Nested apply instead of double for-loops in R for multiple linear regression The Next CEO of Stack OverflowConstrained linear regression coefficients in RCalculating correlation between residuals of linear regression with NAs and independent variable in RUse a function with a linear regression modelmultiple linear regression with apply or loop? in Rpredict lm function in R (multiple linear regression)Nested apply functionHow to conduct linear hypothesis test on regression coefficients with a clustered covariance matrix?loop or apply multiple regressions, extract coefficients and p-values into data frameFast pairwise simple linear regression between variables in a data frameInterpretation of standard errors in lm() model of linear regression in R
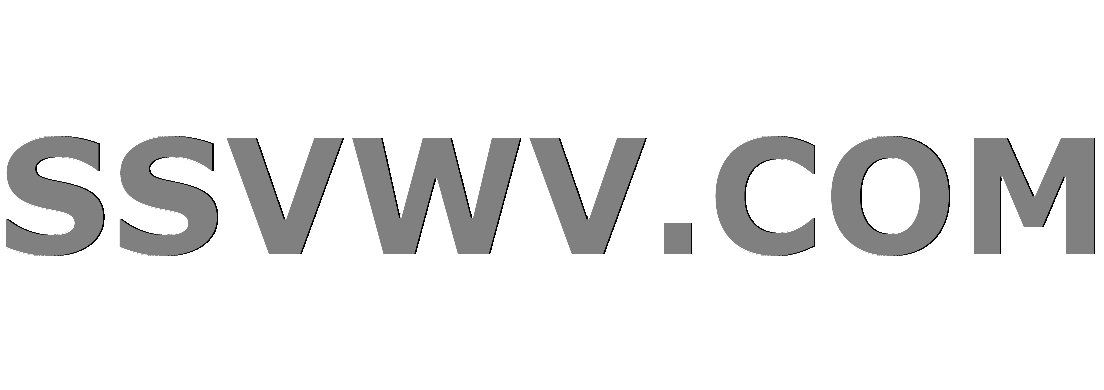
Multi tool use
logical reads on global temp table, but not on session-level temp table
What is the difference between 서고 and 도서관?
How can a day be of 24 hours?
Can I cast Thunderwave and be at the center of its bottom face, but not be affected by it?
Is a linearly independent set whose span is dense a Schauder basis?
My boss doesn't want me to have a side project
What is a typical Mizrachi Seder like?
Can Sri Krishna be called 'a person'?
What did the word "leisure" mean in late 18th Century usage?
Is it possible to make a 9x9 table fit within the default margins?
Could you use a laser beam as a modulated carrier wave for radio signal?
Avoiding the "not like other girls" trope?
Is it OK to decorate a log book cover?
Upgrading From a 9 Speed Sora Derailleur?
How to compactly explain secondary and tertiary characters without resorting to stereotypes?
Identify and count spells (Distinctive events within each group)
How should I connect my cat5 cable to connectors having an orange-green line?
Why was Sir Cadogan fired?
Ising model simulation
pgfplots: How to draw a tangent graph below two others?
Why does sin(x) - sin(y) equal this?
Are British MPs missing the point, with these 'Indicative Votes'?
How to pronounce fünf in 45
Can you teleport closer to a creature you are Frightened of?
Nested apply instead of double for-loops in R for multiple linear regression
The Next CEO of Stack OverflowConstrained linear regression coefficients in RCalculating correlation between residuals of linear regression with NAs and independent variable in RUse a function with a linear regression modelmultiple linear regression with apply or loop? in Rpredict lm function in R (multiple linear regression)Nested apply functionHow to conduct linear hypothesis test on regression coefficients with a clustered covariance matrix?loop or apply multiple regressions, extract coefficients and p-values into data frameFast pairwise simple linear regression between variables in a data frameInterpretation of standard errors in lm() model of linear regression in R
I have two data matrices, a and b (with multiple cols) and 2 covariate matrices (1 col each). I want to apply a multiple linear regression and get the coefficients for the regression between each column of a with the factors of b, repsectively.
Covariates are c1 and c2.
