x 6
variables levels N freq ratio rank
<chr> <ord> <int> <int> <dbl> <int>
1 cut Ideal 53940 21551 40.0 1
2 cut Premium 53940 13791 25.6 2
3 cut Very Good 53940 12082 22.4 3
4 cut Good 53940 4906 9.10 4
5 cut Fair 53940 1610 2.98 5
6 color G 53940 11292 20.9 1
7 color E 53940 9797 18.2 2
8 color F 53940 9542 17.7 3
9 color H 53940 8304 15.4 4
10 color D 53940 6775 12.6 5
11 color I 53940 5422 10.1 6
12 color J 53940 2808 5.21 7
13 clarity SI1 53940 13065 24.2 1
14 clarity VS2 53940 12258 22.7 2
15 clarity SI2 53940 9194 17.0 3
16 clarity VS1 53940 8171 15.1 4
17 clarity VVS2 53940 5066 9.39 5
18 clarity VVS1 53940 3655 6.78 6
19 clarity IF 53940 1790 3.32 7
20 clarity I1 53940 741 1.37 8
R Code:
# Categorical Variable Profile
# Table based on dlookr package, diagnose_category() function
# variables : variable names
# types: the data type of the variable
# levels: level names
# N : Number of observation
# freq : Number of observation at the level
# ratio : Percentage of observation at the level
# rank : Rank of occupancy ratio of levels
library(ggplot2)
library(dplyr)
library(tidyr)
library(purrr)
library(tibble)
library(stringr)
# Helper Function
cat_level_summary <- function(df,x)
count(df,x, sort = TRUE) %>%
transmute(levels = x, N = sum(n), freq = n,
ratio = n / sum(n) * 100, rank = row_number())
# Loading
diamonds_tbl <- diamonds
# Main Code
CategoricalVariableProfile <- diamonds_tbl %>%
select_if(~!is.numeric(.)) %>%
map(~cat_level_summary(data.frame(x=.x), x)) %>%
do.call(rbind.data.frame, .) %>%
rownames_to_column(., "variables")%>%
mutate(variables = str_match(variables, ".*(?=\.)")[, 1] )
Spark Code:
#Spark data Table
diamonds_tbl <- copy_to(sc, diamonds, "diamonds", overwrite = TRUE)
CategoricalVariableProfile <- diamonds_tbl %>%
group_by(cut) %>%
summarize(count = n()) %>%
sdf_register("CategoricalVariableProfile")
r apache-spark dplyr sparklyr
r apache-spark dplyr sparklyr
edited Jan 26 at 0:23
user6910411
35.3k1089108
35.3k1089108
asked Jan 25 at 22:57


amitkb3amitkb3
193111
193111
add a comment |
add a comment |
1 Answer
1
active
oldest
votes
Flatten your data using sdf_gather
:
long <- diamonds_tbl %>%
select(cut, color, clarity) %>%
sdf_gather("variable", "level", "cut", "color", "clarity")
Aggregate by variable
and level
:
counts <- long %>% group_by(variable, level) %>% summarise(freq = n())
And finally apply required window functions:
result <- counts %>%
arrange(-freq) %>%
mutate(
rank = rank(),
total = sum(freq, na.rm = TRUE),
ratio = freq / total * 100)
Which will give you
result
# Source: spark<?> [?? x 6]
# Groups: variable
# Ordered by: -freq
variable level freq rank total ratio
<chr> <chr> <dbl> <int> <dbl> <dbl>
1 cut Ideal 21551 1 53940 40.0
2 cut Premium 13791 2 53940 25.6
3 cut Very Good 12082 3 53940 22.4
4 cut Good 4906 4 53940 9.10
5 cut Fair 1610 5 53940 2.98
6 clarity SI1 13065 1 53940 24.2
7 clarity VS2 12258 2 53940 22.7
8 clarity SI2 9194 3 53940 17.0
9 clarity VS1 8171 4 53940 15.1
10 clarity VVS2 5066 5 53940 9.39
# … with more rows
with following optimized plan
optimizedPlan(result)
<jobj[165]>
org.apache.spark.sql.catalyst.plans.logical.Project
Project [variable#524, level#525, freq#1478L, rank#1479, total#1480L, ((cast(freq#1478L as double) / cast(total#1480L as double)) * 100.0) AS ratio#1481]
