Loss/Accuracy is bad using LSTM Autoencoders The Next CEO of Stack OverflowAda-Delta method doesn't converge when used in Denoising AutoEncoder with MSE loss & ReLU activation?Understanding Keras LSTMsAutoencoder not learning identity functionLSTM autoencoder on sequences - what loss function?Losses for LSTM autoencoderLSTM Autoencoder on timeseriesReally high loss/low accuracy with a LSTM using KerasSeq2seq LSTM fails to produce sensible summariesHow to use Scikit Learn Wrapper around Keras Bi-directional LSTM ModelWhat loss function to use for an Seq2Seq NMT?
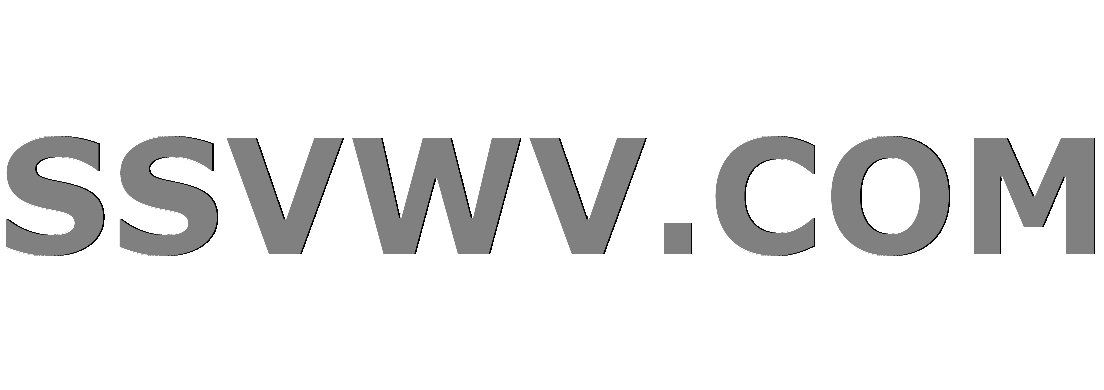
Multi tool use
Is there a difference between "Fahrstuhl" and "Aufzug"
What is meant by "large scale tonal organization?"
Is there a way to save my career from absolute disaster?
Is it ever safe to open a suspicious HTML file (e.g. email attachment)?
Why is the US ranked as #45 in Press Freedom ratings, despite its extremely permissive free speech laws?
Why does standard notation not preserve intervals (visually)
Make solar eclipses exceedingly rare, but still have new moons
Axiom Schema vs Axiom
Is a distribution that is normal, but highly skewed considered Gaussian?
Help understanding this unsettling image of Titan, Epimetheus, and Saturn's rings?
How to write a definition with variants?
"misplaced omit" error when >centering columns
What connection does MS Office have to Netscape Navigator?
Unclear about dynamic binding
Running a General Election and the European Elections together
Does soap repel water?
How to prove a simple equation?
Yu-Gi-Oh cards in Python 3
What does "Its cash flow is deeply negative" mean?
Why doesn't UK go for the same deal Japan has with EU to resolve Brexit?
I believe this to be a fraud - hired, then asked to cash check and send cash as Bitcoin
Find non-case sensitive string in a mixed list of elements?
0 rank tensor vs 1D vector
Does increasing your ability score affect your main stat?
Loss/Accuracy is bad using LSTM Autoencoders
The Next CEO of Stack OverflowAda-Delta method doesn't converge when used in Denoising AutoEncoder with MSE loss & ReLU activation?Understanding Keras LSTMsAutoencoder not learning identity functionLSTM autoencoder on sequences - what loss function?Losses for LSTM autoencoderLSTM Autoencoder on timeseriesReally high loss/low accuracy with a LSTM using KerasSeq2seq LSTM fails to produce sensible summariesHow to use Scikit Learn Wrapper around Keras Bi-directional LSTM ModelWhat loss function to use for an Seq2Seq NMT?