I want the output to look like this:
Estimate Std. Error t value Pr(>|t|)
a1 b1
a1 b2
...
a2 b1
a2 b2
...
a3 b1
a3 b2
...
The basic formula for linear regression is lm(y~x+c1+c2)
I tried this nested apply
apply(a, 2, function(x) apply(b, 2, function(y) summary(lm(y~x+c1+c2))$coefficients)[2,])
but it only gives me the p-values in the following format:
a1 a2 a3
b1
b2
I also tried this:
for (i in dim(a)[2])
pvals= apply(b, 2, function(y) summary(lm(y~a[i]+c1+c2))$coefficients)[2,]
This gives an error "variable lengths differ (found for 'a[i]')"
Any help with this would be much appreciated.
r for-loop nested apply lm
add a comment |
I have two data matrices, a and b (with multiple cols) and 2 covariate matrices (1 col each). I want to apply a multiple linear regression and get the coefficients for the regression between each column of a with the factors of b, repsectively.
Covariates are c1 and c2.
I want the output to look like this:
Estimate Std. Error t value Pr(>|t|)
a1 b1
a1 b2
...
a2 b1
a2 b2
...
a3 b1
a3 b2
...
The basic formula for linear regression is lm(y~x+c1+c2)
I tried this nested apply
apply(a, 2, function(x) apply(b, 2, function(y) summary(lm(y~x+c1+c2))$coefficients)[2,])
but it only gives me the p-values in the following format:
a1 a2 a3
b1
b2
I also tried this:
for (i in dim(a)[2])
pvals= apply(b, 2, function(y) summary(lm(y~a[i]+c1+c2))$coefficients)[2,]
This gives an error "variable lengths differ (found for 'a[i]')"
Any help with this would be much appreciated.
r for-loop nested apply lm
add a comment |
I have two data matrices, a and b (with multiple cols) and 2 covariate matrices (1 col each). I want to apply a multiple linear regression and get the coefficients for the regression between each column of a with the factors of b, repsectively.
Covariates are c1 and c2.
I want the output to look like this:
Estimate Std. Error t value Pr(>|t|)
a1 b1
a1 b2
...
a2 b1
a2 b2
...
a3 b1
a3 b2
...
The basic formula for linear regression is lm(y~x+c1+c2)
I tried this nested apply
apply(a, 2, function(x) apply(b, 2, function(y) summary(lm(y~x+c1+c2))$coefficients)[2,])
but it only gives me the p-values in the following format:
a1 a2 a3
b1
b2
I also tried this:
for (i in dim(a)[2])
pvals= apply(b, 2, function(y) summary(lm(y~a[i]+c1+c2))$coefficients)[2,]
This gives an error "variable lengths differ (found for 'a[i]')"
Any help with this would be much appreciated.
r for-loop nested apply lm
I have two data matrices, a and b (with multiple cols) and 2 covariate matrices (1 col each). I want to apply a multiple linear regression and get the coefficients for the regression between each column of a with the factors of b, repsectively.
Covariates are c1 and c2.
I want the output to look like this:
Estimate Std. Error t value Pr(>|t|)
a1 b1
a1 b2
...
a2 b1
a2 b2
...
a3 b1
a3 b2
...
The basic formula for linear regression is lm(y~x+c1+c2)
I tried this nested apply
apply(a, 2, function(x) apply(b, 2, function(y) summary(lm(y~x+c1+c2))$coefficients)[2,])
but it only gives me the p-values in the following format:
a1 a2 a3
b1
b2
I also tried this:
for (i in dim(a)[2])
pvals= apply(b, 2, function(y) summary(lm(y~a[i]+c1+c2))$coefficients)[2,]
This gives an error "variable lengths differ (found for 'a[i]')"
Any help with this would be much appreciated.