+- Window [rank(_w1#1493L) windowspecdefinition(variable#524, _w1#1493L ASC NULLS FIRST, specifiedwindowframe(RowFrame, unboundedpreceding$(), currentrow$())) AS rank#1479], [variable#524], [_w1#1493L ASC NULLS FIRST]
+- Window [sum(freq#1478L) windowspecdefinition(variable#524, specifiedwindowframe(RowFrame, unboundedpreceding$(), unboundedfollowing$())) AS total#1480L], [variable#524]
+- Project [variable#524, level#525, freq#1478L, -freq#1478L AS _w1#1493L]
+- Sort [-freq#1478L ASC NULLS FIRST], true
+- Aggregate [variable#524, level#525], [variable#524, level#525, count(1) AS freq#1478L]
+- Generate explode(map(cut, cut#19, color, color#20, clarity, clarity#21)), [0, 1, 2], false, [variable#524, level#525]
+- Project [cut#19, color#20, clarity#21]
+- InMemoryRelation [carat#18, cut#19, color#20, clarity#21, depth#22, table#23, price#24, x#25, y#26, z#27], StorageLevel(disk, memory, deserialized, 1 replicas)
+- Scan ExistingRDD[carat#18,cut#19,color#20,clarity#21,depth#22,table#23,price#24,x#25,y#26,z#27]
and query (sdf_gather
component not included):
dbplyr::remote_query(result)
<SQL> SELECT `variable`, `level`, `freq`, `rank`, `total`, `freq` / `total` * 100.0 AS `ratio`
FROM (SELECT `variable`, `level`, `freq`, rank() OVER (PARTITION BY `variable` ORDER BY -`freq`) AS `rank`, sum(`freq`) OVER (PARTITION BY `variable`) AS `total`
FROM (SELECT *
FROM (SELECT `variable`, `level`, count(*) AS `freq`
FROM `sparklyr_tmp_ded2576b9f1`
GROUP BY `variable`, `level`) `dsbksdfhtf`
ORDER BY -`freq`) `obyrzsxeus`) `ekejqyjrfz`
add a comment |
Your Answer
StackExchange.ifUsing("editor", function ()
StackExchange.using("externalEditor", function ()
StackExchange.using("snippets", function ()
StackExchange.snippets.init();
);
);
, "code-snippets");
StackExchange.ready(function()
var channelOptions =
tags: "".split(" "),
id: "1"
;
initTagRenderer("".split(" "), "".split(" "), channelOptions);
StackExchange.using("externalEditor", function()
// Have to fire editor after snippets, if snippets enabled
if (StackExchange.settings.snippets.snippetsEnabled)
StackExchange.using("snippets", function()
createEditor();
);
else
createEditor();
);
function createEditor()
StackExchange.prepareEditor(
heartbeatType: 'answer',
autoActivateHeartbeat: false,
convertImagesToLinks: true,
noModals: true,
showLowRepImageUploadWarning: true,
reputationToPostImages: 10,
bindNavPrevention: true,
postfix: "",
imageUploader:
brandingHtml: "Powered by u003ca class="icon-imgur-white" href="https://imgur.com/"u003eu003c/au003e",
contentPolicyHtml: "User contributions licensed under u003ca href="https://creativecommons.org/licenses/by-sa/3.0/"u003ecc by-sa 3.0 with attribution requiredu003c/au003e u003ca href="https://stackoverflow.com/legal/content-policy"u003e(content policy)u003c/au003e",
allowUrls: true
,
onDemand: true,
discardSelector: ".discard-answer"
,immediatelyShowMarkdownHelp:true
);
);
Sign up or log in
StackExchange.ready(function ()
StackExchange.helpers.onClickDraftSave('#login-link');
);
Sign up using Google
Sign up using Facebook
Sign up using Email and Password
Post as a guest
Required, but never shown
StackExchange.ready(
function ()
StackExchange.openid.initPostLogin('.new-post-login', 'https%3a%2f%2fstackoverflow.com%2fquestions%2f54373886%2fhow-to-use-sparklyr-to-summarize-categorical-variable-level%23new-answer', 'question_page');