I am trying to create LSTM Autoencoders for time series data. My goal is to use the encoded representation of these sequences which are generated by the autoencoder and classify them. The length of all sequences is really large(around 1M) and I have 9 sequences like this. Hence, I am using fit generators along with the LSTMs to incorporate my data. However, my loss function is not converging and accuracy is really bad for the LSTM Autoencoders. Here is a part of my code.
encoder_input = Input(shape=(None, input_dim))
encoder_output = LSTM(latent_space)(encoder_input)
decoder_input = Lambda(repeat_vector, output_shape=(None, latent_space))([encoder_output, encoder_input])
decoder_out = LSTM(latent_space, return_sequences=True)(decoder_input)
decoder_output = TimeDistributed(Dense(input_dim))(decoder_out)
autoencoder = Model(encoder_input, decoder_output)
encoder = Model(encoder_input, encoder_output)
autoencoder.compile(optimizer="Adam", loss="mse", metrics=["accuracy"])
def generator(X_data, window_size, step_size):
size_data = X_data.shape[0]
# print('size:',size_data)
while 1:
for k in range(0, size_data - window_size, step_size):
x_batch = X_data[k:k + window_size, :]
x_batch = x_batch.reshape(1,x_batch.shape[0], x_batch.shape[1])
# print(x_batch.shape)
y_batch = x_batch
# print("i = " + str(i))
yield x_batch, y_batch
# print('value: ', x_batch, y_batch)
sliding_window_size = 200
step_size_of_sliding_window = 200
n_epochs = 50
for epoch in range(n_epochs):
print("At Iteration: " + str(epoch))
losses = []
csv_logger = CSVLogger('log.csv', append=True, separator=';')
loss = autoencoder.fit_generator(generator(X_tr,sliding_window_size, step_size_of_sliding_window),steps_per_epoch=shots_train,epochs=1,verbose=2,callbacks=[csv_logger])
losses.append(loss.history['loss'])
I am getting the following results in brief:
Epoch 1/1
- 6s - loss: 0.0319 - acc: 0.5425
At Iteration: 6
Epoch 1/1
- 6s - loss: 0.0326 - acc: 0.4555
At Iteration: 7
Epoch 1/1
- 6s - loss: 0.0301 - acc: 0.4865
At Iteration: 8
Epoch 1/1
- 6s - loss: 0.0304 - acc: 0.5020
At Iteration: 9
Epoch 1/1
- 6s - loss: 0.0313 - acc: 0.4780
At Iteration: 10
Epoch 1/1
- 6s - loss: 0.0313 - acc: 0.5090
At Iteration: 11
Epoch 1/1
- 6s - loss: 0.0313 - acc: 0.4675
The accuracy is really bad and the reconstructed sequence is also different. How can I improve the same? I have already tried using different code sizes in the LSTMs.
python-2.7 keras lstm floating-accuracy autoencoder
add a comment |
I am trying to create LSTM Autoencoders for time series data. My goal is to use the encoded representation of these sequences which are generated by the autoencoder and classify them. The length of all sequences is really large(around 1M) and I have 9 sequences like this. Hence, I am using fit generators along with the LSTMs to incorporate my data. However, my loss function is not converging and accuracy is really bad for the LSTM Autoencoders. Here is a part of my code.