r for-loop nested apply lm
r for-loop nested apply lm
edited Mar 9 at 0:35
ha_pu
27219
27219
asked Mar 8 at 19:34
geneNaziageneNazia
62
62
add a comment |
add a comment |
2 Answers
2
active
oldest
votes
Try this :
# transform your data matrices into data.frames
a <- as.data.frame( matrix(rnorm(1:(250*4)), ncol = 4) )
colnames(a) <- paste0("A", 1:ncol(a))
b <- as.data.frame( matrix(rnorm(1:(250*6)), ncol = 6) )
colnames(b) <- paste0("B", 1:ncol(b))
c1 <- rnorm(1:250)
c2 <- rnorm(1:250)
# get the explanatory variables, RHS of the formula
X <- paste(c(colnames(b), "c1", "c2"), collapse = "+")
# get the dependent variables, LHS of the formula
Y <- colnames(a)
# Create a single data.frame
dat <- data.frame(a, b, c1, c2)
# Do the regressions
results <- lapply(Y, function(y)
coefficients( lm(
as.formula( paste0(y, " ~ ", X) ), data=dat)) )
```
add a comment |
I suppose the trick is to write the column of the data matrix as variable during your apply / map command.
library(broom) # to clean the regression output
library(tidyverse)
a <- matrix(rnorm(1:1000), ncol = 4)
head(a)
[,1] [,2] [,3] [,4]
[1,] 0.9214791 0.3273086 -0.456702485 1.504571891
[2,] -0.6705181 1.3443408 1.496302280 0.516068092
[3,] -0.9122278 0.2392211 -0.163004516 -0.041937414
[4,] -0.6614763 1.1596926 2.004846224 -0.001818212
[5,] -0.7902421 0.3022333 -0.002848944 0.265987941
[6,] 0.3451988 0.3187038 -0.149836811 0.122283166
b <- matrix(rnorm(1:500), ncol = 2)
head(b)
[,1] [,2]
[1,] 1.6100023 0.4861797
[2,] 0.2128886 -1.0762123
[3,] -0.7645170 -0.4972273
[4,] -0.4084541 0.8930468
[5,] -0.1471686 -1.3193856
[6,] 0.4331506 -0.4044583
c <- matrix(rnorm(1:500), ncol = 2)
head(c)
[,1] [,2]
[1,] -0.9476932 0.1292495
[2,] -0.8653959 -1.3278809
[3,] -1.5162128 0.2765994
[4,] -0.5140617 1.8684472
[5,] 0.8104582 1.7564293
[6,] 1.4162302 -1.5383332
(col_a <- seq(dim(a)[2])) # to map to the columns of matrix a
[1] 1 2 3 4
(col_b <- seq(dim(b)[2])) # to map to the columns of matrix b
[1] 1 2
map_df(col_a, ~ map2_df(.x, col_b, ~ lm(b[,.y] ~ a[,.x] + c) %>% # the first ".x" uses the mapping output from the first "map_df" in the second "map2_df"
tidy() %>% # clean regression output
mutate(y = str_c("b", .y, sep = "_"), # add variable y with indicator for matrix b
x = str_c("a", .x, sep = "_")))) %>% # add variable x with indicator for matrix a
select(y, x, 1:5) # rearrange columns
# A tibble: 32 x 7
y x term estimate std.error statistic p.value
<chr> <chr> <chr> <dbl> <dbl> <dbl> <dbl>
1 b_1 a_1 (Intercept) -0.0747 0.0645 -1.16 0.248
2 b_1 a_1 a[, .x] 0.0653 0.0638 1.02 0.307
3 b_1 a_1 c1 -0.117 0.0672 -1.74 0.0834
4 b_1 a_1 c2 0.0219 0.0617 0.355 0.723
5 b_2 a_1 (Intercept) 0.0145 0.0618 0.234 0.815
6 b_2 a_1 a[, .x] -0.142 0.0612 -2.33 0.0208
7 b_2 a_1 c1 0.0458 0.0644 0.711 0.478
8 b_2 a_1 c2 0.0450 0.0591 0.761 0.447
9 b_1 a_2 (Intercept) -0.0779 0.0645 -1.21 0.229
10 b_1 a_2 a[, .x] -0.0502 0.0678 -0.741 0.459
# ... with 22 more rows
add a comment |
StackExchange.ifUsing("editor", function ()
StackExchange.using("externalEditor", function ()
StackExchange.using("snippets", function ()
StackExchange.snippets.init();
);
);
, "code-snippets");
StackExchange.ready(function()
var channelOptions =
tags: "".split(" "),
id: "1"
;
initTagRenderer("".split(" "), "".split(" "), channelOptions);