);
Post as a guest
Required, but never shown
1 Answer
1
active
oldest
votes
1 Answer
1
active
oldest
votes
active
oldest
votes
active
oldest
votes
Flatten your data using sdf_gather
:
long <- diamonds_tbl %>%
select(cut, color, clarity) %>%
sdf_gather("variable", "level", "cut", "color", "clarity")
Aggregate by variable
and level
:
counts <- long %>% group_by(variable, level) %>% summarise(freq = n())
And finally apply required window functions:
result <- counts %>%
arrange(-freq) %>%
mutate(
rank = rank(),
total = sum(freq, na.rm = TRUE),
ratio = freq / total * 100)
Which will give you
result
# Source: spark<?> [?? x 6]
# Groups: variable
# Ordered by: -freq
variable level freq rank total ratio
<chr> <chr> <dbl> <int> <dbl> <dbl>
1 cut Ideal 21551 1 53940 40.0
2 cut Premium 13791 2 53940 25.6
3 cut Very Good 12082 3 53940 22.4
4 cut Good 4906 4 53940 9.10
5 cut Fair 1610 5 53940 2.98
6 clarity SI1 13065 1 53940 24.2
7 clarity VS2 12258 2 53940 22.7
8 clarity SI2 9194 3 53940 17.0
9 clarity VS1 8171 4 53940 15.1
10 clarity VVS2 5066 5 53940 9.39
# … with more rows
with following optimized plan
optimizedPlan(result)
<jobj[165]>
org.apache.spark.sql.catalyst.plans.logical.Project
Project [variable#524, level#525, freq#1478L, rank#1479, total#1480L, ((cast(freq#1478L as double) / cast(total#1480L as double)) * 100.0) AS ratio#1481]
+- Window [rank(_w1#1493L) windowspecdefinition(variable#524, _w1#1493L ASC NULLS FIRST, specifiedwindowframe(RowFrame, unboundedpreceding$(), currentrow$())) AS rank#1479], [variable#524], [_w1#1493L ASC NULLS FIRST]
+- Window [sum(freq#1478L) windowspecdefinition(variable#524, specifiedwindowframe(RowFrame, unboundedpreceding$(), unboundedfollowing$())) AS total#1480L], [variable#524]
+- Project [variable#524, level#525, freq#1478L, -freq#1478L AS _w1#1493L]
+- Sort [-freq#1478L ASC NULLS FIRST], true
+- Aggregate [variable#524, level#525], [variable#524, level#525, count(1) AS freq#1478L]
+- Generate explode(map(cut, cut#19, color, color#20, clarity, clarity#21)), [0, 1, 2], false, [variable#524, level#525]
+- Project [cut#19, color#20, clarity#21]
+- InMemoryRelation [carat#18, cut#19, color#20, clarity#21, depth#22, table#23, price#24, x#25, y#26, z#27], StorageLevel(disk, memory, deserialized, 1 replicas)
+- Scan ExistingRDD[carat#18,cut#19,color#20,clarity#21,depth#22,table#23,price#24,x#25,y#26,z#27]
and query (sdf_gather
component not included):
dbplyr::remote_query(result)
<SQL> SELECT `variable`, `level`, `freq`, `rank`, `total`, `freq` / `total` * 100.0 AS `ratio`
FROM (SELECT `variable`, `level`, `freq`, rank() OVER (PARTITION BY `variable` ORDER BY -`freq`) AS `rank`, sum(`freq`) OVER (PARTITION BY `variable`) AS `total`
FROM (SELECT *
FROM (SELECT `variable`, `level`, count(*) AS `freq`
FROM `sparklyr_tmp_ded2576b9f1`
GROUP BY `variable`, `level`) `dsbksdfhtf`
ORDER BY -`freq`) `obyrzsxeus`) `ekejqyjrfz`
add a comment |
Flatten your data using sdf_gather
:
long <- diamonds_tbl %>%
select(cut, color, clarity) %>%
sdf_gather("variable", "level", "cut", "color", "clarity")
Aggregate by variable
and level
:
counts <- long %>% group_by(variable, level) %>% summarise(freq = n())
And finally apply required window functions:
result <- counts %>%
arrange(-freq) %>%