encoder_input = Input(shape=(None, input_dim))
encoder_output = LSTM(latent_space)(encoder_input)
decoder_input = Lambda(repeat_vector, output_shape=(None, latent_space))([encoder_output, encoder_input])
decoder_out = LSTM(latent_space, return_sequences=True)(decoder_input)
decoder_output = TimeDistributed(Dense(input_dim))(decoder_out)
autoencoder = Model(encoder_input, decoder_output)
encoder = Model(encoder_input, encoder_output)
autoencoder.compile(optimizer="Adam", loss="mse", metrics=["accuracy"])
def generator(X_data, window_size, step_size):
size_data = X_data.shape[0]
# print('size:',size_data)
while 1:
for k in range(0, size_data - window_size, step_size):
x_batch = X_data[k:k + window_size, :]
x_batch = x_batch.reshape(1,x_batch.shape[0], x_batch.shape[1])
# print(x_batch.shape)
y_batch = x_batch
# print("i = " + str(i))
yield x_batch, y_batch
# print('value: ', x_batch, y_batch)
sliding_window_size = 200
step_size_of_sliding_window = 200
n_epochs = 50
for epoch in range(n_epochs):
print("At Iteration: " + str(epoch))
losses = []
csv_logger = CSVLogger('log.csv', append=True, separator=';')
loss = autoencoder.fit_generator(generator(X_tr,sliding_window_size, step_size_of_sliding_window),steps_per_epoch=shots_train,epochs=1,verbose=2,callbacks=[csv_logger])
losses.append(loss.history['loss'])
I am getting the following results in brief:
Epoch 1/1
- 6s - loss: 0.0319 - acc: 0.5425
At Iteration: 6
Epoch 1/1
- 6s - loss: 0.0326 - acc: 0.4555
At Iteration: 7
Epoch 1/1
- 6s - loss: 0.0301 - acc: 0.4865
At Iteration: 8
Epoch 1/1
- 6s - loss: 0.0304 - acc: 0.5020
At Iteration: 9
Epoch 1/1
- 6s - loss: 0.0313 - acc: 0.4780
At Iteration: 10
Epoch 1/1
- 6s - loss: 0.0313 - acc: 0.5090
At Iteration: 11
Epoch 1/1
- 6s - loss: 0.0313 - acc: 0.4675
The accuracy is really bad and the reconstructed sequence is also different. How can I improve the same? I have already tried using different code sizes in the LSTMs.
python-2.7 keras lstm floating-accuracy autoencoder
add a comment |
I am trying to create LSTM Autoencoders for time series data. My goal is to use the encoded representation of these sequences which are generated by the autoencoder and classify them. The length of all sequences is really large(around 1M) and I have 9 sequences like this. Hence, I am using fit generators along with the LSTMs to incorporate my data. However, my loss function is not converging and accuracy is really bad for the LSTM Autoencoders. Here is a part of my code.
encoder_input = Input(shape=(None, input_dim))
encoder_output = LSTM(latent_space)(encoder_input)
decoder_input = Lambda(repeat_vector, output_shape=(None, latent_space))([encoder_output, encoder_input])
decoder_out = LSTM(latent_space, return_sequences=True)(decoder_input)
decoder_output = TimeDistributed(Dense(input_dim))(decoder_out)
autoencoder = Model(encoder_input, decoder_output)
encoder = Model(encoder_input, encoder_output)
autoencoder.compile(optimizer="Adam", loss="mse", metrics=["accuracy"])
def generator(X_data, window_size, step_size):
size_data = X_data.shape[0]
# print('size:',size_data)
while 1:
for k in range(0, size_data - window_size, step_size):
x_batch = X_data[k:k + window_size, :]
x_batch = x_batch.reshape(1,x_batch.shape[0], x_batch.shape[1])
# print(x_batch.shape)
y_batch = x_batch
# print("i = " + str(i))
yield x_batch, y_batch
# print('value: ', x_batch, y_batch)
sliding_window_size = 200
step_size_of_sliding_window = 200
n_epochs = 50
for epoch in range(n_epochs):
print("At Iteration: " + str(epoch))
losses = []
csv_logger = CSVLogger('log.csv', append=True, separator=';')
loss = autoencoder.fit_generator(generator(X_tr,sliding_window_size, step_size_of_sliding_window),steps_per_epoch=shots_train,epochs=1,verbose=2,callbacks=[csv_logger])
losses.append(loss.history['loss'])
I am getting the following results in brief:
Epoch 1/1
- 6s - loss: 0.0319 - acc: 0.5425
At Iteration: 6
Epoch 1/1
- 6s - loss: 0.0326 - acc: 0.4555
At Iteration: 7
Epoch 1/1
- 6s - loss: 0.0301 - acc: 0.4865
At Iteration: 8
Epoch 1/1
- 6s - loss: 0.0304 - acc: 0.5020
At Iteration: 9
Epoch 1/1
- 6s - loss: 0.0313 - acc: 0.4780
At Iteration: 10
Epoch 1/1
- 6s - loss: 0.0313 - acc: 0.5090
At Iteration: 11
Epoch 1/1
- 6s - loss: 0.0313 - acc: 0.4675
The accuracy is really bad and the reconstructed sequence is also different. How can I improve the same? I have already tried using different code sizes in the LSTMs.