StackExchange.using("externalEditor", function()
// Have to fire editor after snippets, if snippets enabled
if (StackExchange.settings.snippets.snippetsEnabled)
StackExchange.using("snippets", function()
createEditor();
);
else
createEditor();
);
function createEditor()
StackExchange.prepareEditor(
heartbeatType: 'answer',
autoActivateHeartbeat: false,
convertImagesToLinks: true,
noModals: true,
showLowRepImageUploadWarning: true,
reputationToPostImages: 10,
bindNavPrevention: true,
postfix: "",
imageUploader:
brandingHtml: "Powered by u003ca class="icon-imgur-white" href="https://imgur.com/"u003eu003c/au003e",
contentPolicyHtml: "User contributions licensed under u003ca href="https://creativecommons.org/licenses/by-sa/3.0/"u003ecc by-sa 3.0 with attribution requiredu003c/au003e u003ca href="https://stackoverflow.com/legal/content-policy"u003e(content policy)u003c/au003e",
allowUrls: true
,
onDemand: true,
discardSelector: ".discard-answer"
,immediatelyShowMarkdownHelp:true
);
);
Sign up or log in
StackExchange.ready(function ()
StackExchange.helpers.onClickDraftSave('#login-link');
);
Sign up using Google
Sign up using Facebook
Sign up using Email and Password
Post as a guest
Required, but never shown
StackExchange.ready(
function ()
StackExchange.openid.initPostLogin('.new-post-login', 'https%3a%2f%2fstackoverflow.com%2fquestions%2f55069864%2fnested-apply-instead-of-double-for-loops-in-r-for-multiple-linear-regression%23new-answer', 'question_page');
);
Post as a guest
Required, but never shown
2 Answers
2
active
oldest
votes
2 Answers
2
active
oldest
votes
active
oldest
votes
active
oldest
votes
Try this :
# transform your data matrices into data.frames
a <- as.data.frame( matrix(rnorm(1:(250*4)), ncol = 4) )
colnames(a) <- paste0("A", 1:ncol(a))
b <- as.data.frame( matrix(rnorm(1:(250*6)), ncol = 6) )
colnames(b) <- paste0("B", 1:ncol(b))
c1 <- rnorm(1:250)
c2 <- rnorm(1:250)
# get the explanatory variables, RHS of the formula
X <- paste(c(colnames(b), "c1", "c2"), collapse = "+")
# get the dependent variables, LHS of the formula
Y <- colnames(a)
# Create a single data.frame
dat <- data.frame(a, b, c1, c2)
# Do the regressions
results <- lapply(Y, function(y)
coefficients( lm(
as.formula( paste0(y, " ~ ", X) ), data=dat)) )
```
add a comment |
Try this :
# transform your data matrices into data.frames
a <- as.data.frame( matrix(rnorm(1:(250*4)), ncol = 4) )
colnames(a) <- paste0("A", 1:ncol(a))
b <- as.data.frame( matrix(rnorm(1:(250*6)), ncol = 6) )
colnames(b) <- paste0("B", 1:ncol(b))
c1 <- rnorm(1:250)
c2 <- rnorm(1:250)
# get the explanatory variables, RHS of the formula
X <- paste(c(colnames(b), "c1", "c2"), collapse = "+")
# get the dependent variables, LHS of the formula
Y <- colnames(a)
# Create a single data.frame
dat <- data.frame(a, b, c1, c2)
# Do the regressions
results <- lapply(Y, function(y)
coefficients( lm(
as.formula( paste0(y, " ~ ", X) ), data=dat)) )
```
add a comment |
Try this :
# transform your data matrices into data.frames
a <- as.data.frame( matrix(rnorm(1:(250*4)), ncol = 4) )
colnames(a) <- paste0("A", 1:ncol(a))
b <- as.data.frame( matrix(rnorm(1:(250*6)), ncol = 6) )
colnames(b) <- paste0("B", 1:ncol(b))
c1 <- rnorm(1:250)
c2 <- rnorm(1:250)