mutate(
rank = rank(),
total = sum(freq, na.rm = TRUE),
ratio = freq / total * 100)
Which will give you
result
# Source: spark<?> [?? x 6]
# Groups: variable
# Ordered by: -freq
variable level freq rank total ratio
<chr> <chr> <dbl> <int> <dbl> <dbl>
1 cut Ideal 21551 1 53940 40.0
2 cut Premium 13791 2 53940 25.6
3 cut Very Good 12082 3 53940 22.4
4 cut Good 4906 4 53940 9.10
5 cut Fair 1610 5 53940 2.98
6 clarity SI1 13065 1 53940 24.2
7 clarity VS2 12258 2 53940 22.7
8 clarity SI2 9194 3 53940 17.0
9 clarity VS1 8171 4 53940 15.1
10 clarity VVS2 5066 5 53940 9.39
# … with more rows
with following optimized plan
optimizedPlan(result)
<jobj[165]>
org.apache.spark.sql.catalyst.plans.logical.Project
Project [variable#524, level#525, freq#1478L, rank#1479, total#1480L, ((cast(freq#1478L as double) / cast(total#1480L as double)) * 100.0) AS ratio#1481]
+- Window [rank(_w1#1493L) windowspecdefinition(variable#524, _w1#1493L ASC NULLS FIRST, specifiedwindowframe(RowFrame, unboundedpreceding$(), currentrow$())) AS rank#1479], [variable#524], [_w1#1493L ASC NULLS FIRST]
+- Window [sum(freq#1478L) windowspecdefinition(variable#524, specifiedwindowframe(RowFrame, unboundedpreceding$(), unboundedfollowing$())) AS total#1480L], [variable#524]
+- Project [variable#524, level#525, freq#1478L, -freq#1478L AS _w1#1493L]
+- Sort [-freq#1478L ASC NULLS FIRST], true
+- Aggregate [variable#524, level#525], [variable#524, level#525, count(1) AS freq#1478L]
+- Generate explode(map(cut, cut#19, color, color#20, clarity, clarity#21)), [0, 1, 2], false, [variable#524, level#525]
+- Project [cut#19, color#20, clarity#21]
+- InMemoryRelation [carat#18, cut#19, color#20, clarity#21, depth#22, table#23, price#24, x#25, y#26, z#27], StorageLevel(disk, memory, deserialized, 1 replicas)
+- Scan ExistingRDD[carat#18,cut#19,color#20,clarity#21,depth#22,table#23,price#24,x#25,y#26,z#27]
and query (sdf_gather
component not included):
dbplyr::remote_query(result)
<SQL> SELECT `variable`, `level`, `freq`, `rank`, `total`, `freq` / `total` * 100.0 AS `ratio`
FROM (SELECT `variable`, `level`, `freq`, rank() OVER (PARTITION BY `variable` ORDER BY -`freq`) AS `rank`, sum(`freq`) OVER (PARTITION BY `variable`) AS `total`
FROM (SELECT *
FROM (SELECT `variable`, `level`, count(*) AS `freq`
FROM `sparklyr_tmp_ded2576b9f1`
GROUP BY `variable`, `level`) `dsbksdfhtf`
ORDER BY -`freq`) `obyrzsxeus`) `ekejqyjrfz`
add a comment |
Flatten your data using sdf_gather
:
long <- diamonds_tbl %>%
select(cut, color, clarity) %>%
sdf_gather("variable", "level", "cut", "color", "clarity")
Aggregate by variable
and level
:
counts <- long %>% group_by(variable, level) %>% summarise(freq = n())
And finally apply required window functions:
result <- counts %>%
arrange(-freq) %>%
mutate(
rank = rank(),
total = sum(freq, na.rm = TRUE),
ratio = freq / total * 100)
Which will give you
result
# Source: spark<?> [?? x 6]
# Groups: variable
# Ordered by: -freq
variable level freq rank total ratio
<chr> <chr> <dbl> <int> <dbl> <dbl>
1 cut Ideal 21551 1 53940 40.0
2 cut Premium 13791 2 53940 25.6
3 cut Very Good 12082 3 53940 22.4
4 cut Good 4906 4 53940 9.10
5 cut Fair 1610 5 53940 2.98
6 clarity SI1 13065 1 53940 24.2
7 clarity VS2 12258 2 53940 22.7
8 clarity SI2 9194 3 53940 17.0
9 clarity VS1 8171 4 53940 15.1