python-2.7 keras lstm floating-accuracy autoencoder
I am trying to create LSTM Autoencoders for time series data. My goal is to use the encoded representation of these sequences which are generated by the autoencoder and classify them. The length of all sequences is really large(around 1M) and I have 9 sequences like this. Hence, I am using fit generators along with the LSTMs to incorporate my data. However, my loss function is not converging and accuracy is really bad for the LSTM Autoencoders. Here is a part of my code.
encoder_input = Input(shape=(None, input_dim))
encoder_output = LSTM(latent_space)(encoder_input)
decoder_input = Lambda(repeat_vector, output_shape=(None, latent_space))([encoder_output, encoder_input])
decoder_out = LSTM(latent_space, return_sequences=True)(decoder_input)
decoder_output = TimeDistributed(Dense(input_dim))(decoder_out)
autoencoder = Model(encoder_input, decoder_output)
encoder = Model(encoder_input, encoder_output)
autoencoder.compile(optimizer="Adam", loss="mse", metrics=["accuracy"])
def generator(X_data, window_size, step_size):
size_data = X_data.shape[0]
# print('size:',size_data)
while 1:
for k in range(0, size_data - window_size, step_size):
x_batch = X_data[k:k + window_size, :]
x_batch = x_batch.reshape(1,x_batch.shape[0], x_batch.shape[1])
# print(x_batch.shape)
y_batch = x_batch
# print("i = " + str(i))
yield x_batch, y_batch
# print('value: ', x_batch, y_batch)
sliding_window_size = 200
step_size_of_sliding_window = 200
n_epochs = 50
for epoch in range(n_epochs):
print("At Iteration: " + str(epoch))
losses = []
csv_logger = CSVLogger('log.csv', append=True, separator=';')
loss = autoencoder.fit_generator(generator(X_tr,sliding_window_size, step_size_of_sliding_window),steps_per_epoch=shots_train,epochs=1,verbose=2,callbacks=[csv_logger])
losses.append(loss.history['loss'])
I am getting the following results in brief:
Epoch 1/1
- 6s - loss: 0.0319 - acc: 0.5425
At Iteration: 6
Epoch 1/1
- 6s - loss: 0.0326 - acc: 0.4555
At Iteration: 7
Epoch 1/1
- 6s - loss: 0.0301 - acc: 0.4865
At Iteration: 8
Epoch 1/1
- 6s - loss: 0.0304 - acc: 0.5020
At Iteration: 9
Epoch 1/1
- 6s - loss: 0.0313 - acc: 0.4780
At Iteration: 10
Epoch 1/1
- 6s - loss: 0.0313 - acc: 0.5090
At Iteration: 11
Epoch 1/1
- 6s - loss: 0.0313 - acc: 0.4675
The accuracy is really bad and the reconstructed sequence is also different. How can I improve the same? I have already tried using different code sizes in the LSTMs.