# get the explanatory variables, RHS of the formula
X <- paste(c(colnames(b), "c1", "c2"), collapse = "+")
# get the dependent variables, LHS of the formula
Y <- colnames(a)
# Create a single data.frame
dat <- data.frame(a, b, c1, c2)
# Do the regressions
results <- lapply(Y, function(y)
coefficients( lm(
as.formula( paste0(y, " ~ ", X) ), data=dat)) )
```
Try this :
# transform your data matrices into data.frames
a <- as.data.frame( matrix(rnorm(1:(250*4)), ncol = 4) )
colnames(a) <- paste0("A", 1:ncol(a))
b <- as.data.frame( matrix(rnorm(1:(250*6)), ncol = 6) )
colnames(b) <- paste0("B", 1:ncol(b))
c1 <- rnorm(1:250)
c2 <- rnorm(1:250)
# get the explanatory variables, RHS of the formula
X <- paste(c(colnames(b), "c1", "c2"), collapse = "+")
# get the dependent variables, LHS of the formula
Y <- colnames(a)
# Create a single data.frame
dat <- data.frame(a, b, c1, c2)
# Do the regressions
results <- lapply(Y, function(y)
coefficients( lm(
as.formula( paste0(y, " ~ ", X) ), data=dat)) )
```
edited Mar 8 at 20:14
answered Mar 8 at 20:06


Ismail MüllerIsmail Müller
1164
1164
add a comment |
add a comment |
I suppose the trick is to write the column of the data matrix as variable during your apply / map command.
library(broom) # to clean the regression output
library(tidyverse)
a <- matrix(rnorm(1:1000), ncol = 4)
head(a)
[,1] [,2] [,3] [,4]
[1,] 0.9214791 0.3273086 -0.456702485 1.504571891
[2,] -0.6705181 1.3443408 1.496302280 0.516068092
[3,] -0.9122278 0.2392211 -0.163004516 -0.041937414
[4,] -0.6614763 1.1596926 2.004846224 -0.001818212
[5,] -0.7902421 0.3022333 -0.002848944 0.265987941
[6,] 0.3451988 0.3187038 -0.149836811 0.122283166
b <- matrix(rnorm(1:500), ncol = 2)
head(b)
[,1] [,2]
[1,] 1.6100023 0.4861797
[2,] 0.2128886 -1.0762123
[3,] -0.7645170 -0.4972273
[4,] -0.4084541 0.8930468
[5,] -0.1471686 -1.3193856
[6,] 0.4331506 -0.4044583
c <- matrix(rnorm(1:500), ncol = 2)
head(c)
[,1] [,2]
[1,] -0.9476932 0.1292495
[2,] -0.8653959 -1.3278809
[3,] -1.5162128 0.2765994
[4,] -0.5140617 1.8684472
[5,] 0.8104582 1.7564293
[6,] 1.4162302 -1.5383332
(col_a <- seq(dim(a)[2])) # to map to the columns of matrix a
[1] 1 2 3 4
(col_b <- seq(dim(b)[2])) # to map to the columns of matrix b
[1] 1 2
map_df(col_a, ~ map2_df(.x, col_b, ~ lm(b[,.y] ~ a[,.x] + c) %>% # the first ".x" uses the mapping output from the first "map_df" in the second "map2_df"
tidy() %>% # clean regression output
mutate(y = str_c("b", .y, sep = "_"), # add variable y with indicator for matrix b
x = str_c("a", .x, sep = "_")))) %>% # add variable x with indicator for matrix a
select(y, x, 1:5) # rearrange columns
# A tibble: 32 x 7
y x term estimate std.error statistic p.value
<chr> <chr> <chr> <dbl> <dbl> <dbl> <dbl>
1 b_1 a_1 (Intercept) -0.0747 0.0645 -1.16 0.248
2 b_1 a_1 a[, .x] 0.0653 0.0638 1.02 0.307
3 b_1 a_1 c1 -0.117 0.0672 -1.74 0.0834
4 b_1 a_1 c2 0.0219 0.0617 0.355 0.723
5 b_2 a_1 (Intercept) 0.0145 0.0618 0.234 0.815
6 b_2 a_1 a[, .x] -0.142 0.0612 -2.33 0.0208
7 b_2 a_1 c1 0.0458 0.0644 0.711 0.478
8 b_2 a_1 c2 0.0450 0.0591 0.761 0.447
9 b_1 a_2 (Intercept) -0.0779 0.0645 -1.21 0.229
10 b_1 a_2 a[, .x] -0.0502 0.0678 -0.741 0.459
# ... with 22 more rows
add a comment |
I suppose the trick is to write the column of the data matrix as variable during your apply / map command.