10 clarity VVS2 5066 5 53940 9.39
# … with more rows
with following optimized plan
optimizedPlan(result)
<jobj[165]>
org.apache.spark.sql.catalyst.plans.logical.Project
Project [variable#524, level#525, freq#1478L, rank#1479, total#1480L, ((cast(freq#1478L as double) / cast(total#1480L as double)) * 100.0) AS ratio#1481]
+- Window [rank(_w1#1493L) windowspecdefinition(variable#524, _w1#1493L ASC NULLS FIRST, specifiedwindowframe(RowFrame, unboundedpreceding$(), currentrow$())) AS rank#1479], [variable#524], [_w1#1493L ASC NULLS FIRST]
+- Window [sum(freq#1478L) windowspecdefinition(variable#524, specifiedwindowframe(RowFrame, unboundedpreceding$(), unboundedfollowing$())) AS total#1480L], [variable#524]
+- Project [variable#524, level#525, freq#1478L, -freq#1478L AS _w1#1493L]
+- Sort [-freq#1478L ASC NULLS FIRST], true
+- Aggregate [variable#524, level#525], [variable#524, level#525, count(1) AS freq#1478L]
+- Generate explode(map(cut, cut#19, color, color#20, clarity, clarity#21)), [0, 1, 2], false, [variable#524, level#525]
+- Project [cut#19, color#20, clarity#21]
+- InMemoryRelation [carat#18, cut#19, color#20, clarity#21, depth#22, table#23, price#24, x#25, y#26, z#27], StorageLevel(disk, memory, deserialized, 1 replicas)
+- Scan ExistingRDD[carat#18,cut#19,color#20,clarity#21,depth#22,table#23,price#24,x#25,y#26,z#27]
and query (sdf_gather
component not included):
dbplyr::remote_query(result)
<SQL> SELECT `variable`, `level`, `freq`, `rank`, `total`, `freq` / `total` * 100.0 AS `ratio`
FROM (SELECT `variable`, `level`, `freq`, rank() OVER (PARTITION BY `variable` ORDER BY -`freq`) AS `rank`, sum(`freq`) OVER (PARTITION BY `variable`) AS `total`
FROM (SELECT *
FROM (SELECT `variable`, `level`, count(*) AS `freq`
FROM `sparklyr_tmp_ded2576b9f1`
GROUP BY `variable`, `level`) `dsbksdfhtf`
ORDER BY -`freq`) `obyrzsxeus`) `ekejqyjrfz`
Flatten your data using sdf_gather
:
long <- diamonds_tbl %>%
select(cut, color, clarity) %>%
sdf_gather("variable", "level", "cut", "color", "clarity")
Aggregate by variable
and level
:
counts <- long %>% group_by(variable, level) %>% summarise(freq = n())
And finally apply required window functions:
result <- counts %>%
arrange(-freq) %>%
mutate(
rank = rank(),
total = sum(freq, na.rm = TRUE),
ratio = freq / total * 100)
Which will give you
result
# Source: spark<?> [?? x 6]
# Groups: variable
# Ordered by: -freq
variable level freq rank total ratio
<chr> <chr> <dbl> <int> <dbl> <dbl>
1 cut Ideal 21551 1 53940 40.0
2 cut Premium 13791 2 53940 25.6
3 cut Very Good 12082 3 53940 22.4
4 cut Good 4906 4 53940 9.10
5 cut Fair 1610 5 53940 2.98
6 clarity SI1 13065 1 53940 24.2
7 clarity VS2 12258 2 53940 22.7
8 clarity SI2 9194 3 53940 17.0
9 clarity VS1 8171 4 53940 15.1
10 clarity VVS2 5066 5 53940 9.39
# … with more rows
with following optimized plan
optimizedPlan(result)
<jobj[165]>
org.apache.spark.sql.catalyst.plans.logical.Project
Project [variable#524, level#525, freq#1478L, rank#1479, total#1480L, ((cast(freq#1478L as double) / cast(total#1480L as double)) * 100.0) AS ratio#1481]
+- Window [rank(_w1#1493L) windowspecdefinition(variable#524, _w1#1493L ASC NULLS FIRST, specifiedwindowframe(RowFrame, unboundedpreceding$(), currentrow$())) AS rank#1479], [variable#524], [_w1#1493L ASC NULLS FIRST]