python-2.7 keras lstm floating-accuracy autoencoder
python-2.7 keras lstm floating-accuracy autoencoder
asked Mar 8 at 16:23


Simran AgarwalSimran Agarwal
65
65
add a comment |
add a comment |
0
active
oldest
votes
Your Answer
StackExchange.ifUsing("editor", function ()
StackExchange.using("externalEditor", function ()
StackExchange.using("snippets", function ()
StackExchange.snippets.init();
);
);
, "code-snippets");
StackExchange.ready(function()
var channelOptions =
tags: "".split(" "),
id: "1"
;
initTagRenderer("".split(" "), "".split(" "), channelOptions);
StackExchange.using("externalEditor", function()
// Have to fire editor after snippets, if snippets enabled
if (StackExchange.settings.snippets.snippetsEnabled)
StackExchange.using("snippets", function()
createEditor();
);
else
createEditor();
);
function createEditor()
StackExchange.prepareEditor(
heartbeatType: 'answer',
autoActivateHeartbeat: false,
convertImagesToLinks: true,
noModals: true,
showLowRepImageUploadWarning: true,
reputationToPostImages: 10,
bindNavPrevention: true,
postfix: "",
imageUploader:
brandingHtml: "Powered by u003ca class="icon-imgur-white" href="https://imgur.com/"u003eu003c/au003e",
contentPolicyHtml: "User contributions licensed under u003ca href="https://creativecommons.org/licenses/by-sa/3.0/"u003ecc by-sa 3.0 with attribution requiredu003c/au003e u003ca href="https://stackoverflow.com/legal/content-policy"u003e(content policy)u003c/au003e",
allowUrls: true
,
onDemand: true,
discardSelector: ".discard-answer"
,immediatelyShowMarkdownHelp:true
);
);
Sign up or log in
StackExchange.ready(function ()
StackExchange.helpers.onClickDraftSave('#login-link');
);
Sign up using Google
Sign up using Facebook
Sign up using Email and Password
Post as a guest
Required, but never shown
StackExchange.ready(
function ()
StackExchange.openid.initPostLogin('.new-post-login', 'https%3a%2f%2fstackoverflow.com%2fquestions%2f55067157%2floss-accuracy-is-bad-using-lstm-autoencoders%23new-answer', 'question_page');
);
Post as a guest
Required, but never shown
0
active
oldest
votes
0
active
oldest
votes
active
oldest
votes
active
oldest
votes
Thanks for contributing an answer to Stack Overflow!
- Please be sure to answer the question. Provide details and share your research!
But avoid …
- Asking for help, clarification, or responding to other answers.
- Making statements based on opinion; back them up with references or personal experience.
To learn more, see our tips on writing great answers.
Sign up or log in
StackExchange.ready(function ()
StackExchange.helpers.onClickDraftSave('#login-link');
);
Sign up using Google
Sign up using Facebook
Sign up using Email and Password
Post as a guest
Required, but never shown
StackExchange.ready(
function ()
StackExchange.openid.initPostLogin('.new-post-login', 'https%3a%2f%2fstackoverflow.com%2fquestions%2f55067157%2floss-accuracy-is-bad-using-lstm-autoencoders%23new-answer', 'question_page');
);
Post as a guest
Required, but never shown
Sign up or log in
StackExchange.ready(function ()
StackExchange.helpers.onClickDraftSave('#login-link');
);
Sign up using Google
Sign up using Facebook
Sign up using Email and Password
Post as a guest
Required, but never shown
Sign up or log in
StackExchange.ready(function ()
StackExchange.helpers.onClickDraftSave('#login-link');
);
Sign up using Google
Sign up using Facebook
Sign up using Email and Password
Post as a guest
Required, but never shown
Sign up or log in
StackExchange.ready(function ()
StackExchange.helpers.onClickDraftSave('#login-link');
);
Sign up using Google
Sign up using Facebook
Sign up using Email and Password
Sign up using Google
Sign up using Facebook
Sign up using Email and Password
Post as a guest
Required, but never shown
Required, but never shown
Required, but never shown
Required, but never shown
Required, but never shown
Required, but never shown
Required, but never shown
Required, but never shown
Required, but never shown
yn2 VpyWL8TF8PhfL8,heL,K9O5vpv0tRfI9