library(broom) # to clean the regression output
library(tidyverse)
a <- matrix(rnorm(1:1000), ncol = 4)
head(a)
[,1] [,2] [,3] [,4]
[1,] 0.9214791 0.3273086 -0.456702485 1.504571891
[2,] -0.6705181 1.3443408 1.496302280 0.516068092
[3,] -0.9122278 0.2392211 -0.163004516 -0.041937414
[4,] -0.6614763 1.1596926 2.004846224 -0.001818212
[5,] -0.7902421 0.3022333 -0.002848944 0.265987941
[6,] 0.3451988 0.3187038 -0.149836811 0.122283166
b <- matrix(rnorm(1:500), ncol = 2)
head(b)
[,1] [,2]
[1,] 1.6100023 0.4861797
[2,] 0.2128886 -1.0762123
[3,] -0.7645170 -0.4972273
[4,] -0.4084541 0.8930468
[5,] -0.1471686 -1.3193856
[6,] 0.4331506 -0.4044583
c <- matrix(rnorm(1:500), ncol = 2)
head(c)
[,1] [,2]
[1,] -0.9476932 0.1292495
[2,] -0.8653959 -1.3278809
[3,] -1.5162128 0.2765994
[4,] -0.5140617 1.8684472
[5,] 0.8104582 1.7564293
[6,] 1.4162302 -1.5383332
(col_a <- seq(dim(a)[2])) # to map to the columns of matrix a
[1] 1 2 3 4
(col_b <- seq(dim(b)[2])) # to map to the columns of matrix b
[1] 1 2
map_df(col_a, ~ map2_df(.x, col_b, ~ lm(b[,.y] ~ a[,.x] + c) %>% # the first ".x" uses the mapping output from the first "map_df" in the second "map2_df"
tidy() %>% # clean regression output
mutate(y = str_c("b", .y, sep = "_"), # add variable y with indicator for matrix b
x = str_c("a", .x, sep = "_")))) %>% # add variable x with indicator for matrix a
select(y, x, 1:5) # rearrange columns
# A tibble: 32 x 7
y x term estimate std.error statistic p.value
<chr> <chr> <chr> <dbl> <dbl> <dbl> <dbl>
1 b_1 a_1 (Intercept) -0.0747 0.0645 -1.16 0.248
2 b_1 a_1 a[, .x] 0.0653 0.0638 1.02 0.307
3 b_1 a_1 c1 -0.117 0.0672 -1.74 0.0834
4 b_1 a_1 c2 0.0219 0.0617 0.355 0.723
5 b_2 a_1 (Intercept) 0.0145 0.0618 0.234 0.815
6 b_2 a_1 a[, .x] -0.142 0.0612 -2.33 0.0208
7 b_2 a_1 c1 0.0458 0.0644 0.711 0.478
8 b_2 a_1 c2 0.0450 0.0591 0.761 0.447
9 b_1 a_2 (Intercept) -0.0779 0.0645 -1.21 0.229
10 b_1 a_2 a[, .x] -0.0502 0.0678 -0.741 0.459
# ... with 22 more rows
add a comment |
I suppose the trick is to write the column of the data matrix as variable during your apply / map command.