+- Window [sum(freq#1478L) windowspecdefinition(variable#524, specifiedwindowframe(RowFrame, unboundedpreceding$(), unboundedfollowing$())) AS total#1480L], [variable#524]
+- Project [variable#524, level#525, freq#1478L, -freq#1478L AS _w1#1493L]
+- Sort [-freq#1478L ASC NULLS FIRST], true
+- Aggregate [variable#524, level#525], [variable#524, level#525, count(1) AS freq#1478L]
+- Generate explode(map(cut, cut#19, color, color#20, clarity, clarity#21)), [0, 1, 2], false, [variable#524, level#525]
+- Project [cut#19, color#20, clarity#21]
+- InMemoryRelation [carat#18, cut#19, color#20, clarity#21, depth#22, table#23, price#24, x#25, y#26, z#27], StorageLevel(disk, memory, deserialized, 1 replicas)
+- Scan ExistingRDD[carat#18,cut#19,color#20,clarity#21,depth#22,table#23,price#24,x#25,y#26,z#27]
and query (sdf_gather
component not included):
dbplyr::remote_query(result)
<SQL> SELECT `variable`, `level`, `freq`, `rank`, `total`, `freq` / `total` * 100.0 AS `ratio`
FROM (SELECT `variable`, `level`, `freq`, rank() OVER (PARTITION BY `variable` ORDER BY -`freq`) AS `rank`, sum(`freq`) OVER (PARTITION BY `variable`) AS `total`
FROM (SELECT *
FROM (SELECT `variable`, `level`, count(*) AS `freq`
FROM `sparklyr_tmp_ded2576b9f1`
GROUP BY `variable`, `level`) `dsbksdfhtf`
ORDER BY -`freq`) `obyrzsxeus`) `ekejqyjrfz`
edited Jan 26 at 11:08
answered Jan 26 at 10:59
user6910411user6910411
35.3k1089108
35.3k1089108
add a comment |
add a comment |
Thanks for contributing an answer to Stack Overflow!
- Please be sure to answer the question. Provide details and share your research!
But avoid …
- Asking for help, clarification, or responding to other answers.
- Making statements based on opinion; back them up with references or personal experience.
To learn more, see our tips on writing great answers.
Sign up or log in
StackExchange.ready(function ()
StackExchange.helpers.onClickDraftSave('#login-link');
);
Sign up using Google
Sign up using Facebook
Sign up using Email and Password
Post as a guest
Required, but never shown
StackExchange.ready(
function ()
StackExchange.openid.initPostLogin('.new-post-login', 'https%3a%2f%2fstackoverflow.com%2fquestions%2f54373886%2fhow-to-use-sparklyr-to-summarize-categorical-variable-level%23new-answer', 'question_page');
);
Post as a guest
Required, but never shown
Sign up or log in
StackExchange.ready(function ()
StackExchange.helpers.onClickDraftSave('#login-link');
);
Sign up using Google
Sign up using Facebook
Sign up using Email and Password
Post as a guest
Required, but never shown
Sign up or log in
StackExchange.ready(function ()
StackExchange.helpers.onClickDraftSave('#login-link');
);
Sign up using Google
Sign up using Facebook
Sign up using Email and Password
Post as a guest
Required, but never shown
Sign up or log in
StackExchange.ready(function ()
StackExchange.helpers.onClickDraftSave('#login-link');
);
Sign up using Google
Sign up using Facebook
Sign up using Email and Password
Sign up using Google
Sign up using Facebook
Sign up using Email and Password
Post as a guest
Required, but never shown
Required, but never shown
Required, but never shown
Required, but never shown
Required, but never shown
Required, but never shown
Required, but never shown
Required, but never shown
Required, but never shown
jJ otghIYZ,qhix9c72IAU13brgPoF25P7vQ3 CNWYCojt8dq