library(broom) # to clean the regression output
library(tidyverse)
a <- matrix(rnorm(1:1000), ncol = 4)
head(a)
[,1] [,2] [,3] [,4]
[1,] 0.9214791 0.3273086 -0.456702485 1.504571891
[2,] -0.6705181 1.3443408 1.496302280 0.516068092
[3,] -0.9122278 0.2392211 -0.163004516 -0.041937414
[4,] -0.6614763 1.1596926 2.004846224 -0.001818212
[5,] -0.7902421 0.3022333 -0.002848944 0.265987941
[6,] 0.3451988 0.3187038 -0.149836811 0.122283166
b <- matrix(rnorm(1:500), ncol = 2)
head(b)
[,1] [,2]
[1,] 1.6100023 0.4861797
[2,] 0.2128886 -1.0762123
[3,] -0.7645170 -0.4972273
[4,] -0.4084541 0.8930468
[5,] -0.1471686 -1.3193856
[6,] 0.4331506 -0.4044583
c <- matrix(rnorm(1:500), ncol = 2)
head(c)
[,1] [,2]
[1,] -0.9476932 0.1292495
[2,] -0.8653959 -1.3278809
[3,] -1.5162128 0.2765994
[4,] -0.5140617 1.8684472
[5,] 0.8104582 1.7564293
[6,] 1.4162302 -1.5383332
(col_a <- seq(dim(a)[2])) # to map to the columns of matrix a
[1] 1 2 3 4
(col_b <- seq(dim(b)[2])) # to map to the columns of matrix b
[1] 1 2
map_df(col_a, ~ map2_df(.x, col_b, ~ lm(b[,.y] ~ a[,.x] + c) %>% # the first ".x" uses the mapping output from the first "map_df" in the second "map2_df"
tidy() %>% # clean regression output
mutate(y = str_c("b", .y, sep = "_"), # add variable y with indicator for matrix b
x = str_c("a", .x, sep = "_")))) %>% # add variable x with indicator for matrix a
select(y, x, 1:5) # rearrange columns
# A tibble: 32 x 7
y x term estimate std.error statistic p.value
<chr> <chr> <chr> <dbl> <dbl> <dbl> <dbl>
1 b_1 a_1 (Intercept) -0.0747 0.0645 -1.16 0.248
2 b_1 a_1 a[, .x] 0.0653 0.0638 1.02 0.307
3 b_1 a_1 c1 -0.117 0.0672 -1.74 0.0834
4 b_1 a_1 c2 0.0219 0.0617 0.355 0.723
5 b_2 a_1 (Intercept) 0.0145 0.0618 0.234 0.815
6 b_2 a_1 a[, .x] -0.142 0.0612 -2.33 0.0208
7 b_2 a_1 c1 0.0458 0.0644 0.711 0.478
8 b_2 a_1 c2 0.0450 0.0591 0.761 0.447
9 b_1 a_2 (Intercept) -0.0779 0.0645 -1.21 0.229
10 b_1 a_2 a[, .x] -0.0502 0.0678 -0.741 0.459
# ... with 22 more rows
I suppose the trick is to write the column of the data matrix as variable during your apply / map command.
library(broom) # to clean the regression output
library(tidyverse)
a <- matrix(rnorm(1:1000), ncol = 4)
head(a)
[,1] [,2] [,3] [,4]
[1,] 0.9214791 0.3273086 -0.456702485 1.504571891
[2,] -0.6705181 1.3443408 1.496302280 0.516068092
[3,] -0.9122278 0.2392211 -0.163004516 -0.041937414
[4,] -0.6614763 1.1596926 2.004846224 -0.001818212
[5,] -0.7902421 0.3022333 -0.002848944 0.265987941
[6,] 0.3451988 0.3187038 -0.149836811 0.122283166
b <- matrix(rnorm(1:500), ncol = 2)
head(b)
[,1] [,2]
[1,] 1.6100023 0.4861797
[2,] 0.2128886 -1.0762123
[3,] -0.7645170 -0.4972273
[4,] -0.4084541 0.8930468
[5,] -0.1471686 -1.3193856
[6,] 0.4331506 -0.4044583
c <- matrix(rnorm(1:500), ncol = 2)
head(c)
[,1] [,2]
[1,] -0.9476932 0.1292495
[2,] -0.8653959 -1.3278809
[3,] -1.5162128 0.2765994
[4,] -0.5140617 1.8684472
[5,] 0.8104582 1.7564293
[6,] 1.4162302 -1.5383332
(col_a <- seq(dim(a)[2])) # to map to the columns of matrix a
[1] 1 2 3 4
(col_b <- seq(dim(b)[2])) # to map to the columns of matrix b
[1] 1 2
map_df(col_a, ~ map2_df(.x, col_b, ~ lm(b[,.y] ~ a[,.x] + c) %>% # the first ".x" uses the mapping output from the first "map_df" in the second "map2_df"
tidy() %>% # clean regression output
mutate(y = str_c("b", .y, sep = "_"), # add variable y with indicator for matrix b
x = str_c("a", .x, sep = "_")))) %>% # add variable x with indicator for matrix a
select(y, x, 1:5) # rearrange columns
# A tibble: 32 x 7
y x term estimate std.error statistic p.value
<chr> <chr> <chr> <dbl> <dbl> <dbl> <dbl>
1 b_1 a_1 (Intercept) -0.0747 0.0645 -1.16 0.248
2 b_1 a_1 a[, .x] 0.0653 0.0638 1.02 0.307
3 b_1 a_1 c1 -0.117 0.0672 -1.74 0.0834
4 b_1 a_1 c2 0.0219 0.0617 0.355 0.723
5 b_2 a_1 (Intercept) 0.0145 0.0618 0.234 0.815
6 b_2 a_1 a[, .x] -0.142 0.0612 -2.33 0.0208
7 b_2 a_1 c1 0.0458 0.0644 0.711 0.478
8 b_2 a_1 c2 0.0450 0.0591 0.761 0.447
9 b_1 a_2 (Intercept) -0.0779 0.0645 -1.21 0.229
10 b_1 a_2 a[, .x] -0.0502 0.0678 -0.741 0.459
# ... with 22 more rows
answered Mar 8 at 19:58
ha_puha_pu
27219
27219
add a comment |
add a comment |
Thanks for contributing an answer to Stack Overflow!
- Please be sure to answer the question. Provide details and share your research!
But avoid …
- Asking for help, clarification, or responding to other answers.
- Making statements based on opinion; back them up with references or personal experience.
To learn more, see our tips on writing great answers.
Sign up or log in
StackExchange.ready(function ()
StackExchange.helpers.onClickDraftSave('#login-link');
);
Sign up using Google
Sign up using Facebook
Sign up using Email and Password
Post as a guest
Required, but never shown
StackExchange.ready(
function ()
StackExchange.openid.initPostLogin('.new-post-login', 'https%3a%2f%2fstackoverflow.com%2fquestions%2f55069864%2fnested-apply-instead-of-double-for-loops-in-r-for-multiple-linear-regression%23new-answer', 'question_page');
);
Post as a guest
Required, but never shown
Sign up or log in
StackExchange.ready(function ()
StackExchange.helpers.onClickDraftSave('#login-link');
);
Sign up using Google
Sign up using Facebook
Sign up using Email and Password
Post as a guest
Required, but never shown
Sign up or log in
StackExchange.ready(function ()
StackExchange.helpers.onClickDraftSave('#login-link');
);
Sign up using Google
Sign up using Facebook
Sign up using Email and Password
Post as a guest
Required, but never shown
Sign up or log in
StackExchange.ready(function ()
StackExchange.helpers.onClickDraftSave('#login-link');
);
Sign up using Google
Sign up using Facebook
Sign up using Email and Password
Sign up using Google
Sign up using Facebook
Sign up using Email and Password
Post as a guest
Required, but never shown
Required, but never shown
Required, but never shown
Required, but never shown
Required, but never shown
Required, but never shown
Required, but never shown
Required, but never shown
Required, but never shown
ClE gm,IQiC79IDJGT,Bmq,7k,u 3EKKf1xz5Fg7JK2cLq6MHjPos0P0ItNb4CmBE7dVMIDYO,72ga86tf,J